Receive a weekly summary and discussion of the top papers of the week by leading researchers in the field.
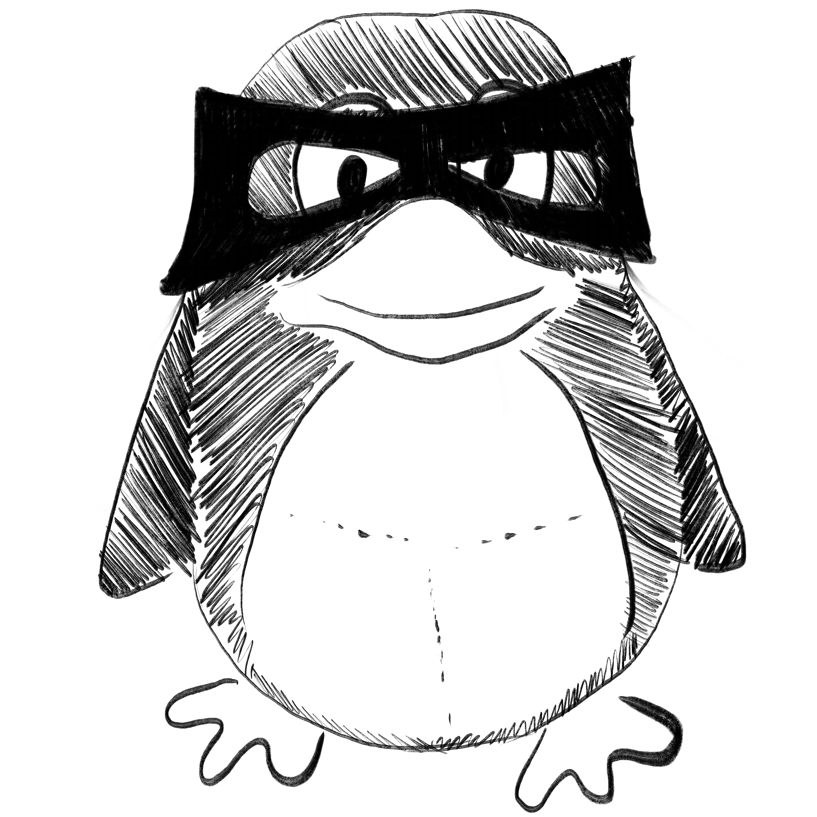
The debate over understanding in AI's large language models.
In Proceedings of the National Academy of Sciences of the United States of America
Mitchell Melanie, Krakauer David C
2023-Mar-28
artificial intelligence, large language models, understanding
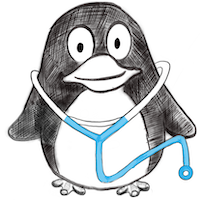
Association between urinary albumin creatinine ratio and cardiovascular disease.
In PloS one ; h5-index 176.0
INTRODUCTION :
METHOD :
RESULTS :
CONCLUSION :
Kim Yoo Jin, Hwang Sang Won, Lee Taesic, Lee Jun Young, Uh Young
2023
Prevalence of and factors associated with intimate partner violence victimhood among men who have sex with men in Guangzhou, China.
In Sexually transmitted diseases
BACKGROUND :
AIMS :
METHODS :
RESULTS :
CONCLUSIONS :
Lin Kaihao, Tan Zhimin, Li Jing, Cheng Weibin, Yang Yi, Jiang Hongbo
2023-Mar-22
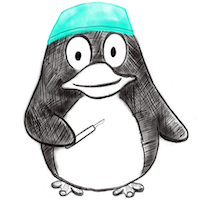
Expanding Cosmetic Plastic Surgery Research Using ChatGPT.
In Aesthetic surgery journal ; h5-index 35.0
BACKGROUND :
OBJECTIVES :
METHODS :
RESULTS :
CONCLUSIONS :
Gupta Rohun, Park John B, Bisht Chirag, Herzog Isabel, Weisberger Joseph, Chao John, Chaiyasate Kongkrit, Lee Edward S
2023-Mar-21
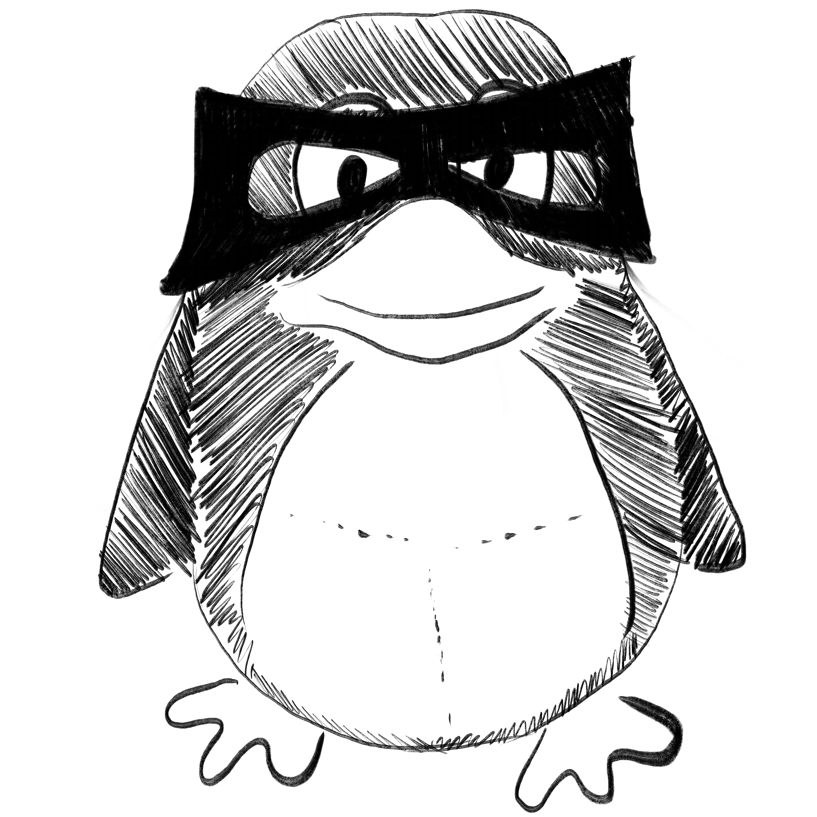
Protein structure prediction with in-cell photo-crosslinking mass spectrometry and deep learning.
In Nature biotechnology ; h5-index 151.0
Stahl Kolja, Graziadei Andrea, Dau Therese, Brock Oliver, Rappsilber Juri
2023-Mar-20
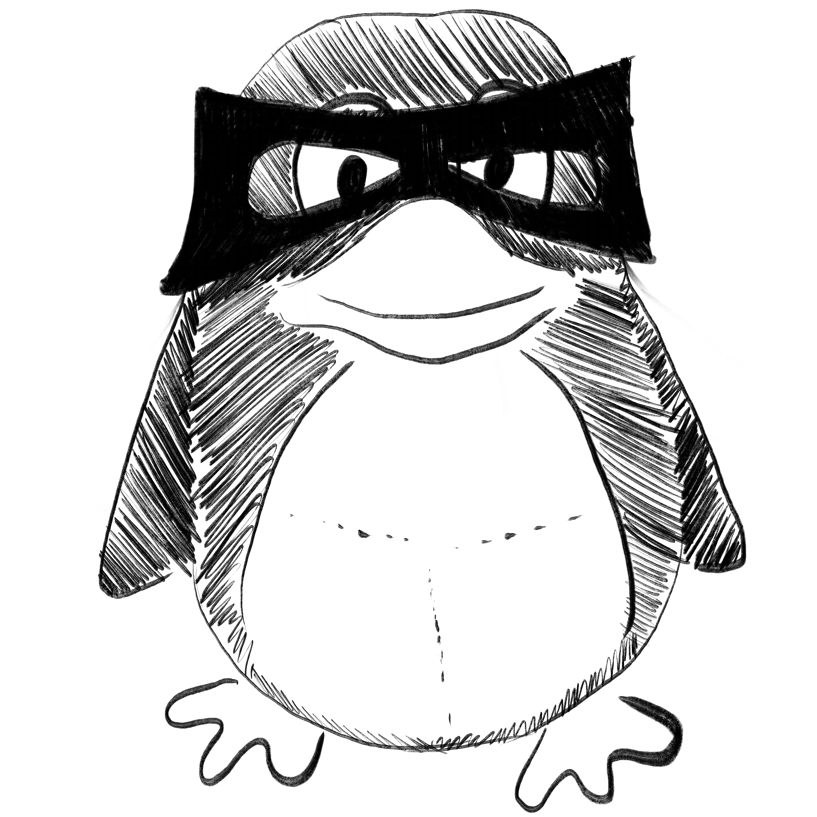
Relationship Between Coronavirus Disease 2019 Vaccination Rates and Rare But Potentially Fatal Adverse Events: A Regression Discontinuity Analysis of Western Countries.
In Journal of Korean medical science
BACKGROUND :
METHODS :
RESULTS :
CONCLUSION :
Chae Seung Hoon, Park Hyung Jun, Radnaabaatar Munkhzul, Park Hojun, Jung Jaehun
2023-Mar-20
COVID-19, Regression Discontinuity Analysis, SARS-CoV-2
Weekly Summary
Receive a weekly summary and discussion of the top papers of the week by leading researchers in the field.