Receive a weekly summary and discussion of the top papers of the week by leading researchers in the field.
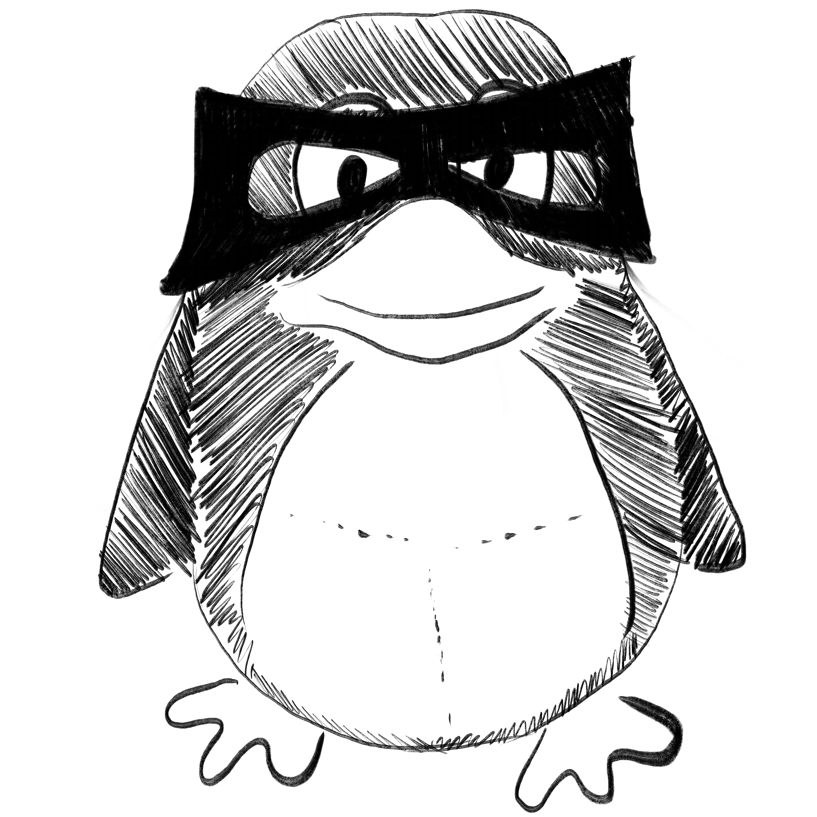
Bone age assessment based on deep neural networks with annotation-free cascaded critical bone region extraction.
In Frontiers in artificial intelligence
Li Zhangyong, Chen Wang, Ju Yang, Chen Yong, Hou Zhengjun, Li Xinwei, Jiang Yuhao
2023
bone age assessment, critical bone region extraction network, gender-assisted bone age estimation network, two-stage deep learning method, visual heat map
An AI-enabled research support tool for the classification system of COVID-19.
In Frontiers in public health
Tiwari Arti, Bhattacharjee Kamanasish, Pant Millie, Srivastava Shilpa, Snasel Vaclav
2023
Artificial Intelligence, COVID-19, bi-directional LSTM, classification, long short-term memory
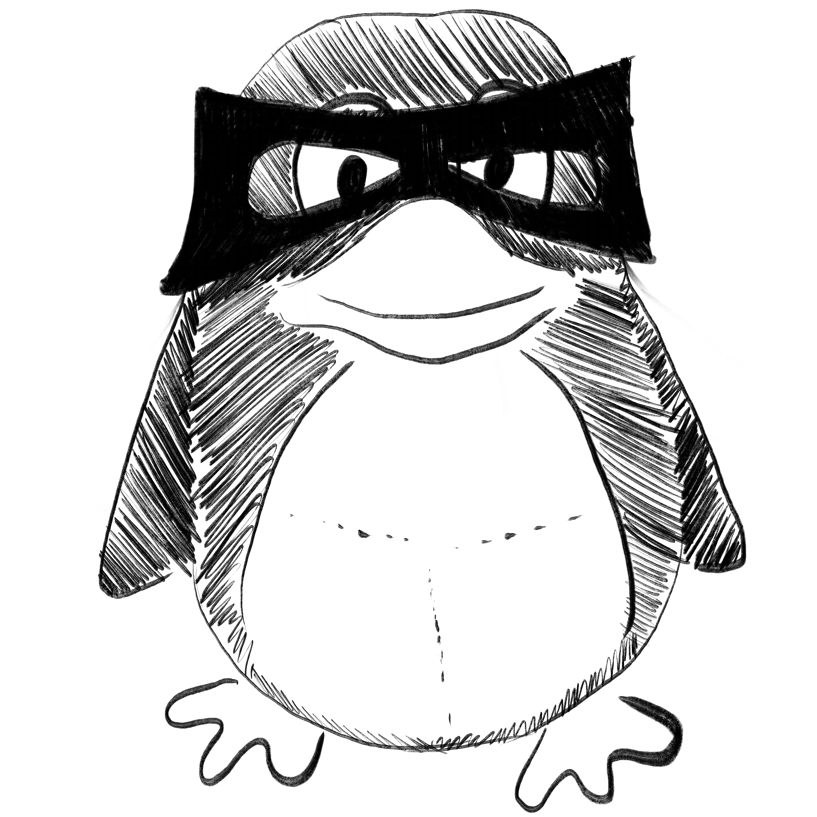
Precision recruitment for high-risk participants in a COVID-19 cohort study.
In Contemporary clinical trials communications
BACKGROUND :
METHODS :
RESULTS :
CONCLUSION :
Mezlini Aziz M, Caddigan Eamon, Shapiro Allison, Ramirez Ernesto, Kondow-McConaghy Helena M, Yang Justin, DeMarco Kerry, Naraghi-Arani Pejman, Foschini Luca
2023-Jun
CDC, Centers for Disease Control and Prevention, COVID-19, Clinical trials, GAMs, generalized additive models, Risk modeling
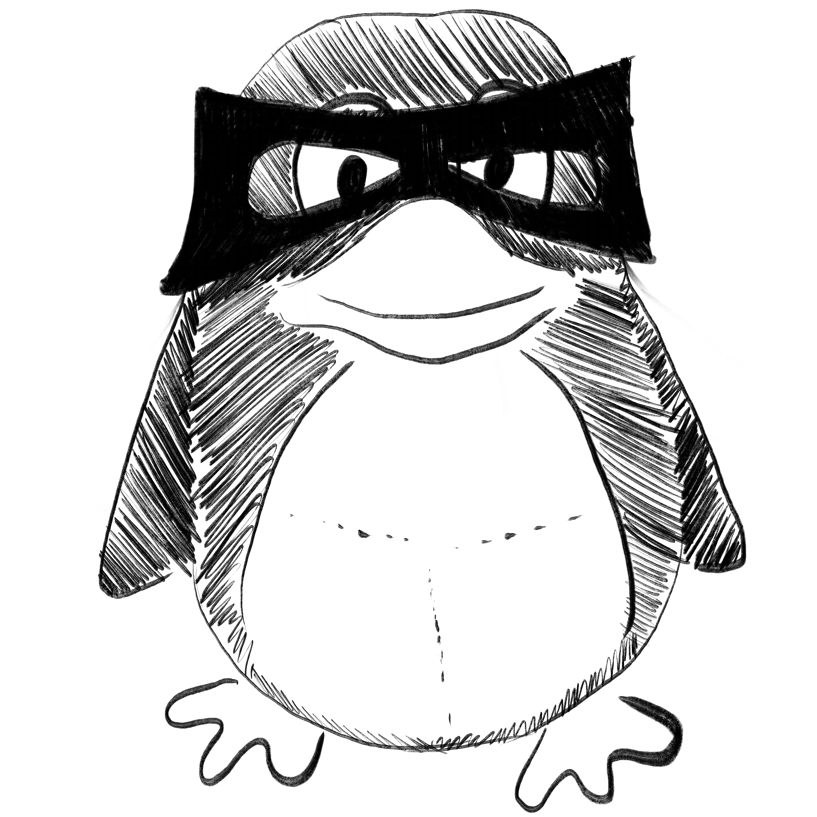
Non-Asymptotic Pointwise and Worst-Case Bounds for Classical Spectrum Estimators
ArXiv Preprint
Andrew Lamperski
2023-03-21
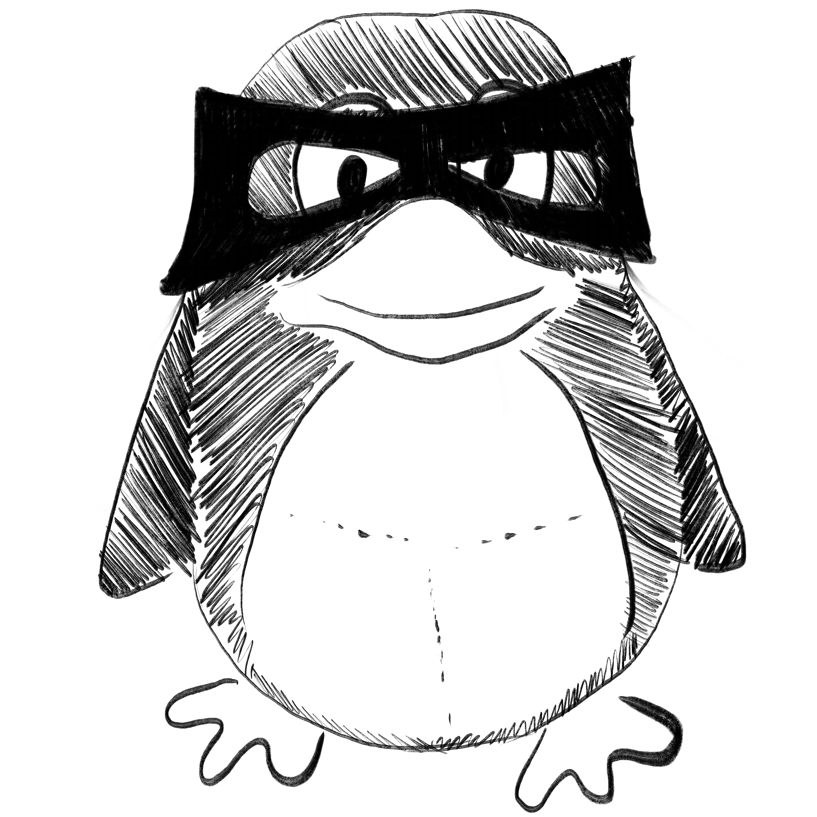
Disembodied AI and the limits to machine understanding of students' embodied interactions.
In Frontiers in artificial intelligence
Nathan Mitchell J
2023
artificial intelligence, augmented intelligence, cognitive science, embodied learning, foundation models, learning sciences, multimodality
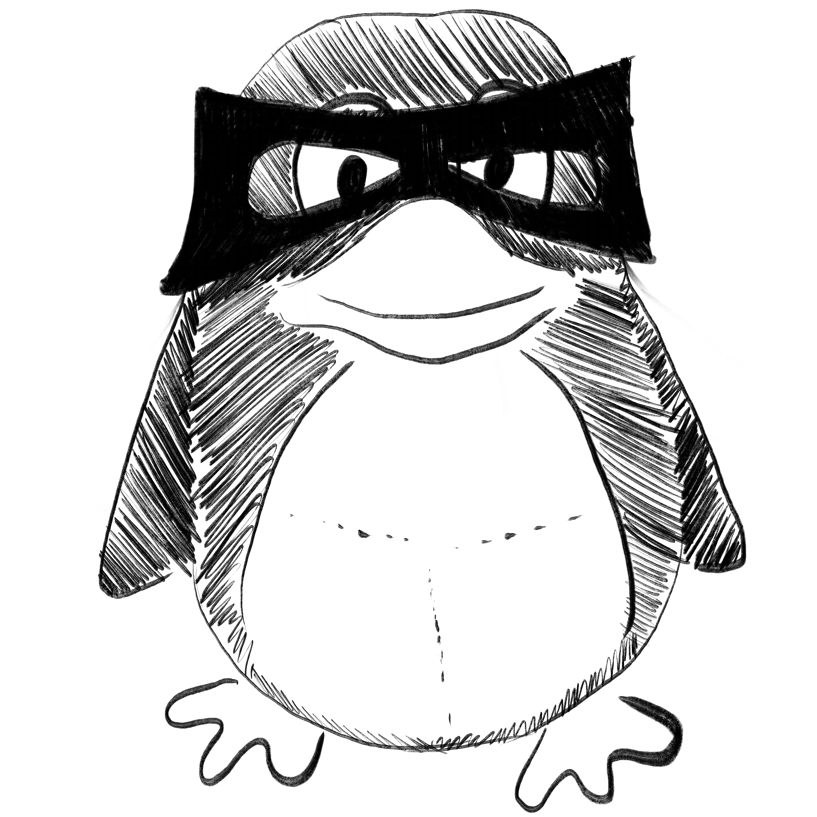
Validation of the influence of biosignals on performance of machine learning algorithms for sleep stage classification.
In Digital health
BACKGROUND :
METHODS :
RESULTS :
CONCLUSION :
Choi Junggu, Kwon Seohyun, Park Sohyun, Han Sanghoon
2023
Sleep stage classification, biosignal, classification algorithm, machine learning, polysomnography
Weekly Summary
Receive a weekly summary and discussion of the top papers of the week by leading researchers in the field.