Receive a weekly summary and discussion of the top papers of the week by leading researchers in the field.
Artificial intelligence applications in pediatric oncology diagnosis.
In Exploration of targeted anti-tumor therapy
Yang Yuhan, Zhang Yimao, Li Yuan
2023
Pediatric oncology, artificial intelligence, cancer diagnosis, deep learning, machine learning
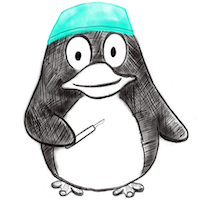
Artificial intelligence fusion for predicting survival of rectal cancer patients using immunohistochemical expression of Ras homolog family member B in biopsy.
In Exploration of targeted anti-tumor therapy
AIM :
METHODS :
RESULTS :
CONCLUSIONS :
Pham Tuan D, Ravi Vinayakumar, Luo Bin, Fan Chuanwen, Sun Xiao-Feng
2023
Artificial intelligence, biomarkers, immunohistochemistry, machine learning, precision medicine, proteins, rectal neoplasms
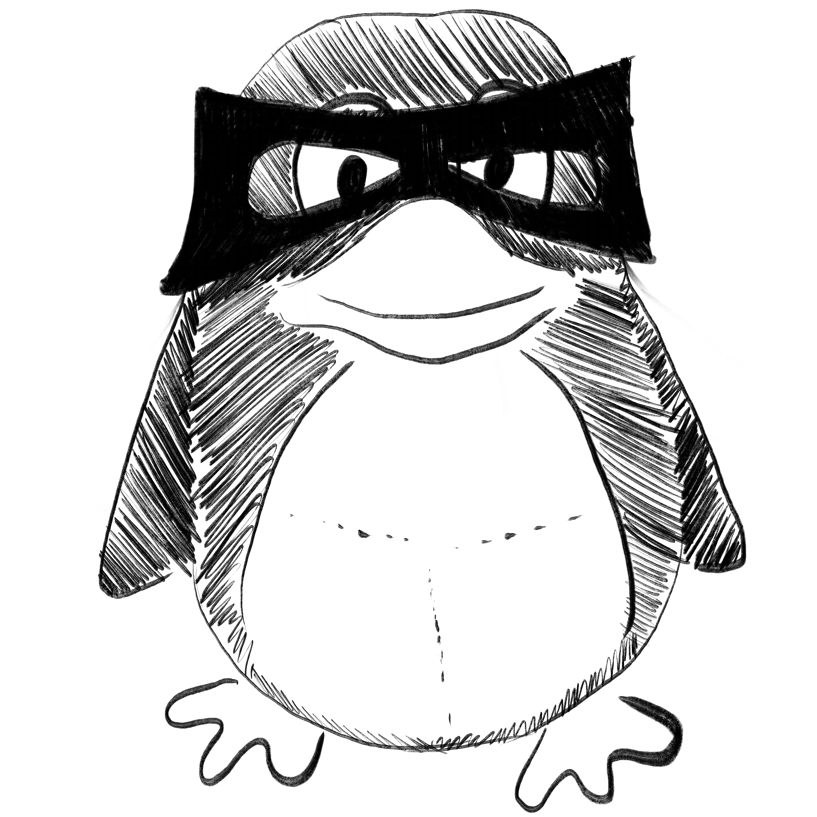
A chatbot-based intervention with ELME to improve stress and health-related parameters in a stressed sample: Study protocol of a randomised controlled trial.
In Frontiers in digital health
BACKGROUND :
METHODS :
DISCUSSION :
TRIAL REGISTRATION :
Schillings C, Meissner D, Erb B, Schultchen D, Bendig E, Pollatos O
2023
chatbot, digital health, interoception, intervention, mindfulness, stress
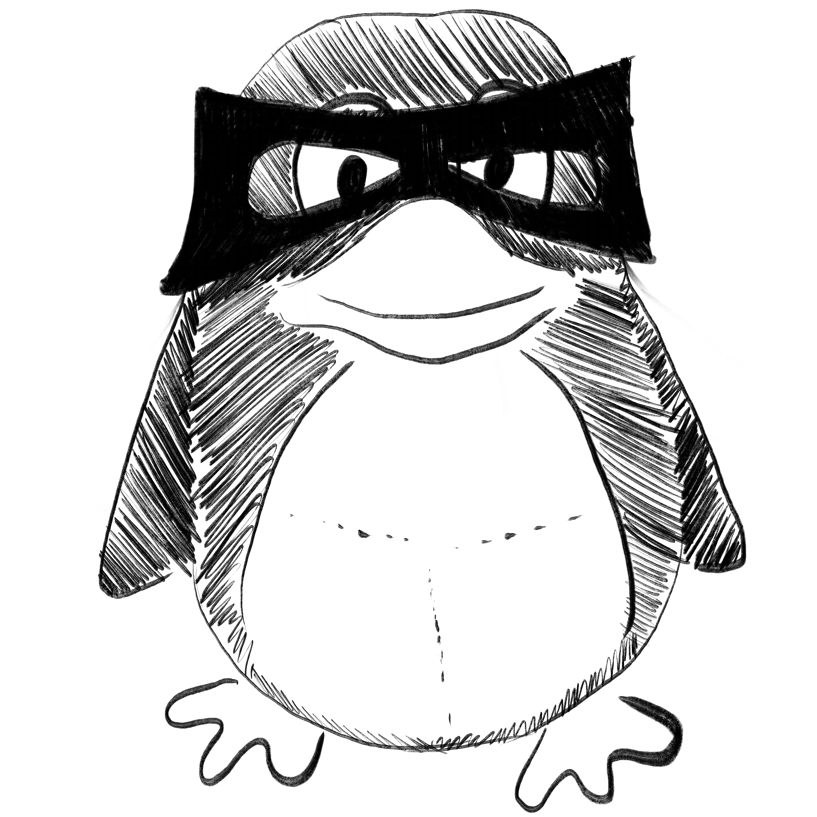
CRMSNet: a deep learning model that uses convolution and residual multi-head self-attention block to predict RBPs for RNA sequence.
In Proteins
Pan Zhengsen, Zhou Shusen, Zou Hailin, Liu Chanjuan, Zang Mujun, Liu Tong, Wang Qingjun
2023-Mar-19
RNA-protein binding sites, convolutional neural networks, deep learning, multi-head self-attention, prediction, residual neural networks
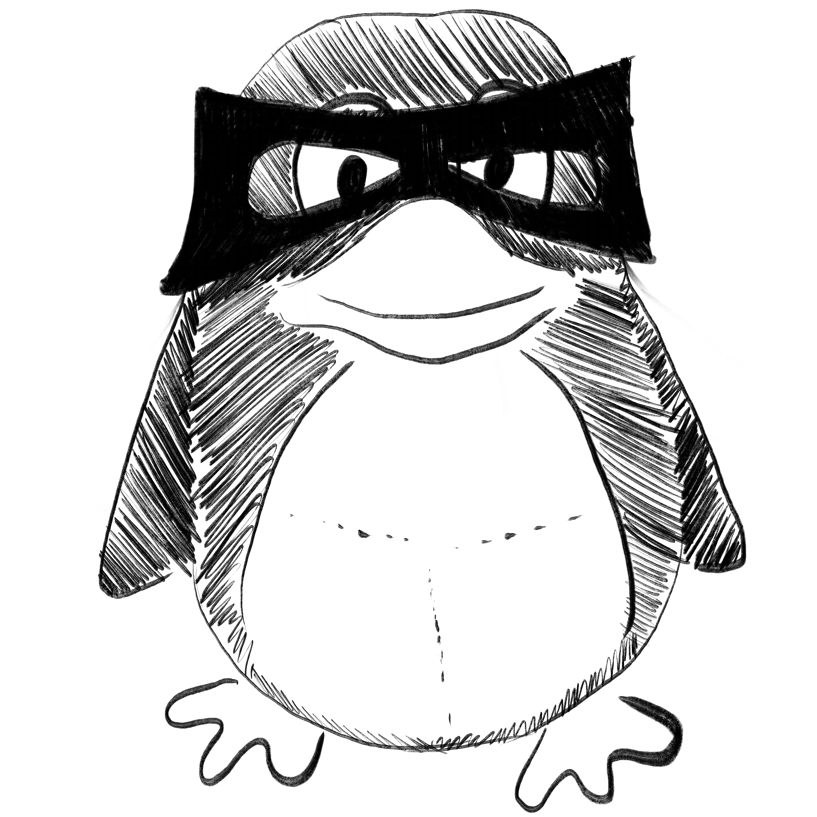
Applying Blockchain Technology in Network Public Opinion Risk Management System in Big Data Environment.
In Computational intelligence and neuroscience
Luo Zhenqing, Zhang Cheng
2023
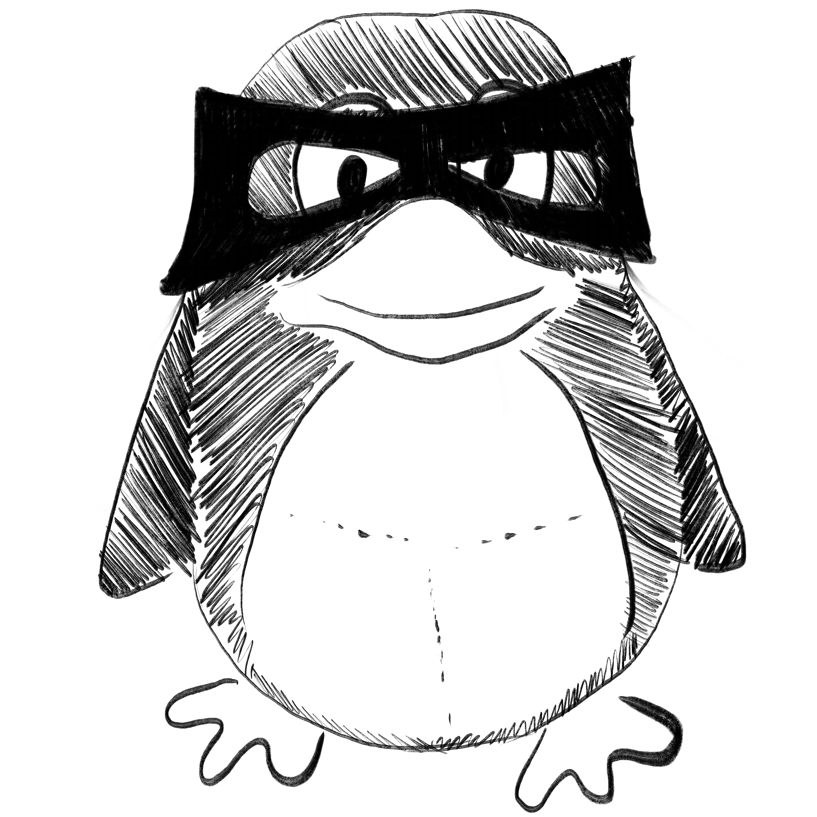
Semantic Latent Space Regression of Diffusion Autoencoders for Vertebral Fracture Grading
ArXiv Preprint
Matthias Keicher, Matan Atad, David Schinz, Alexandra S. Gersing, Sarah C. Foreman, Sophia S. Goller, Juergen Weissinger, Jon Rischewski, Anna-Sophia Dietrich, Benedikt Wiestler, Jan S. Kirschke, Nassir Navab
2023-03-21
Weekly Summary
Receive a weekly summary and discussion of the top papers of the week by leading researchers in the field.