Receive a weekly summary and discussion of the top papers of the week by leading researchers in the field.
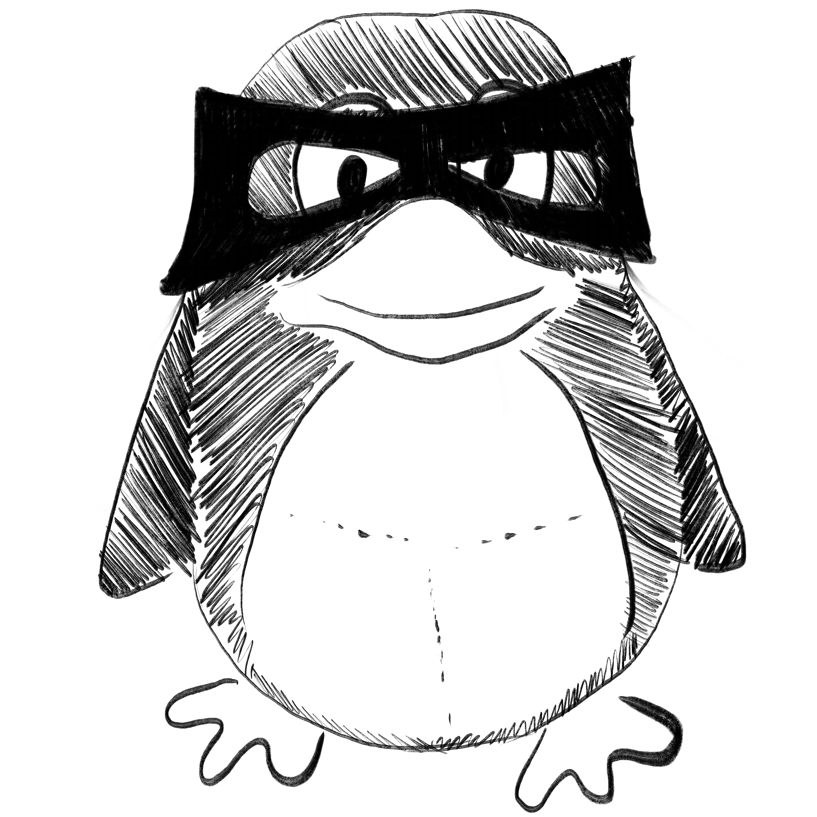
Applying frontier approach to measure the financial efficiency of hospitals.
In Digital health
OBJECTIVES :
METHODS :
RESULTS :
CONCLUSIONS :
Wu Jih-Shong
2023
Financial efficiency, data envelopment analysis, health care, hospital, stochastic frontier analysis
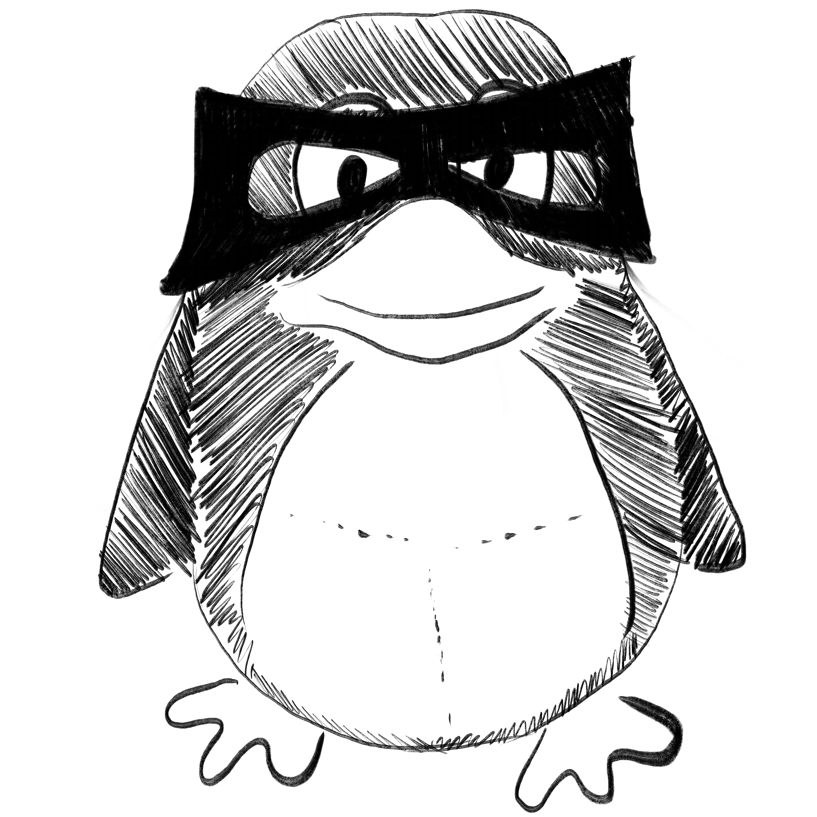
Trainable quantization for Speedy Spiking Neural Networks.
In Frontiers in neuroscience ; h5-index 72.0
Castagnetti Andrea, Pegatoquet Alain, Miramond Benoît
2023
Spiking Neural Networks, direct training, low latency, quantization error, sparsity
Abnormal structural and functional network topological properties associated with left prefrontal, parietal, and occipital cortices significantly predict childhood TBI-related attention deficits: A semi-supervised deep learning study.
In Frontiers in neuroscience ; h5-index 72.0
INTRODUCTION :
METHODS :
RESULTS :
DISCUSSION :
Cao Meng, Wu Kai, Halperin Jeffery M, Li Xiaobo
2023
attention deficits, autoencoder, diffusion tensor imaging, functional magnetic resonance imaging, graph theory, pediatric, semi-supervised deep learning technique, traumatic brain injury
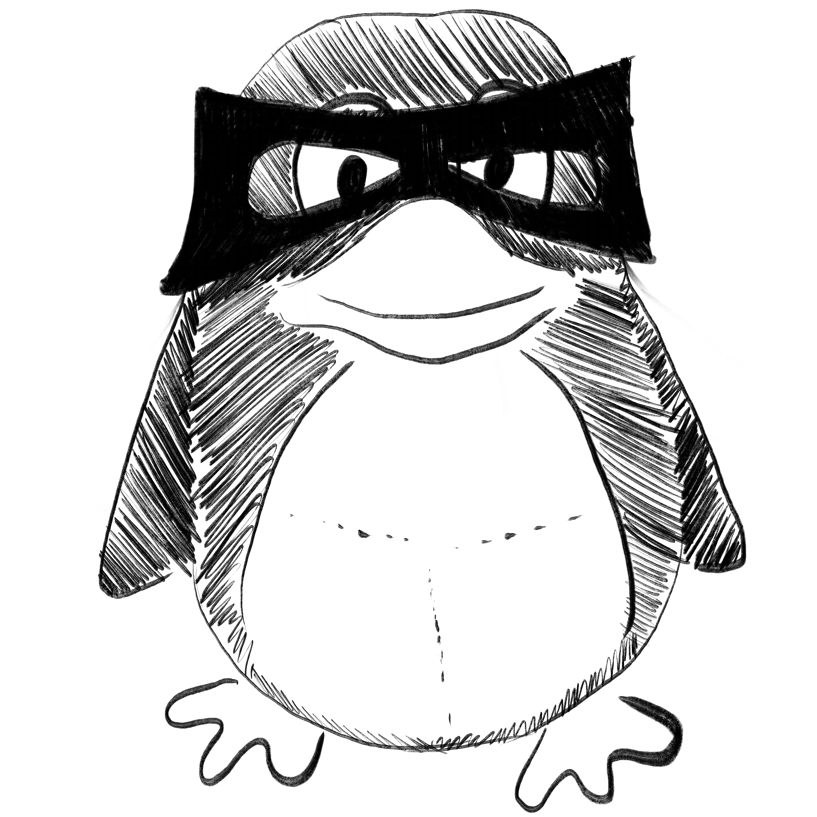
An artificial intelligence lightweight blockchain security model for security and privacy in IIoT systems.
In Journal of cloud computing (Heidelberg, Germany)
Selvarajan Shitharth, Srivastava Gautam, Khadidos Alaa O, Khadidos Adil O, Baza Mohamed, Alshehri Ali, Lin Jerry Chun-Wei
2023
Artificial intelligence, Blockchain, Cloud computing, Convivial Optimized Sprinter Neural Network, Fog computing, Security
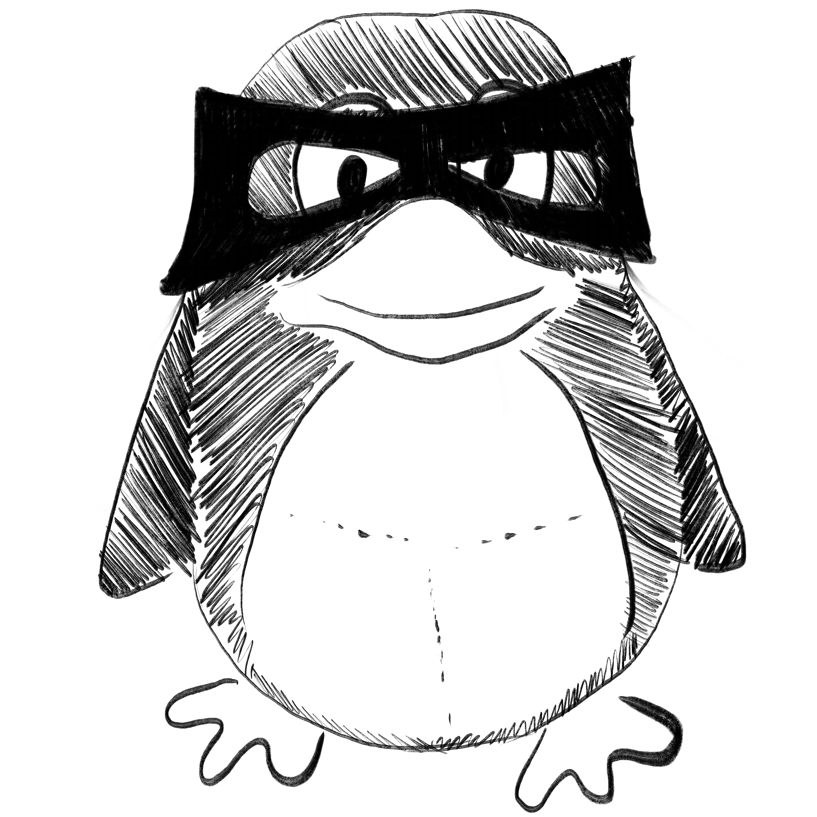
Brain inspired path planning algorithms for drones.
In Frontiers in neurorobotics
INTRODUCTION :
METHODS :
RESULTS :
DISCUSSION :
Chao Yixun, Augenstein Philipp, Roennau Arne, Dillmann Ruediger, Xiong Zhi
2023
Airsim, navigation, path planning, place cells, spiking neural network
Discovering cryptic splice mutations in cancers via a deep neural network framework.
In NAR cancer
Teboul Raphaël, Grabias Michalina, Zucman-Rossi Jessica, Letouzé Eric
2023-Jun
Weekly Summary
Receive a weekly summary and discussion of the top papers of the week by leading researchers in the field.