Receive a weekly summary and discussion of the top papers of the week by leading researchers in the field.
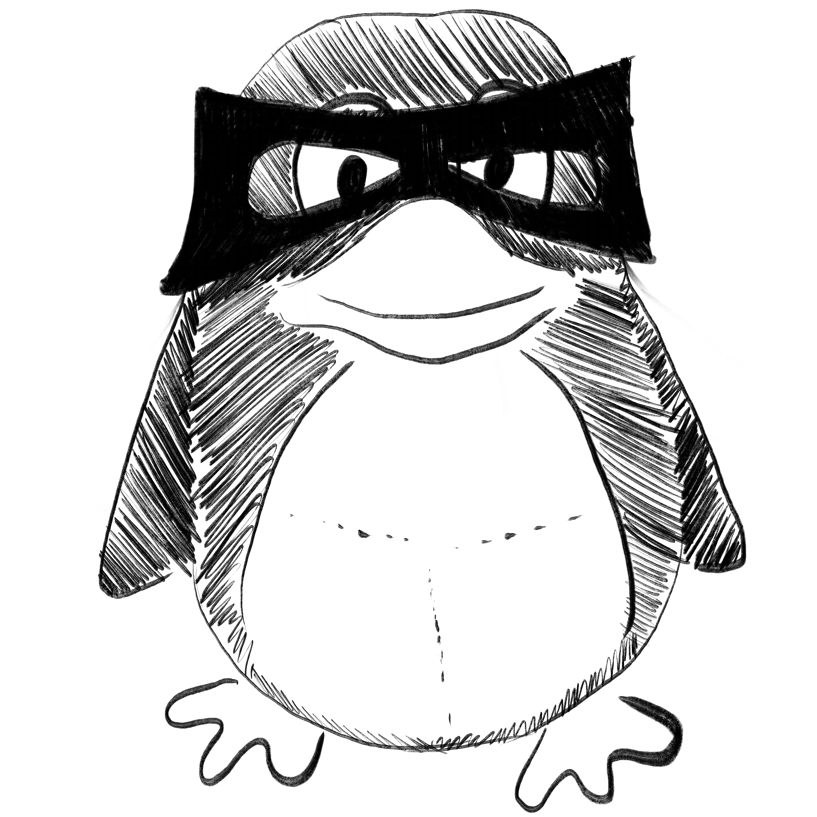
Identifying Disease of Interest With Deep Learning Using Diagnosis Code.
In Journal of Korean medical science
BACKGROUND :
METHODS :
RESULTS :
CONCLUSION :
Cho Yoon-Sik, Kim Eunsun, Stafford Patrick L, Oh Min-Hwan, Kwon Younghoon
2023-Mar-20
Deep Learning, Diagnosis Code, Gastric Cancer, Machine Learning, Prediction
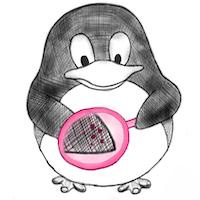
[Progress in research of risk prediction model for chronic kidney disease].
In Zhonghua liu xing bing xue za zhi = Zhonghua liuxingbingxue zazhi
Zeng Z Q, Yang S C, Yu C Q, Zhang L X, Lyu J, Li L M
2023-Mar-10
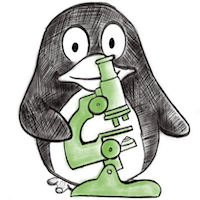
Relationship between the deep features of the full-scan pathological map of mucinous gastric carcinoma and related genes based on deep learning.
In Heliyon
BACKGROUND :
METHODS :
RESULT :
CONCLUSION :
Li Ding, Li Xiaoyuan, Li Shifang, Qi Mengmeng, Sun Xiaowei, Hu Guojie
2023-Mar
Bioinformatics, Deep learning, Genomics, Mucinous gastric carcinoma, Pathomics
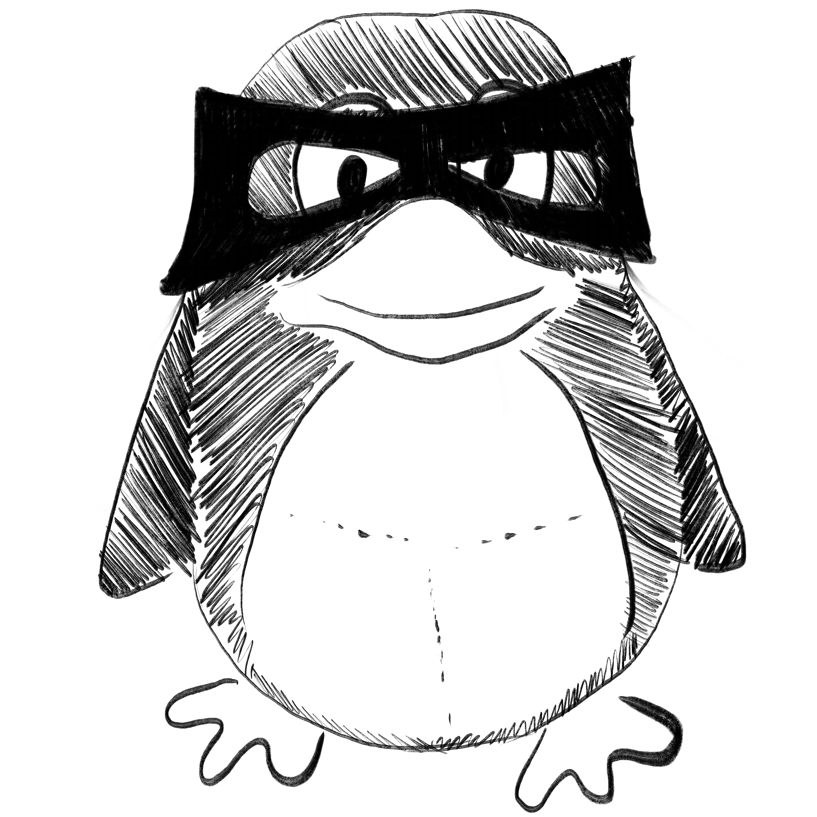
A deep learning approach for lane marking detection applying encode-decode instant segmentation network.
In Heliyon
Al Mamun Abdullah, Em Poh Ping, Hossen Md Jakir, Jahan Busrat, Tahabilder Anik
2023-Mar
ADAS, CalTech, Deep learning, Lane markings detection, Segmentation, Tusimple
Acceptability and Effectiveness of COVID-19 Contact Tracing Applications: A Case Study in Saudi Arabia of the Tawakkalna Application.
In Cureus
Dawood Safia, AlKadi Khulud
2023-Feb
acceptability, contact tracing, covid-19, mhealth, permission, privacy, privilege, security, tawakkalna
Racial Equity in Healthcare Machine Learning: Illustrating Bias in Models With Minimal Bias Mitigation.
In Cureus
Barton Michael, Hamza Mahmoud, Guevel Borna
2023-Feb
data science, health equity, healthcare technology, machine learning, racial bias
Weekly Summary
Receive a weekly summary and discussion of the top papers of the week by leading researchers in the field.