Receive a weekly summary and discussion of the top papers of the week by leading researchers in the field.
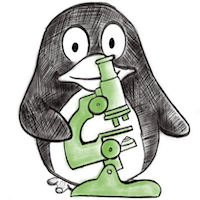
Uncovering spatiotemporal patterns of atrophy in progressive supranuclear palsy using unsupervised machine learning.
In Brain communications
Scotton William J, Shand Cameron, Todd Emily, Bocchetta Martina, Cash David M, VandeVrede Lawren, Heuer Hilary, Young Alexandra L, Oxtoby Neil, Alexander Daniel C, Rowe James B, Morris Huw R, Boxer Adam L, Rohrer Jonathan D, Wijeratne Peter A
2023
Subtype and Stage Inference, biomarkers, disease progression, machine learning, progressive supranuclear palsy
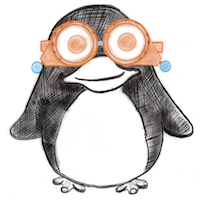
CMS-NET: deep learning algorithm to segment and quantify the ciliary muscle in swept-source optical coherence tomography images.
In Therapeutic advances in chronic disease
BACKGROUND :
OBJECTIVES :
DESIGN :
METHODS :
RESULTS :
CONCLUSION :
Chen Wen, Yu Xiangle, Ye Yiru, Gao Hebei, Cao Xinyuan, Lin Guangqing, Zhang Riyan, Li Zixuan, Wang Xinmin, Zhou Yuheng, Shen Meixiao, Shao Yilei
2023
accommodation, ciliary muscle, deep learning, optical coherence tomography (OCT), presbyopia
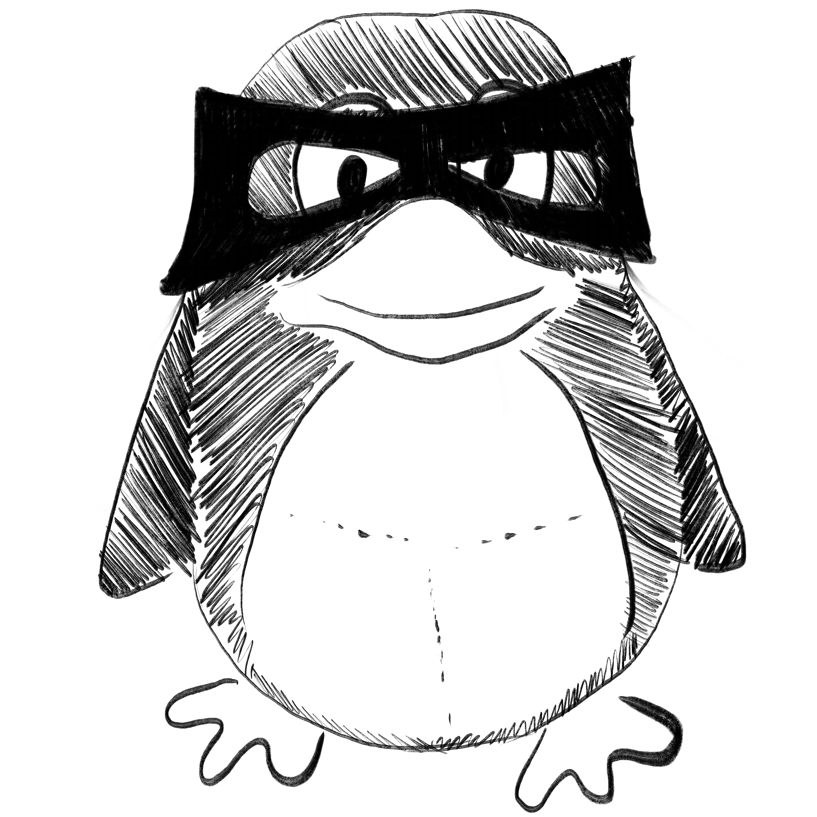
Automated ICD coding for coronary heart diseases by a deep learning method.
In Heliyon
Zhao Shuai, Diao Xiaolin, Xia Yun, Huo Yanni, Cui Meng, Wang Yuxin, Yuan Jing, Zhao Wei
2023-Mar
BERT, Coronary heart diseases, Deep learning, ICD coding, Interpretability
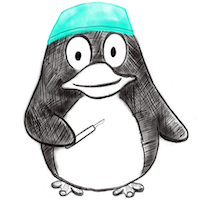
Interactions between silica and titanium nanoparticles and oral and gastrointestinal epithelia: Consequences for inflammatory diseases and cancer.
In Heliyon
Coutinho Almeida-da-Silva Cássio Luiz, Cabido Leticia Ferreira, Chin Wei-Chun, Wang Ge, Ojcius David M, Li Changqing
2023-Mar
Cancer, Cytotoxicity, DAMP, damage-assocaited molecular pattern, Epithelium, FBG, foreign body gingivitis, Imaging, Inflammasome, Inflammation, NP, nanoparticle, Nanoparticles, PAMP, pathogen-assocaited molecular pattern, ROS, reactive oxygen species
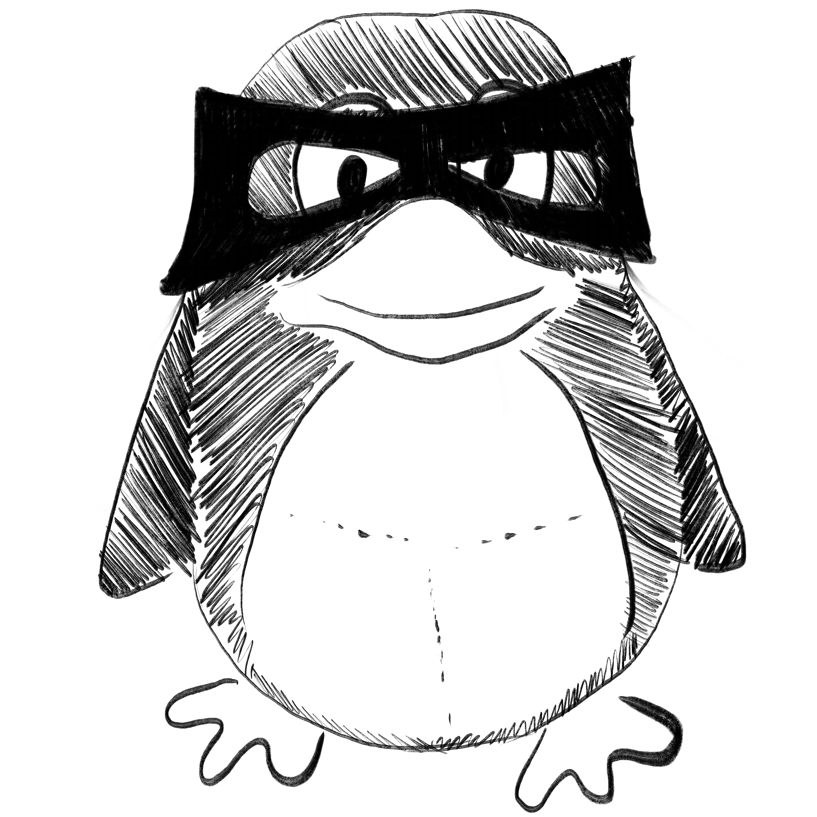
COVID-19 and pneumonia diagnosis from chest X-ray images using convolutional neural networks.
In Network modeling and analysis in health informatics and bioinformatics
Hariri Muhab, Avşar Ercan
2023
COVID-19, Classification, Convolutional neural networks, Deep learning, Lung diseases, Transfer learning
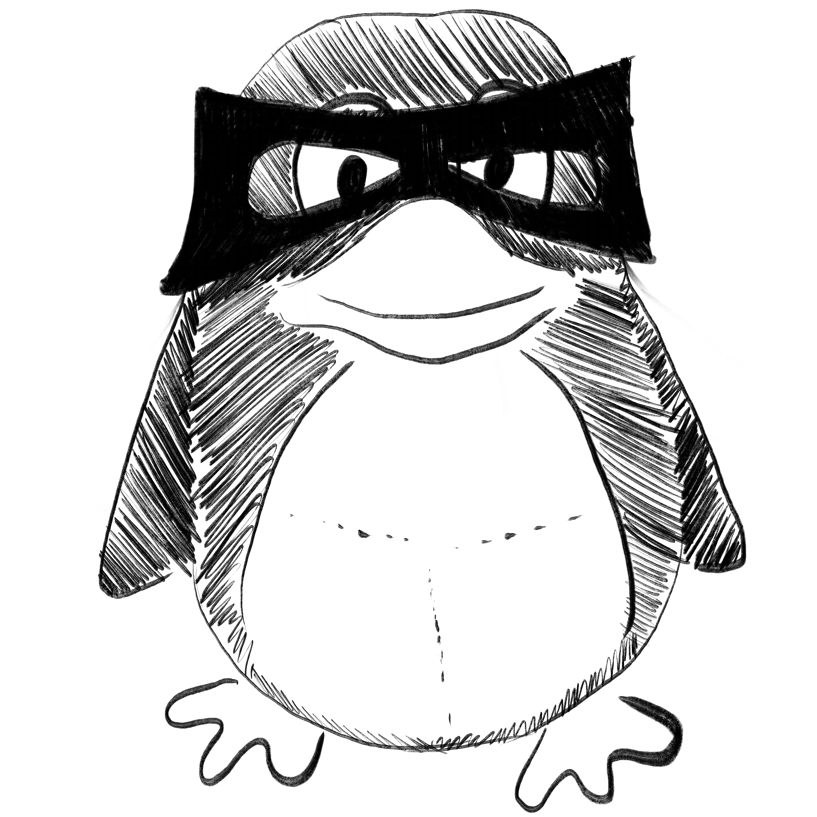
An accessible and versatile deep learning-based sleep stage classifier.
In Frontiers in neuroinformatics
Hanna Jevri, Flöel Agnes
2023
EEG, classification, deep learning, machine learning, sleep
Weekly Summary
Receive a weekly summary and discussion of the top papers of the week by leading researchers in the field.