Receive a weekly summary and discussion of the top papers of the week by leading researchers in the field.
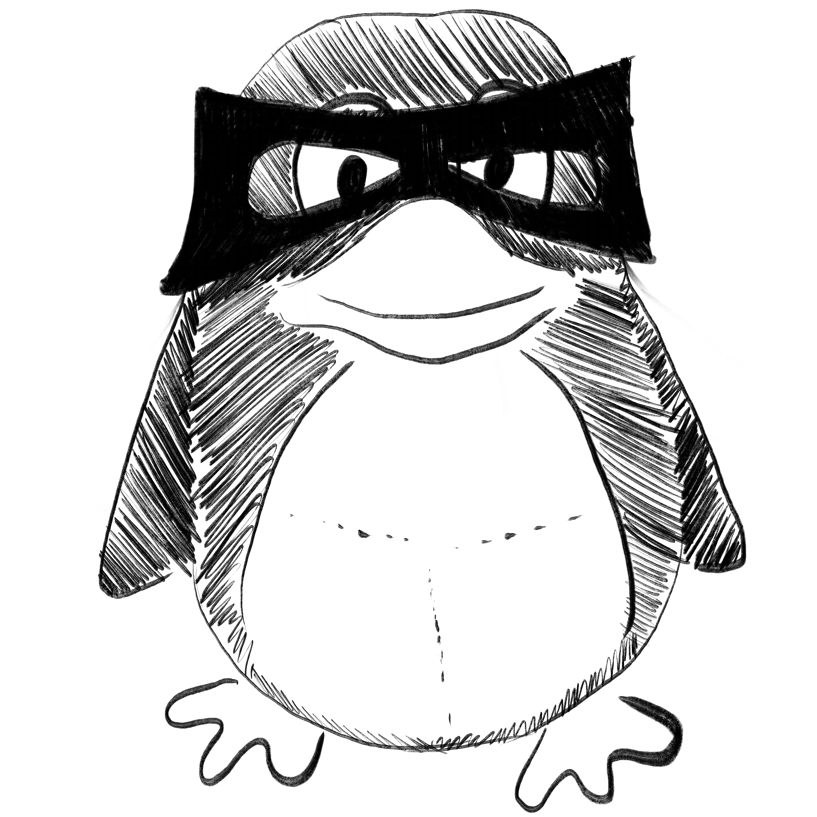
A comparison of machine learning models and Cox proportional hazards models regarding their ability to predict the risk of gastrointestinal cancer based on metabolic syndrome and its components.
In Frontiers in oncology
BACKGROUND :
METHODS :
RESULTS :
CONCLUSIONS :
Tran Tao Thi, Lee Jeonghee, Gunathilake Madhawa, Kim Junetae, Kim Sun-Young, Cho Hyunsoon, Kim Jeongseon
2023
Korea, gastrointestinal cancer, machine learning, metabolic syndrome, prospective cohort study
A CT based radiomics nomogram for differentiation between focal-type autoimmune pancreatitis and pancreatic ductal adenocarcinoma.
In Frontiers in oncology
OBJECTIVES :
METHODS :
RESULTS :
CONCLUSIONS :
Lu Jia, Jiang Nannan, Zhang Yuqing, Li Daowei
2023
differential, focal-type autoimmune pancreatitis, machine learning, pancreatic ductal adenocarcinoma, radiomics
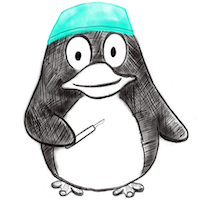
Identification of the mitophagy-related diagnostic biomarkers in hepatocellular carcinoma based on machine learning algorithm and construction of prognostic model.
In Frontiers in oncology
BACKGROUND AND AIMS :
METHODS :
RESULTS :
CONCLUSION :
Tu Dao-Yuan, Cao Jun, Zhou Jie, Su Bing-Bing, Wang Shun-Yi, Jiang Guo-Qing, Jin Sheng-Jie, Zhang Chi, Peng Rui, Bai Dou-Sheng
2023
bioinformatics, hepatocelluar carcinoma, machine learning, mitophagy, prognostic model, time
Self-supervised contrastive learning using CT images for PD-1/PD-L1 expression prediction in hepatocellular carcinoma.
In Frontiers in oncology
BACKGROUND AND PURPOSE :
MATERIALS AND METHODS :
RESULTS :
CONCLUSIONS :
Xie Tianshu, Wei Yi, Xu Lifeng, Li Qian, Che Feng, Xu Qing, Cheng Xuan, Liu Minghui, Yang Meiyi, Wang Xiaomin, Zhang Feng, Song Bin, Liu Ming
2023
PD-1/L1, computed tomography, contrastive learning, deep learning, hepatocellular carcinoma, self-supervised learning
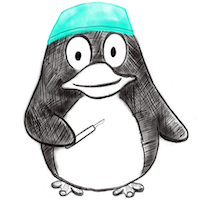
Investigation of trends in gut microbiome associated with colorectal cancer using machine learning.
In Frontiers in oncology
BACKGROUND :
METHODS :
RESULTS :
CONCLUSION :
Yu Chaoran, Zhou Zhiyuan, Liu Bin, Yao Danhua, Huang Yuhua, Wang Pengfei, Li Yousheng
2023
Latent Dirichlet Allocation, Web of Science, bibliometric, colorectal cancer, microbiome
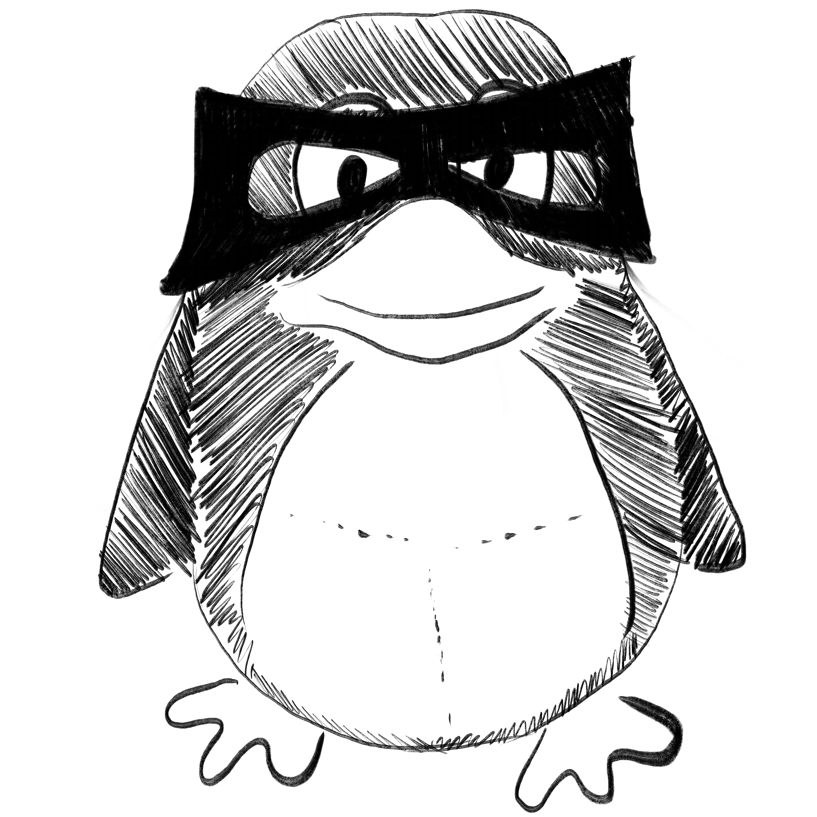
Configural relations in humans and deep convolutional neural networks.
In Frontiers in artificial intelligence
Baker Nicholas, Garrigan Patrick, Phillips Austin, Kellman Philip J
2022
DCNNs, abstract relations, abstract representation, deep convolutional neural networks, deep learning, perception of relations, shape perception, visual relations
Weekly Summary
Receive a weekly summary and discussion of the top papers of the week by leading researchers in the field.