Receive a weekly summary and discussion of the top papers of the week by leading researchers in the field.
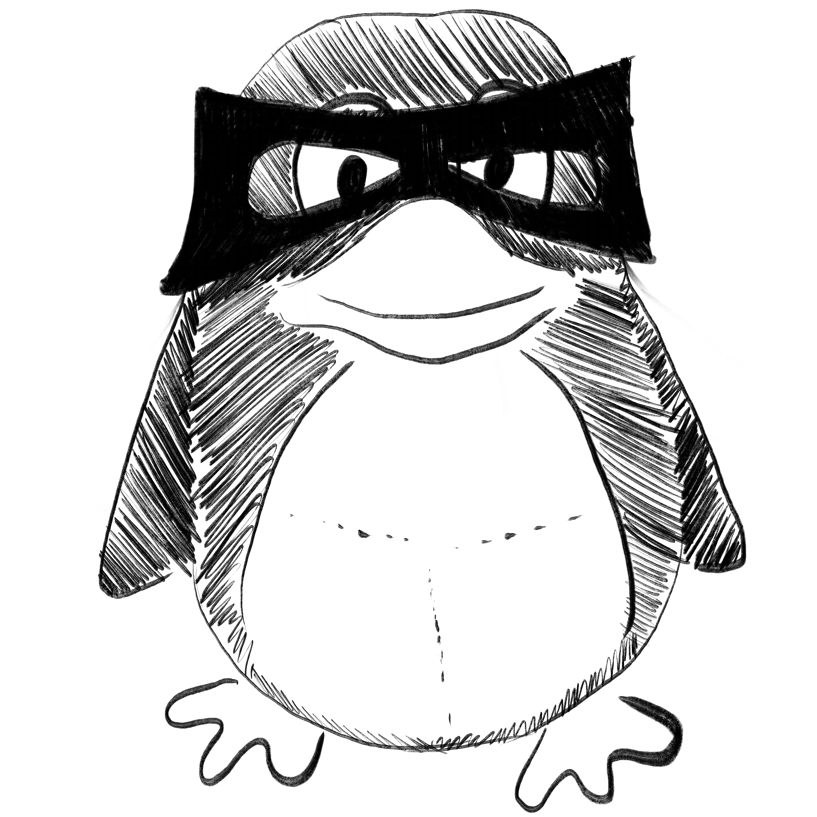
Sparks of Artificial General Intelligence: Early experiments with GPT-4
ArXiv Preprint
Sébastien Bubeck, Varun Chandrasekaran, Ronen Eldan, Johannes Gehrke, Eric Horvitz, Ece Kamar, Peter Lee, Yin Tat Lee, Yuanzhi Li, Scott Lundberg, Harsha Nori, Hamid Palangi, Marco Tulio Ribeiro, Yi Zhang
2023-03-22
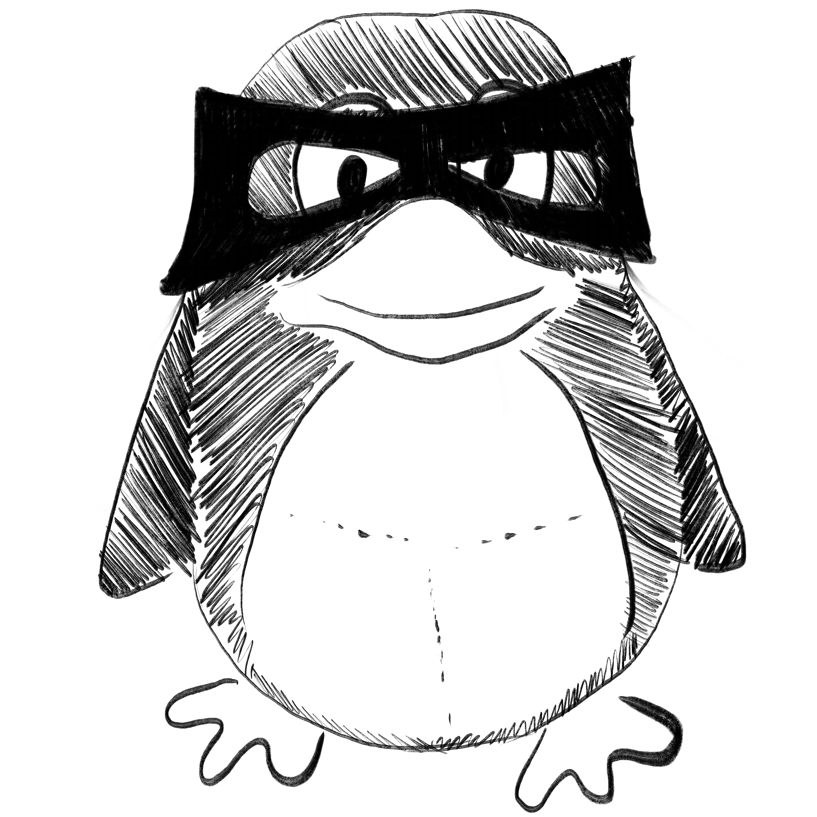
Understanding Social Robots: Attribution of Intentional Agency to Artificial and Biological Bodies.
In Artificial life
Ziemke Tom
2023-Mar-16
Attribution, embodiment, grounding, human–robot interaction, intentionality, observer’s grounding problem, social robots
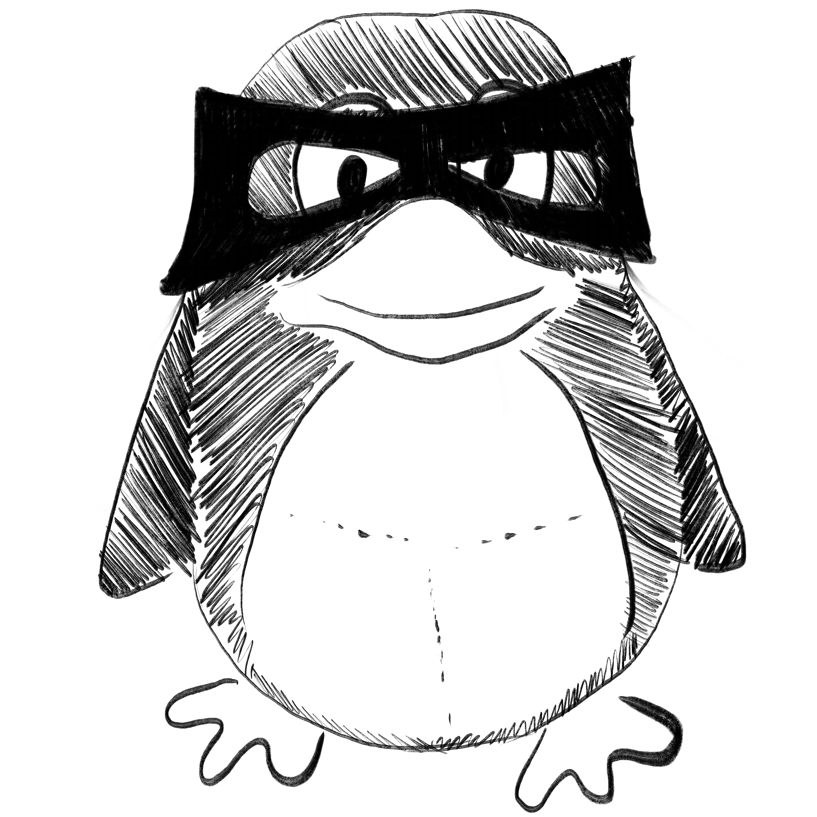
Artificial intelligence based virtual screening study for competitive and allosteric inhibitors of the SARS-CoV-2 main protease.
In Journal of biomolecular structure & dynamics
Charles Ssemuyiga, Edgar Mulumba Pius, Mahapatra Rajani Kanta
2023-Mar-21
Artificial intelligence, COVID-19, SARS-CoV-2 main protease, deep docking, molecular docking, molecular dynamics simulation, neural networks
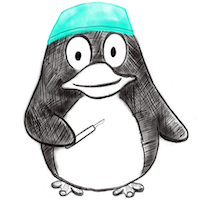
CranioRate TM: An Image-Based, Deep-Phenotyping Analysis Toolset and Online Clinician Interface for Metopic Craniosynostosis.
In Plastic and reconstructive surgery ; h5-index 62.0
INTRODUCTION :
METHODS :
RESULTS :
CONCLUSION :
Beiriger Justin W, Tao Wenzheng, Bruce Madeleine K, Anstadt Erin, Christensen Cameron, Smetona John, Whitaker Ross, Goldstein Jesse
2023-Mar-22
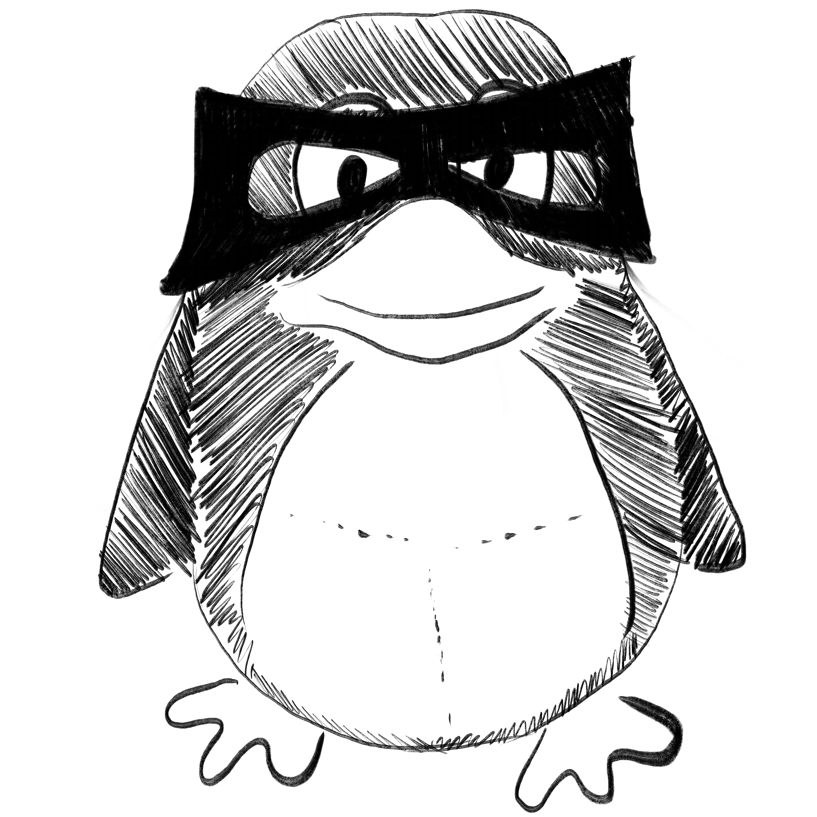
The Role of MicroRNAs in Predicting the Neurological Outcome of Patients with Subarachnoid Hemorrhage: A Meta-analysis.
In Cellular and molecular neurobiology
Li Jian, Liu Wei, Anniwaer Ankaerjiang, Li Bo, Chen Yutang, Yu Zhaoxia, Yu Xiangyou
2023-Mar-21
Machine learning, Meta analysis, Subarachnoid hemorrhage, microRNAs
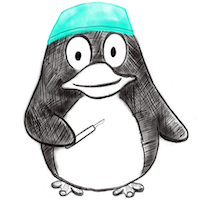
Deep learning-based high-accuracy detection for lumbar and cervical degenerative disease on T2-weighted MR images.
In European spine journal : official publication of the European Spine Society, the European Spinal Deformity Society, and the European Section of the Cervical Spine Research Society
PURPOSE :
METHODS :
RESULTS :
CONCLUSION :
Yi Wei, Zhao Jingwei, Tang Wen, Yin Hongkun, Yu Lifeng, Wang Yaohui, Tian Wei
2023-Mar-21
Deep learning, Degenerative disc disease, Magnet resonance imaging, Spine
Weekly Summary
Receive a weekly summary and discussion of the top papers of the week by leading researchers in the field.