Receive a weekly summary and discussion of the top papers of the week by leading researchers in the field.
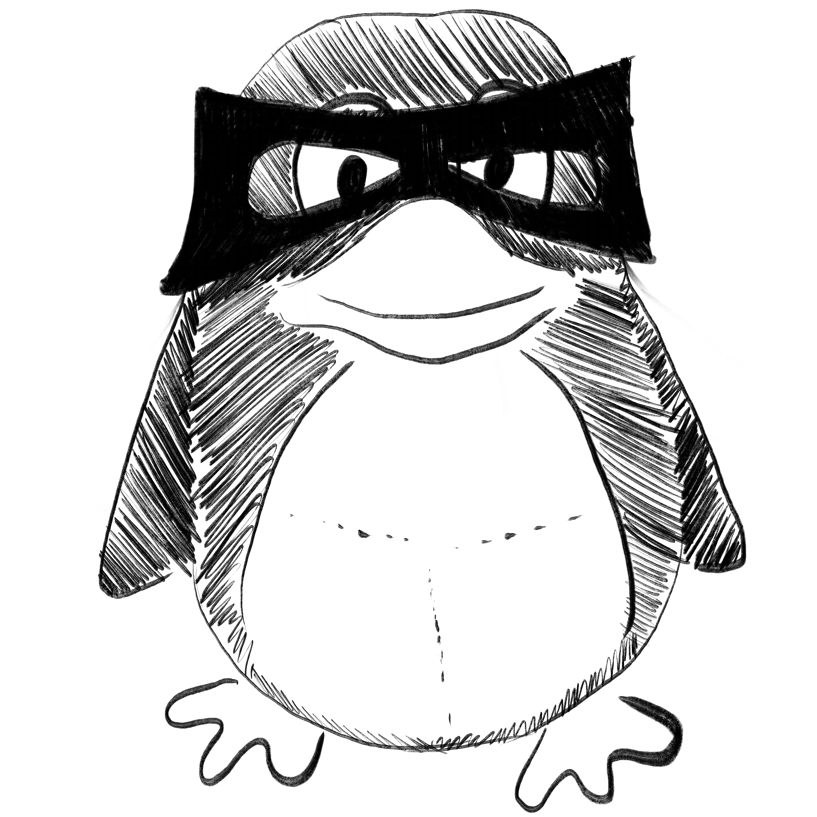
CBCovid19EC: A dataset complete blood count and PCR test for COVID-19 detection in Ecuadorian population.
In Data in brief
Ordoñez-Avila R, Parraga-Alava J, Hormaza J Meza, Vaca-Cárdenas L, Portmann E, Terán L, Dorn M
2023-Apr
Ecuador, Hematological data, Machine learning, SARS-Cov-2
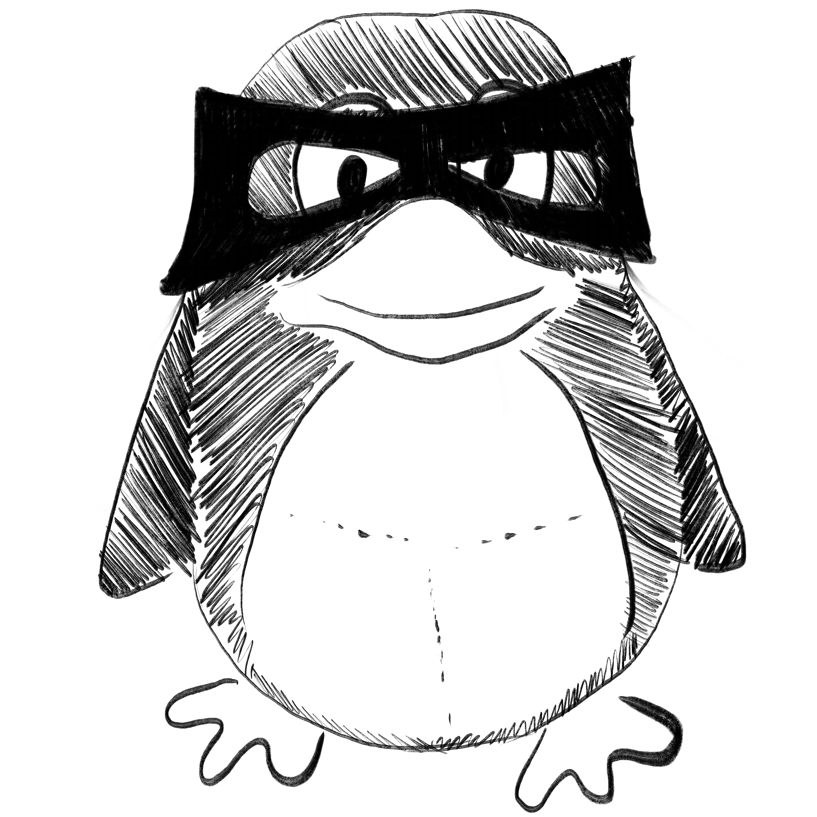
Image dataset of urine test results on petri dishes for deep learning classification.
In Data in brief
da Silva Gabriel Rodrigues, Rosmaninho Igor Batista, Zancul Eduardo, de Oliveira Vanessa Rita, Francisco Gabriela Rodrigues, Dos Santos Nathamy Fernanda, de Mello Macêdo Karin, da Silva Amauri José, de Lima Érika Knabben, Lemo Mara Elisa Borsato, Maldonado Alessandra, Moura Maria Emilia G, da Silva Flávia Helena, Guimarães Gustavo Stuani
2023-Apr
Computational Vision, Image Classification, Petri Dish, Urine Test Classification
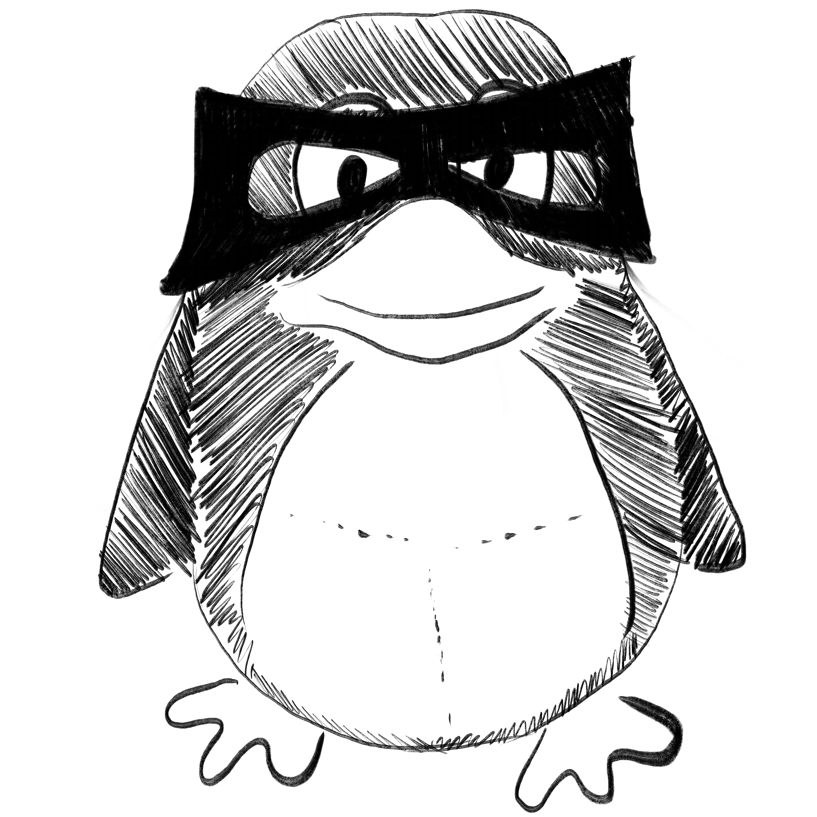
Using machine learning prediction models for quality control: a case study from the automotive industry.
In Computational management science
Msakni Mohamed Kais, Risan Anders, Schütz Peter
2023
Manufacturing, Neural network, Quality control, Random forest
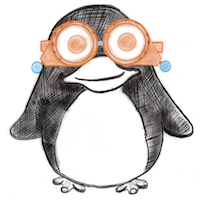
Latest Trends in Retinopathy of Prematurity: Research on Risk Factors, Diagnostic Methods and Therapies.
In International journal of general medicine
Bujoreanu Bezman Laura, Tiutiuca Carmen, Totolici Geanina, Carneciu Nicoleta, Bujoreanu Florin Ciprian, Ciortea Diana Andreea, Niculet Elena, Fulga Ana, Alexandru Anamaria Madalina, Stan Daniela Jicman, Nechita Aurel
2023
anti-VEGF, artificial intelligence, optical coherence tomography, retinopathy of prematurity, risk factors, telescreening
Assessment of a combined musculoskeletal and chest deep learning-based detection solution in an emergency setting.
In European journal of radiology open
RATIONALE AND OBJECTIVES :
MATERIAL AND METHODS :
RESULTS :
CONCLUSION :
Parpaleix Alexandre, Parsy Clémence, Cordari Marina, Mejdoubi Mehdi
2023
Add-on, Chest, Deep learning, Emergency, Musculoskeletal, Xray
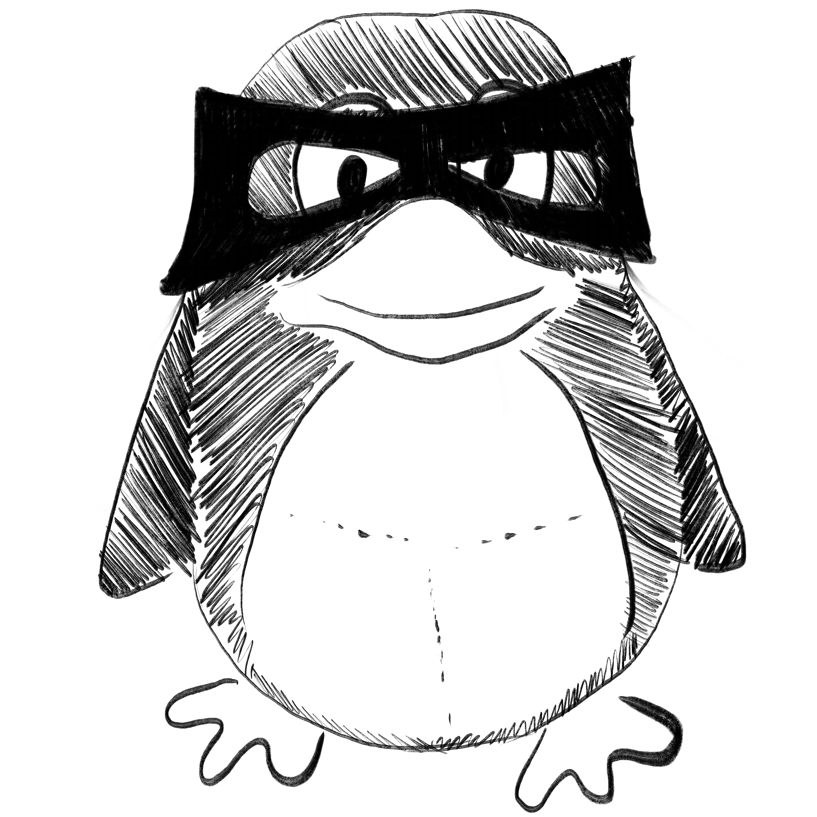
Chemical rules for optimization of chemical mutagenicity via matched molecular pairs analysis and machine learning methods.
In Journal of cheminformatics
Lou Chaofeng, Yang Hongbin, Deng Hua, Huang Mengting, Li Weihua, Liu Guixia, Lee Philip W, Tang Yun
2023-Mar-20
Consensus model, Lead optimization, Machine learning, Matched molecular pairs analysis, Mutagenicity optimization
Weekly Summary
Receive a weekly summary and discussion of the top papers of the week by leading researchers in the field.