Receive a weekly summary and discussion of the top papers of the week by leading researchers in the field.
AI Cardiac MRI Scar Analysis Aids Prediction of Major Arrhythmic Events in the Multicenter DERIVATE Registry.
In Radiology ; h5-index 91.0
Ghanbari Fahime, Joyce Thomas, Lorenzoni Valentina, Guaricci Andrea I, Pavon Anna-Giulia, Fusini Laura, Andreini Daniele, Rabbat Mark G, Aquaro Giovanni Donato, Abete Raffaele, Bogaert Jan, Camastra Giovanni, Carigi Samuela, Carrabba Nazario, Casavecchia Grazia, Censi Stefano, Cicala Gloria, De Cecco Carlo N, De Lazzari Manuel, Di Giovine Gabriella, Di Roma Mauro, Focardi Marta, Gaibazzi Nicola, Gismondi Annalaura, Gravina Matteo, Lanzillo Chiara, Lombardi Massimo, Lozano-Torres Jordi, Masi Ambra, Moro Claudio, Muscogiuri Giuseppe, Nese Alberto, Pradella Silvia, Sbarbati Stefano, Schoepf U Joseph, Valentini Adele, Crelier Gérard, Masci Pier Giorgio, Pontone Gianluca, Kozerke Sebastian, Schwitter Juerg
2023-Mar-21
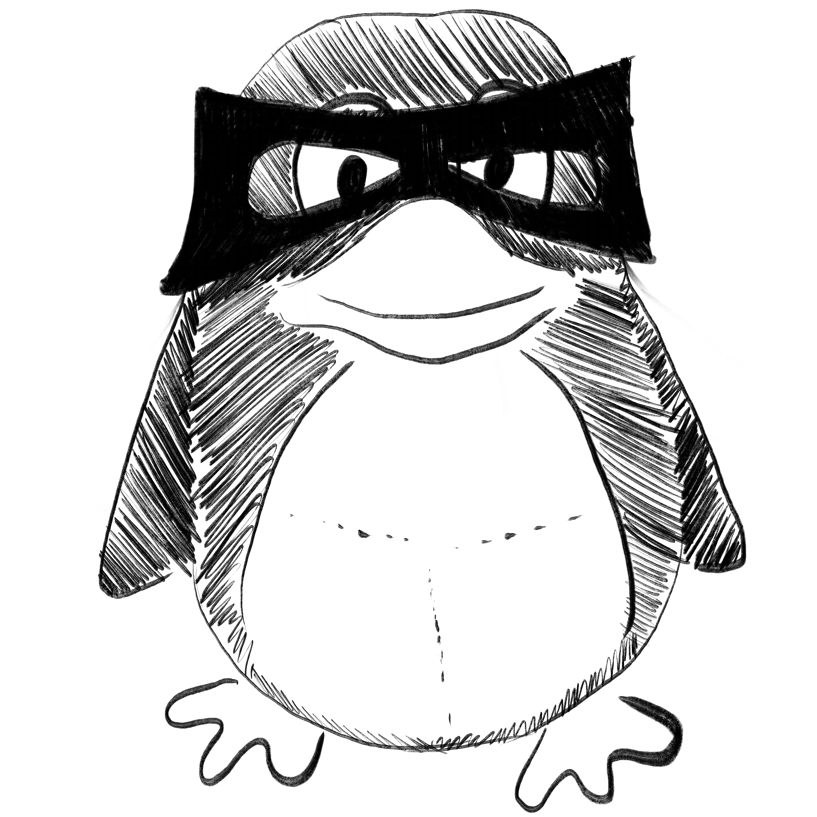
Paradoxical decrease of imitation performance with age in children.
In British journal of psychology (London, England : 1953)
Ottoboni Giovanni, Toraldo Alessio, Proietti Riccardo, Cangelosi Angelo, Tessari Alessia
2023-Mar-21
body knowledge, children, development, double dissociation, imitation, meaningless action, movement complexity, working memory
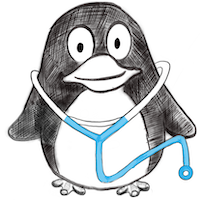
Machine learning to predict major bleeding during anticoagulation for venous thromboembolism: possibilities and limitations.
In British journal of haematology ; h5-index 64.0
Mora Damián, Mateo Jorge, Nieto José A, Bikdeli Behnood, Yamashita Yugo, Barco Stefano, Jimenez David, Demelo-Rodriguez Pablo, Rosa Vladimir, Yoo Hugo Hyung Bok, Sadeghipour Parham, Monreal Manuel
2023-Mar-21
haemorrhage, machine learning, outcomes, pulmonary embolism, venous thrombosis
Convolution Neural Network Algorithm for Shockable Arrhythmia Classification Within a Digitally Connected Automated External Defibrillator.
In Journal of the American Heart Association ; h5-index 70.0
Shen Christine P, Freed Benjamin C, Walter David P, Perry James C, Barakat Amr F, Elashery Ahmad Ramy A, Shah Kevin S, Kutty Shelby, McGillion Michael, Ng Fu Siong, Khedraki Rola, Nayak Keshav R, Rogers John D, Bhavnani Sanjeev P
2023-Mar-21
ECG, automated external defibrillator, convolution neural network, machine learning, ventricular arrhythmias
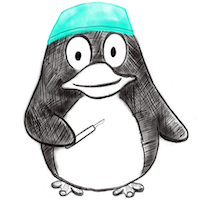
Artificial Intelligence-enabled Decision Support in Surgery: State-of-the-art and Future Directions.
In Annals of surgery ; h5-index 104.0
OBJECTIVE :
SUMMARY BACKGROUND DATA :
METHODS :
RESULTS :
CONCLUSIONS :
Loftus Tyler J, Altieri Maria S, Balch Jeremy A, Abbott Kenneth L, Choi Jeff, Marwaha Jayson S, Hashimoto Daniel A, Brat Gabriel A, Raftopoulos Yannis, Evans Heather L, Jackson Gretchen P, Walsh Danielle S, Tignanelli Christopher J
2023-Mar-21
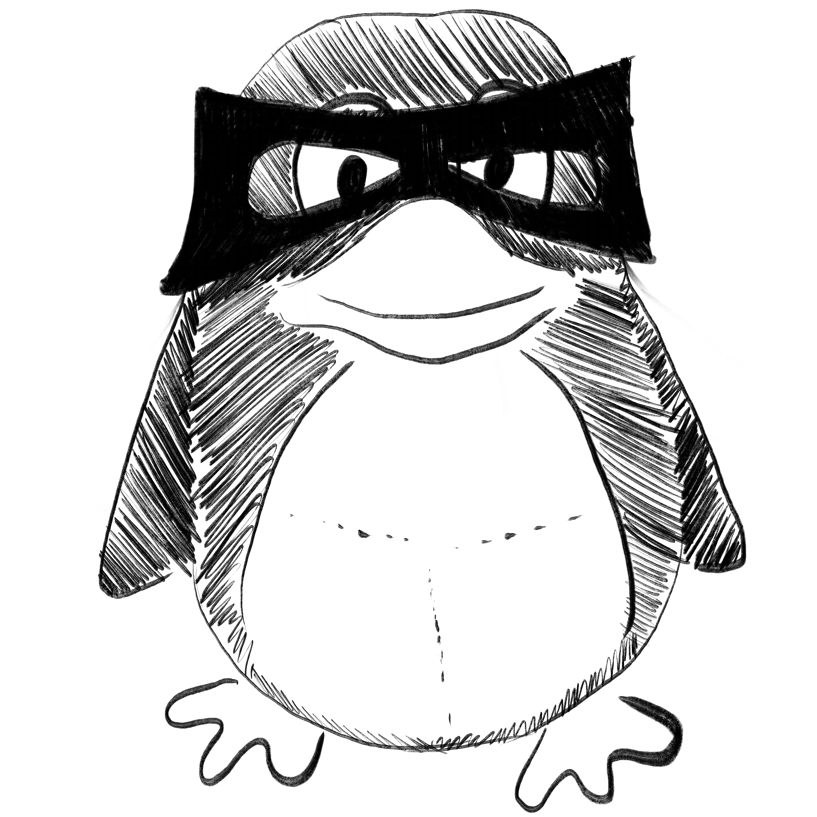
Gender Differences in the Nonspecific and Health-Specific Use of Social Media Before and During the COVID-19 Pandemic: Trend Analysis Using HINTS 2017-2020 Data.
In Journal of health communication ; h5-index 36.0
Ye Linglong, Chen Yang, Cai Yongming, Kao Yi-Wei, Wang Yuanxin, Chen Mingchih, Shia Ben-Chang, Qin Lei
2023-Mar-21
Weekly Summary
Receive a weekly summary and discussion of the top papers of the week by leading researchers in the field.