Receive a weekly summary and discussion of the top papers of the week by leading researchers in the field.
Acceptability and Effectiveness of COVID-19 Contact Tracing Applications: A Case Study in Saudi Arabia of the Tawakkalna Application.
In Cureus
Dawood Safia, AlKadi Khulud
2023-Feb
acceptability, contact tracing, covid-19, mhealth, permission, privacy, privilege, security, tawakkalna
ExBEHRT: Extended Transformer for Electronic Health Records to Predict Disease Subtypes & Progressions
ArXiv Preprint
Maurice Rupp, Oriane Peter, Thirupathi Pattipaka
2023-03-22
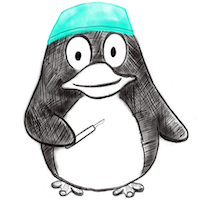
Early Triage of Critically Ill Adult Patients With Mushroom Poisoning: Machine Learning Approach.
In JMIR formative research
BACKGROUND :
OBJECTIVE :
METHODS :
RESULTS :
CONCLUSIONS :
Liu Yuxuan, Lyu Xiaoguang, Yang Bo, Fang Zhixiang, Hu Dejun, Shi Lei, Wu Bisheng, Tian Yong, Zhang Enli, Yang YuanChao
2023-Mar-21
XGBoost, extreme gradient boosting, machine learning, model, mushroom poisoning, triage
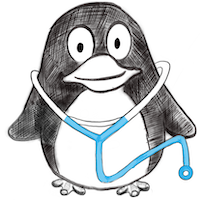
Vitamin D in atherosclerosis and cardiovascular events.
In European heart journal ; h5-index 154.0
Carbone Federico, Liberale Luca, Libby Peter, Montecucco Fabrizio
2023-Mar-21
Adipose tissue, Atherosclerosis, Inflammation, Senescence, Vitamin D, Vitamin D receptor
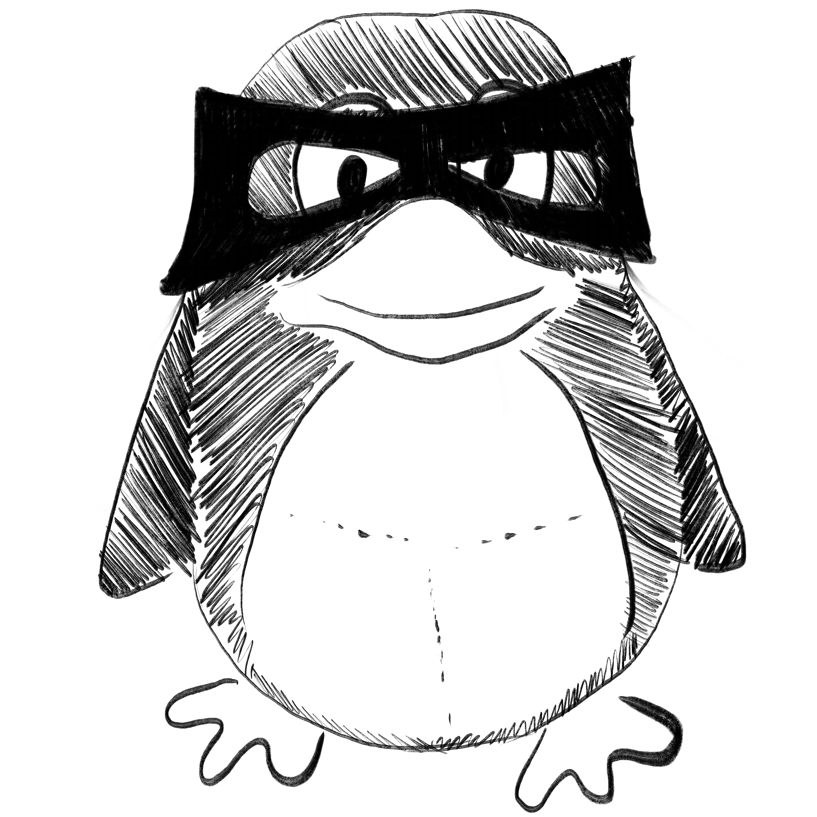
A Standardized Clinical Data Harmonization Pipeline for Scalable AI Application Deployment (FHIR-DHP): Validation and Usability Study.
In JMIR medical informatics ; h5-index 23.0
BACKGROUND :
OBJECTIVE :
METHODS :
RESULTS :
CONCLUSIONS :
Williams Elena, Kienast Manuel, Medawar Evelyn, Reinelt Janis, Merola Alberto, Klopfenstein Sophie Anne Ines, Flint Anne Rike, Heeren Patrick, Poncette Akira-Sebastian, Balzer Felix, Beimes Julian, von Bünau Paul, Chromik Jonas, Arnrich Bert, Scherf Nico, Niehaus Sebastian
2023-Mar-21
AI, AI application, FHIR, MIMIC IV, artificial intelligence, care, care unit, cooperation, data, data interoperability, data standardization pipeline, deployment, diagnosis, fast healthcare interoperability resources, medical information mart for intensive care, medical research, patient care, usability
Heterogeneity in the response to n-3 polyunsaturated fatty acids.
In Current opinion in clinical nutrition and metabolic care
PURPOSE OF REVIEW :
RECENT FINDINGS :
SUMMARY :
Shaikh Saame Raza, Bazinet Richard P
2023-Mar-21
Weekly Summary
Receive a weekly summary and discussion of the top papers of the week by leading researchers in the field.