Receive a weekly summary and discussion of the top papers of the week by leading researchers in the field.
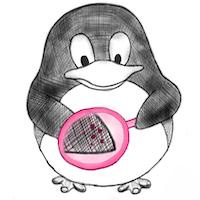
Artificial intelligence for the classification of pigmented skin lesions in populations with skin of colour: A systematic review.
In Dermatology (Basel, Switzerland)
Liu Yuyang, Primiero Clare A, Kulkarni Vishnutheertha, Soyer H Peter, Betz-Stablein Brigid
2023-Mar-21
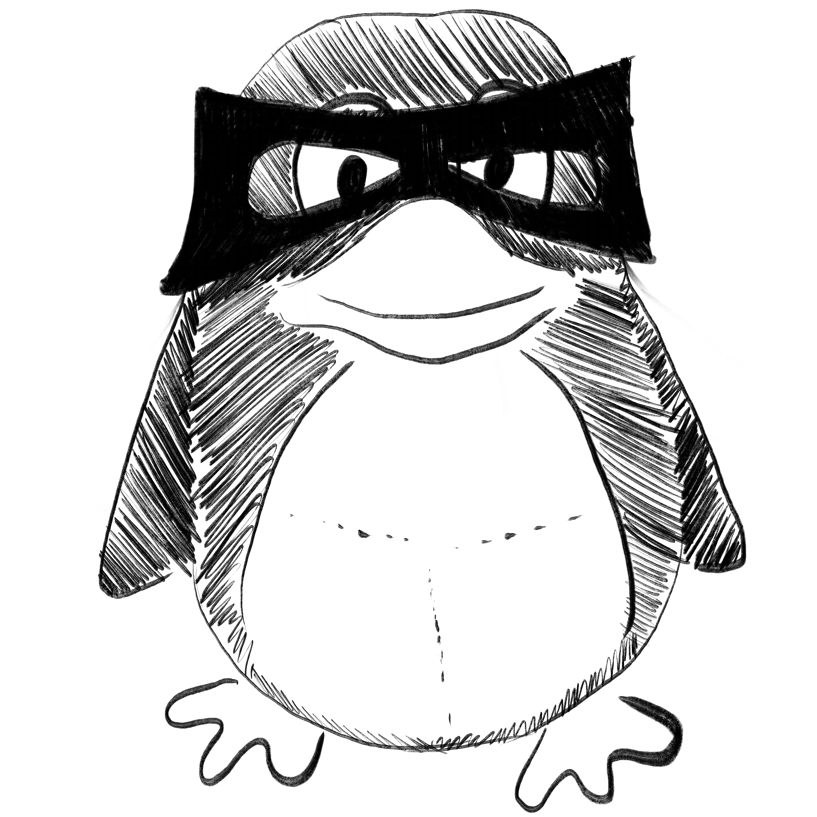
Lower body kinematics estimation during walking using an accelerometer.
In Journal of biomechanics
Mantashloo Zahed, Abbasi Ali, Tazji Mehdi Khaleghi, Pedram Mir Mohsen
2023-Mar-17
Accelerometer, Gait analysis, Joint angle, Machine learning, Random forest, Statistical parametric mapping
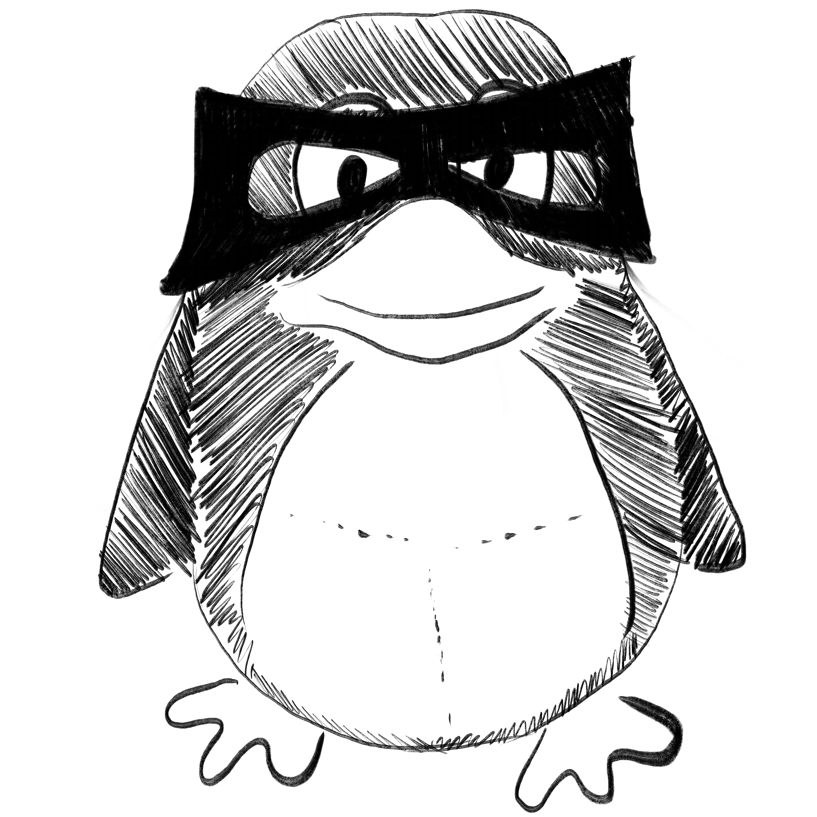
Metabolic and Inflammatory profiles define phenotypes with clinical relevance in female knee osteoarthritis patients with joint effusion.
In Rheumatology (Oxford, England)
OBJECTIVES :
METHODS :
RESULTS :
CONCLUSION :
Calvet Joan, García-Manrique María, Berenguer-Llergo Antoni, Orellana Cristóbal, Cirera Silvia Garcia, Llop Maria, Lencastre Carlos Galisteo, Arévalo Marta, Aymerich Cristina, Gómez Rafael, Giménez Néstor Albiñana, Gratacó Jordi
2023-Mar-21
Knee osteoarthritis, clinical severity, inflammatory, machine learning, metabolism, phenotype
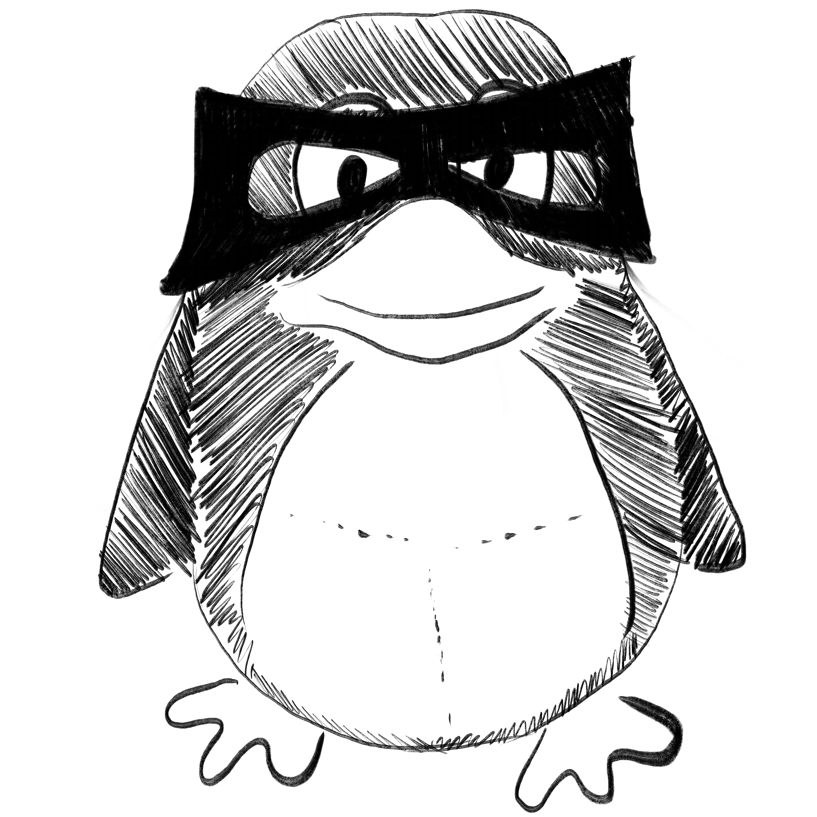
Phenonaut; multiomics data integration for phenotypic space exploration.
In Bioinformatics (Oxford, England)
SUMMARY :
AVAILABILITY AND IMPLEMENTATION :
SUPPLEMENTARY INFORMATION :
Shave Steven, Dawson John C, Athar Abdullah M, Nguyen Cuong Q, Kasprowicz Richard, Carragher Neil O
2023-Mar-21
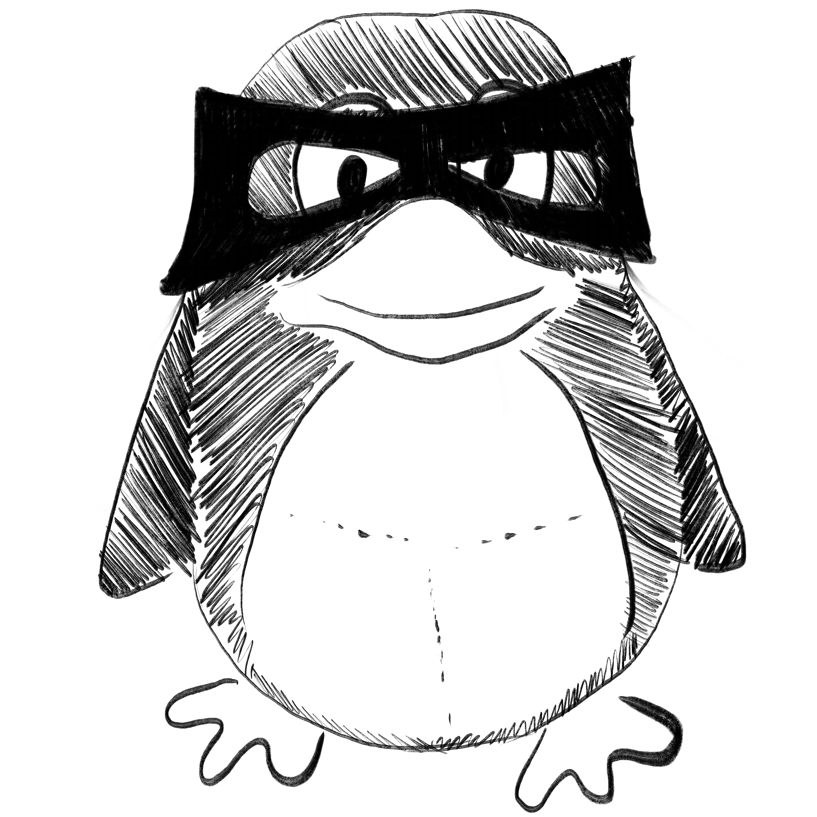
Racial difference in the association between non-alcoholic fatty liver disease and incident type 2 diabetes: findings from the CARDIA study.
In Diabetologia ; h5-index 79.0
AIMS/HYPOTHESIS :
METHODS :
RESULTS :
CONCLUSIONS/INTERPRETATION :
Hatano Yu, VanWagner Lisa B, Carnethon Mercedes R, Bancks Michael P, Carson April P, Lloyd-Jones Donald M, Østbye Truls, Viera Anthony J, Yano Yuichiro
2023-Mar-21
Machine learning, Non-alcoholic fatty liver disease, Racial difference, Type 2 diabetes
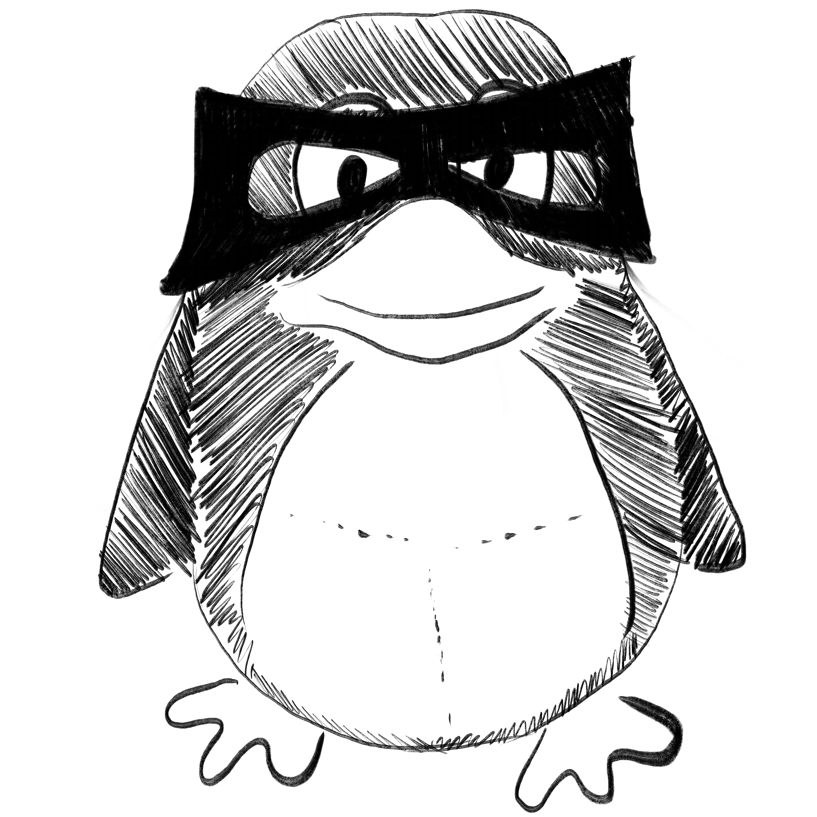
Gender Differences in the Nonspecific and Health-Specific Use of Social Media Before and During the COVID-19 Pandemic: Trend Analysis Using HINTS 2017-2020 Data.
In Journal of health communication ; h5-index 36.0
Ye Linglong, Chen Yang, Cai Yongming, Kao Yi-Wei, Wang Yuanxin, Chen Mingchih, Shia Ben-Chang, Qin Lei
2023-Mar-21
Weekly Summary
Receive a weekly summary and discussion of the top papers of the week by leading researchers in the field.