Receive a weekly summary and discussion of the top papers of the week by leading researchers in the field.
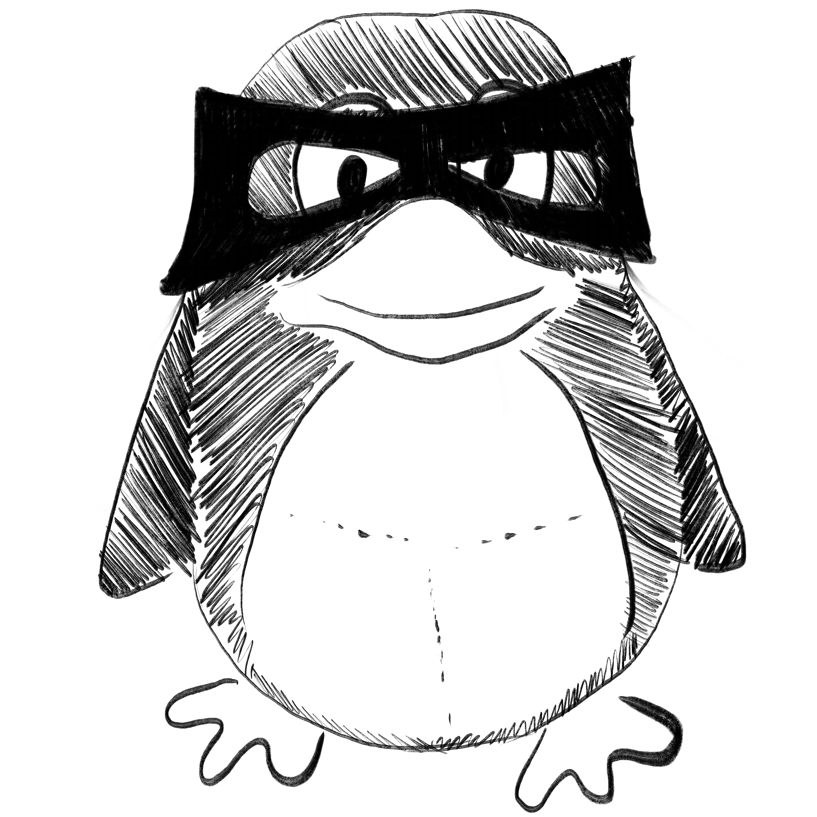
Discovery of novel acetylcholinesterase inhibitors through integration of machine learning with genetic algorithm based in silico screening approaches.
In Frontiers in neuroscience ; h5-index 72.0
INTRODUCTION :
MATERIALS AND METHODS :
RESULT AND DISCUSSION :
Khan Mohd Imran, Taehwan Park, Cho Yunseong, Scotti Marcus, Priscila Barros de Menezes Renata, Husain Fohad Mabood, Alomar Suliman Yousef, Baig Mohammad Hassan, Dong Jae-June
2022
Alzheimer’s disease, acetylcholinesterase (AChE), machine learning (ML), molecular dynamics (MD), virtual screening
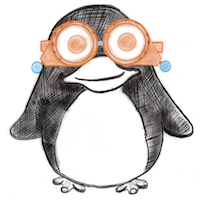
Clinical Applications of Artificial Intelligence in Glaucoma.
In Journal of ophthalmic & vision research
Yousefi Siamak
2023
** Convolutional Neural Network (CNN), Deep Learning, Glaucoma, Machine Learning, Ophthalmology, Artificial Intelligence**
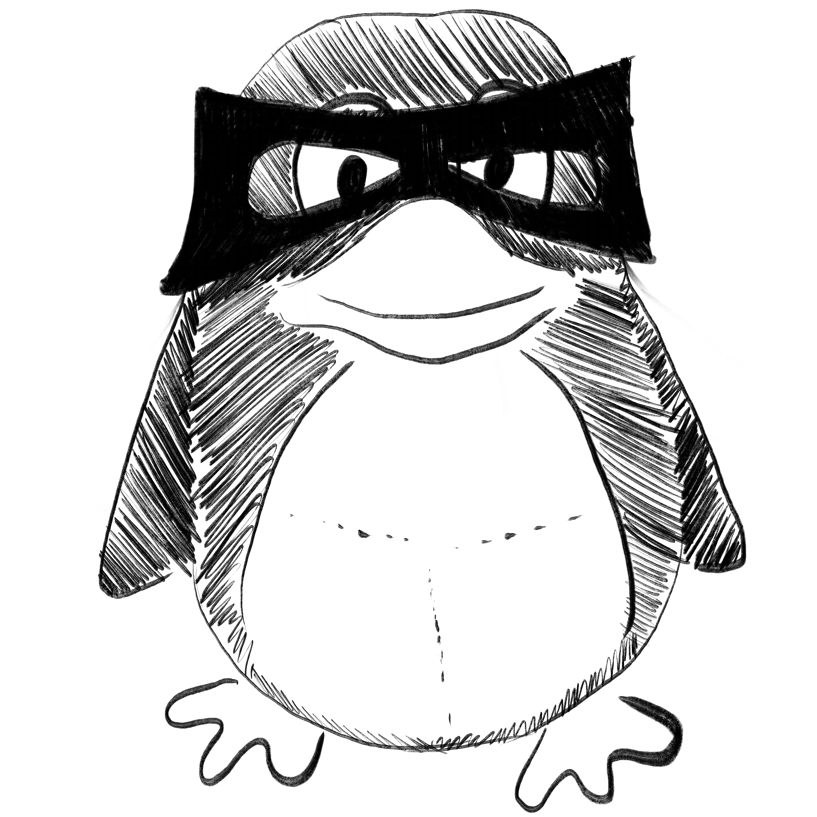
Daily two-photon neuronal population imaging with targeted single-cell electrophysiology and subcellular imaging in auditory cortex of behaving mice.
In Frontiers in cellular neuroscience ; h5-index 74.0
Huang Junjie, Liang Susu, Li Longhui, Li Xingyi, Liao Xiang, Hu Qianshuo, Zhang Chunqing, Jia Hongbo, Chen Xiaowei, Wang Meng, Li Ruijie
2023
auditory cortex, behaving mouse, daily two-photon Ca2+ imaging, dendritic spines, loose-patch recording
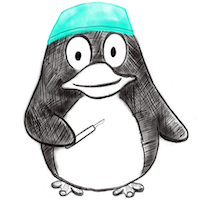
Prediction of Acute Kidney Injury After Cardiac Surgery Using Interpretable Machine Learning.
In Anesthesiology and pain medicine ; h5-index 21.0
BACKGROUND :
OBJECTIVES :
METHODS :
RESULTS :
CONCLUSIONS :
Ejmalian Azar, Aghaei Atefe, Nabavi Shahabedin, Abedzadeh Darabad Maryam, Tajbakhsh Ardeshir, Abin Ahmad Ali, Ebrahimi Moghaddam Mohsen, Dabbagh Ali, Jahangirifard Alireza, Memary Elham, Sayyadi Shahram
2022-Aug
AKI Prediction, Acute Kidney Injury, Cardiac Surgery, Interpretable Machine Learning
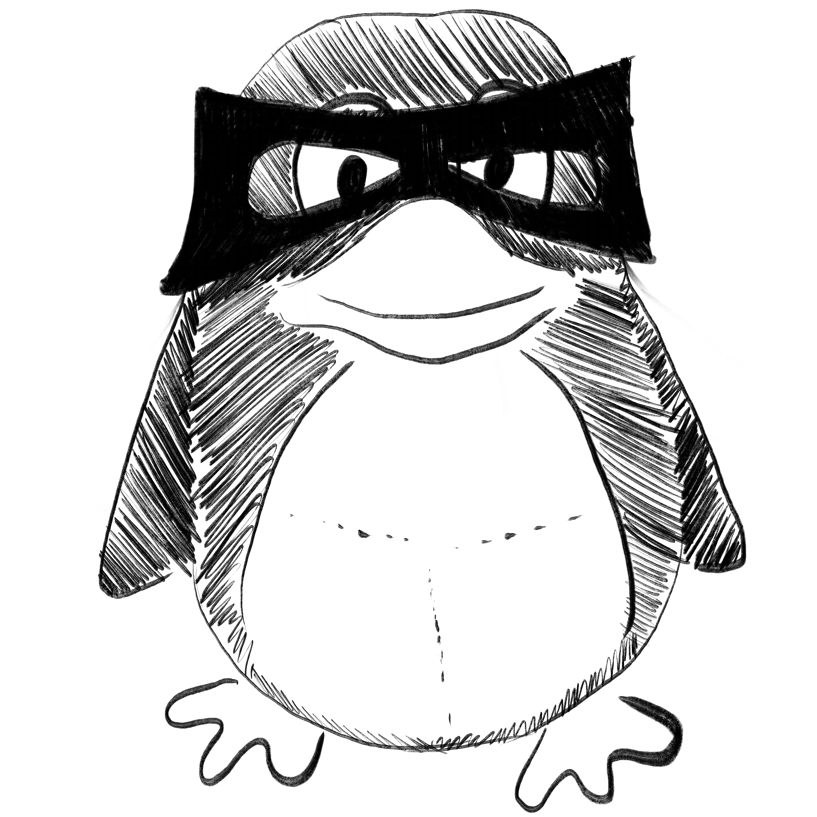
How to prevent malicious use of intelligent unmanned swarms?
In Innovation (Cambridge (Mass.))
Wang Qi, Li Tingting, Xu Yongjun, Wang Fei, Diao Boyu, Zheng Lei, Huang Jincai
2023-Mar-13
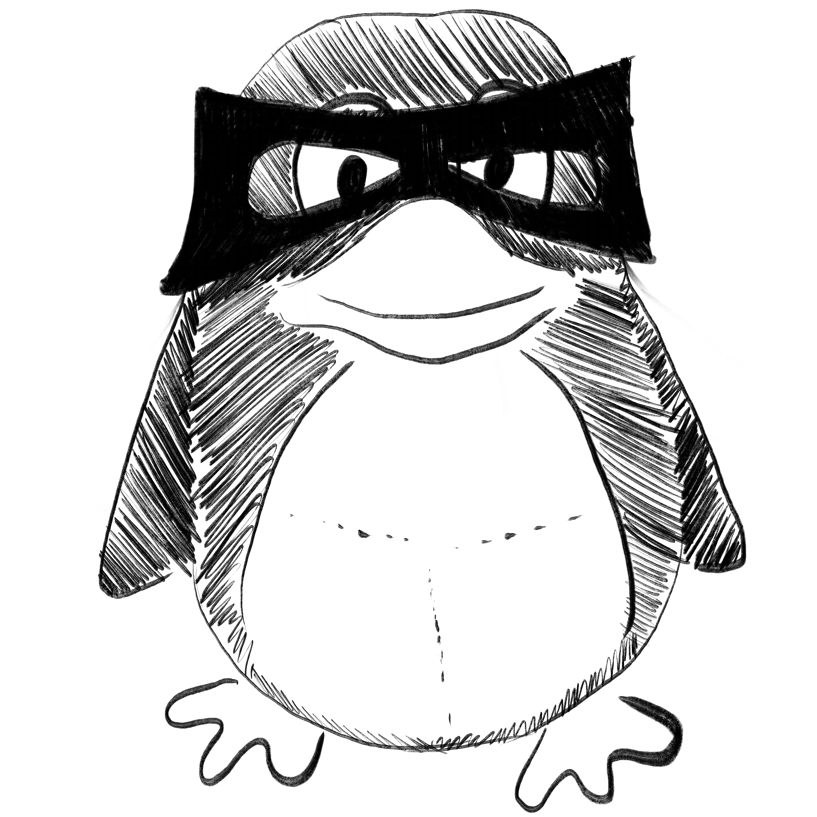
YOUPI: Your powerful and intelligent tool for segmenting cells from imaging mass cytometry data.
In Frontiers in immunology ; h5-index 100.0
Scuiller Yvonne, Hemon Patrice, Le Rochais Marion, Pers Jacques-Olivier, Jamin Christophe, Foulquier Nathan
2023
U-NET, cell segmentation, images, imaging mass cytometry, new algorithm
Weekly Summary
Receive a weekly summary and discussion of the top papers of the week by leading researchers in the field.