Receive a weekly summary and discussion of the top papers of the week by leading researchers in the field.
Building a comprehensive cardiovascular magnetic resonance exam on a commercial 0.55 T system: A pictorial essay on potential applications.
In Frontiers in cardiovascular medicine
BACKGROUND :
METHODS :
RESULTS :
CONCLUSION :
Varghese Juliet, Jin Ning, Giese Daniel, Chen Chong, Liu Yingmin, Pan Yue, Nair Nikita, Shalaan Mahmoud T, Khan Mahmood, Tong Matthew S, Ahmad Rizwan, Han Yuchi, Simonetti Orlando P
2023
0.55 T, CMR, LGE, MRA, cine, flow, low-field
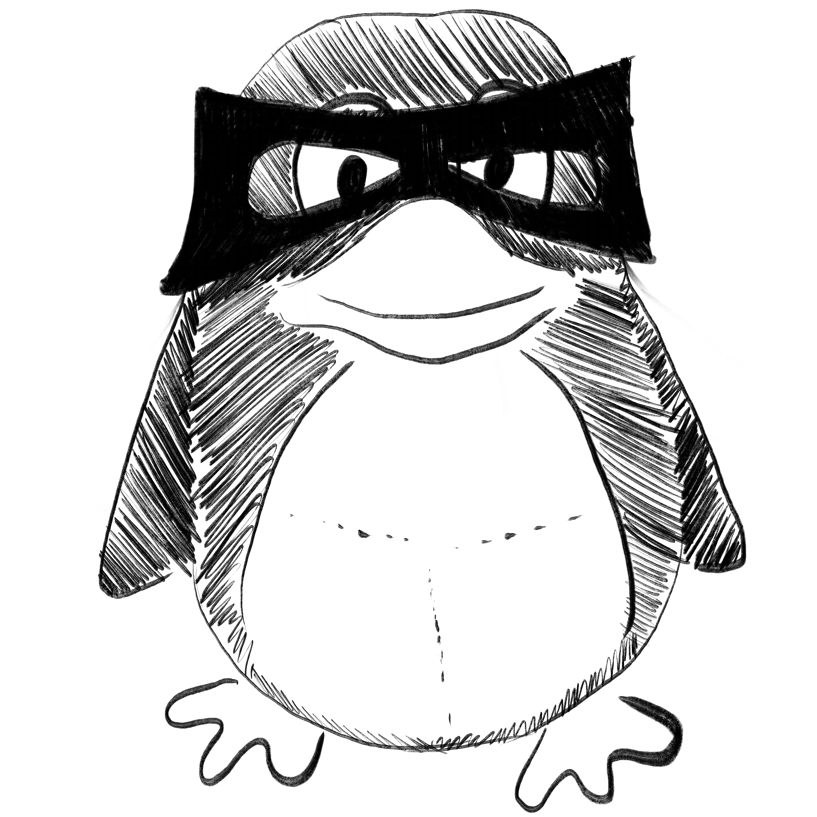
Modelling blood flow in patients with heart valve disease using deep learning: A computationally efficient method to expand diagnostic capabilities in clinical routine.
In Frontiers in cardiovascular medicine
INTRODUCTION :
METHODS :
RESULTS :
Yevtushenko Pavlo, Goubergrits Leonid, Franke Benedikt, Kuehne Titus, Schafstedde Marie
2023
aortic stenosis, artificial neural network, computational fluid dynamics, deep learning, heart valve disease, image-based modelling, in-silico modelling
The application of machine learning in early diagnosis of osteoarthritis: a narrative review.
In Therapeutic advances in musculoskeletal disease
Xuan Anran, Chen Haowei, Chen Tianyu, Li Jia, Lu Shilong, Fan Tianxiang, Zeng Dong, Wen Zhibo, Ma Jianhua, Hunter David, Ding Changhai, Zhu Zhaohua
2023
early diagnosis, machine learning, osteoarthritis, prediction model
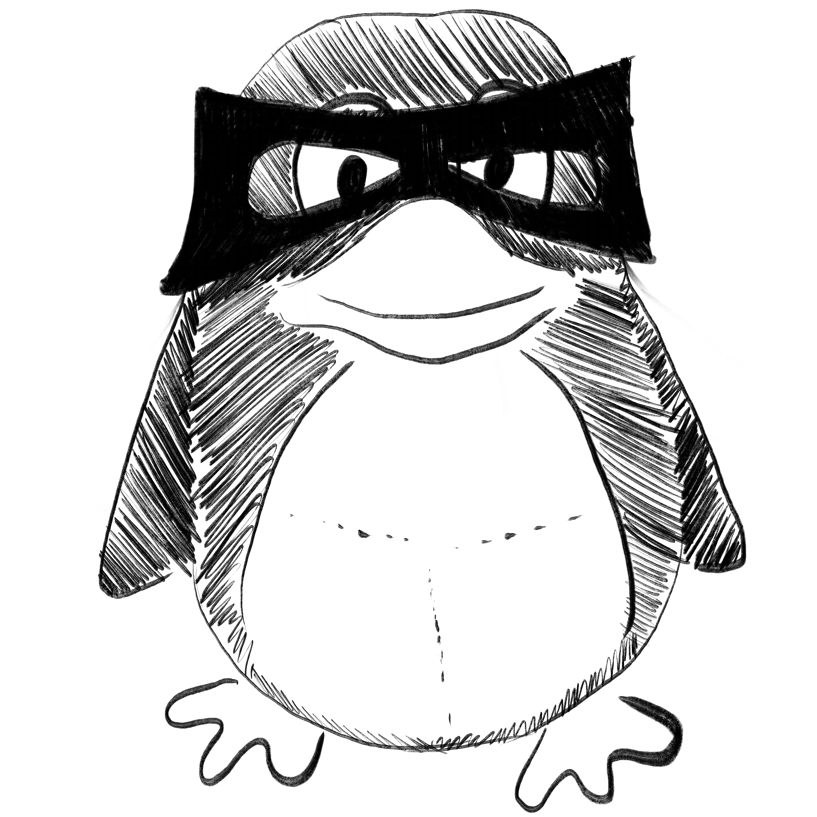
Acquisition of bipedal locomotion in a neuromusculoskeletal model with unilateral transtibial amputation.
In Frontiers in bioengineering and biotechnology
Ichimura Daisuke, Hobara Hiroaki, Hisano Genki, Maruyama Tsubasa, Tada Mitsunori
2023
amputee locomotion, central pattern generator, neuromusculoskeletal model, pathological locomotion, sensory feedback, unilateral transtibial amputation
How social media expression can reveal personality.
In Frontiers in psychiatry
BACKGROUND :
METHODS :
RESULTS :
CONCLUSION :
Han Nuo, Li Sijia, Huang Feng, Wen Yeye, Su Yue, Li Linyan, Liu Xiaoqian, Zhu Tingshao
2023
Big Five, domain knowledge, machine learning, mental health, personality, psychological lexicons, social media
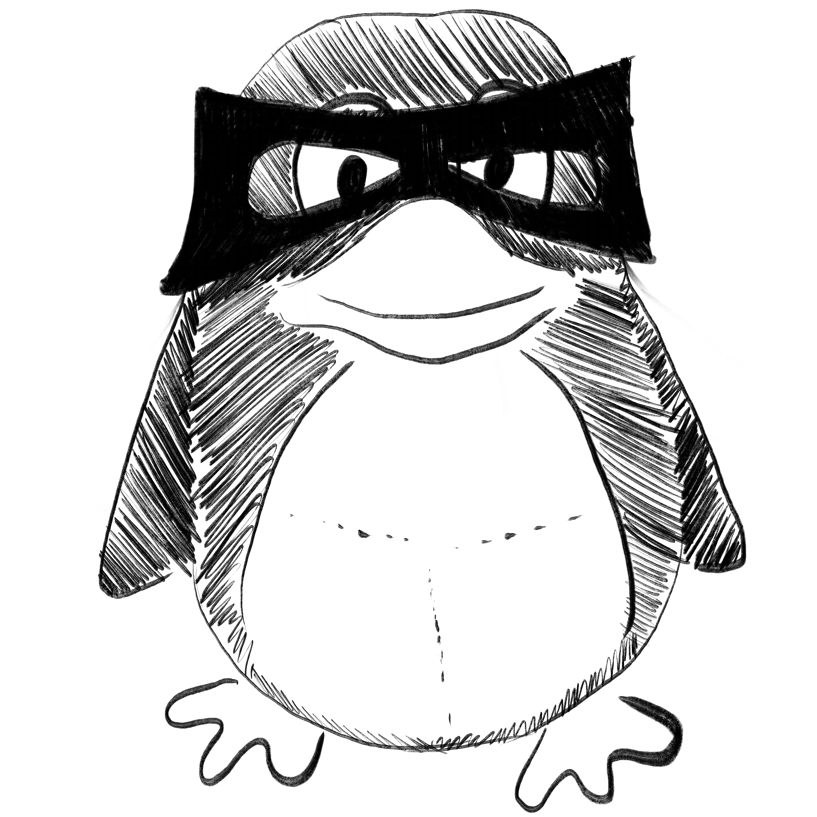
Incorporating multi-stage diagnosis status to mine associations between genetic risk variants and the multi-modality phenotype network in major depressive disorder.
In Frontiers in psychiatry
Zhang Li, Pang Mengqian, Liu Xiaoyun, Hao Xiaoke, Wang Meiling, Xie Chunming, Zhang Zhijun, Yuan Yonggui, Zhang Daoqiang
2023
imaging genetics, major depressive disorder, multi-modality, multi-stage diagnosis status, single-nucleotide polymorphisms
Weekly Summary
Receive a weekly summary and discussion of the top papers of the week by leading researchers in the field.