Receive a weekly summary and discussion of the top papers of the week by leading researchers in the field.
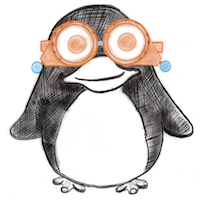
A quantitative study of the effect of ICL orientation selection on post-operative vault and model-assisted vault prediction.
In Frontiers in neurology
BACKGROUND :
METHODS :
RESULTS :
CONCLUSION :
Zhang Weijie, Li Fang, Li Lin, Zhang Jing
2023
ICL implantation orientation, machine-learning, myopia, optic nerve, vault height
Feasibility of a deep-learning based anatomical region labeling tool for Cone-Beam Computed Tomography scans in radiotherapy.
In Physics and imaging in radiation oncology
BACKGROUND AND PURPOSE :
MATERIALS AND METHODS :
RESULTS :
CONCLUSION :
Luximon Dishane C, Neylon John, Lamb James M
2023-Jan
Anatomy labeling, Cone-beam computed tomography, Deep learning, Radiotherapy
Mechanisms influencing the factors of urban built environments and coronavirus disease 2019 at macroscopic and microscopic scales: The role of cities.
In Frontiers in public health
Zhang Longhao, Han Xin, Wu Jun, Wang Lei
2023
COVID-19, computer vision, deep learning, relevance, street view images, urban built environment
Computed tomography-based COVID-19 triage through a deep neural network using mask-weighted global average pooling.
In Frontiers in cellular and infection microbiology ; h5-index 53.0
BACKGROUND :
METHODS :
RESULTS :
CONCLUSIONS :
Zhang Hong-Tao, Sun Ze-Yu, Zhou Juan, Gao Shen, Dong Jing-Hui, Liu Yuan, Bai Xu, Ma Jin-Lin, Li Ming, Li Guang, Cai Jian-Ming, Sheng Fu-Geng
2023
artificial intelligence, computed tomography (CT), coronavirus disease 2019 (COVID-19), deep learning, global average pooling (GAP)
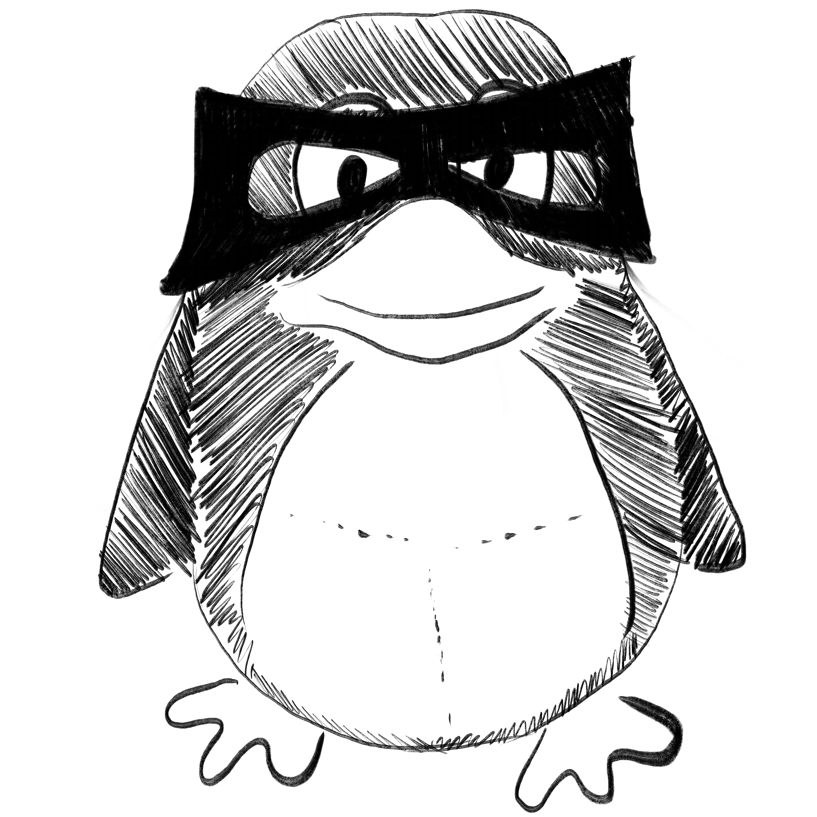
Dynamic Healthcare Embeddings for Improving Patient Care
ArXiv Preprint
Hankyu Jang, Sulyun Lee, D. M. Hasibul Hasan, Philip M. Polgreen, Sriram V. Pemmaraju, Bijaya Adhikari
2023-03-21
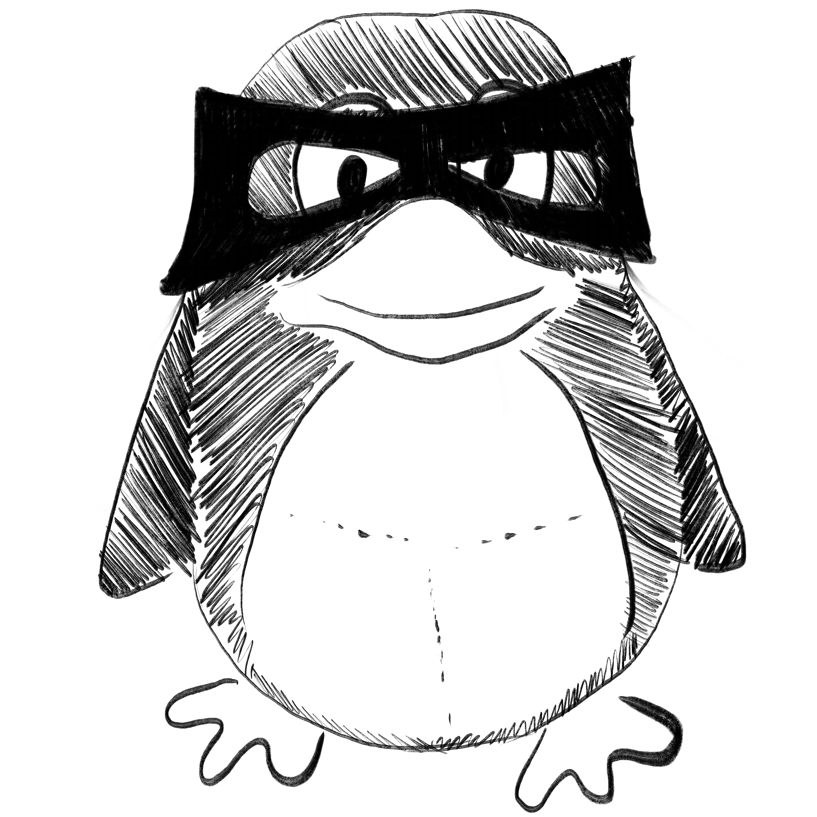
Diagnosis and prognosis prediction model for digestive system tumors based on immunologic gene sets.
In Frontiers in oncology
Zhou Lin, Wang Chunyu
2023
XGBoost, diagnostic, digestive system tumors, immunologic gene set, prognostic
Weekly Summary
Receive a weekly summary and discussion of the top papers of the week by leading researchers in the field.