Receive a weekly summary and discussion of the top papers of the week by leading researchers in the field.
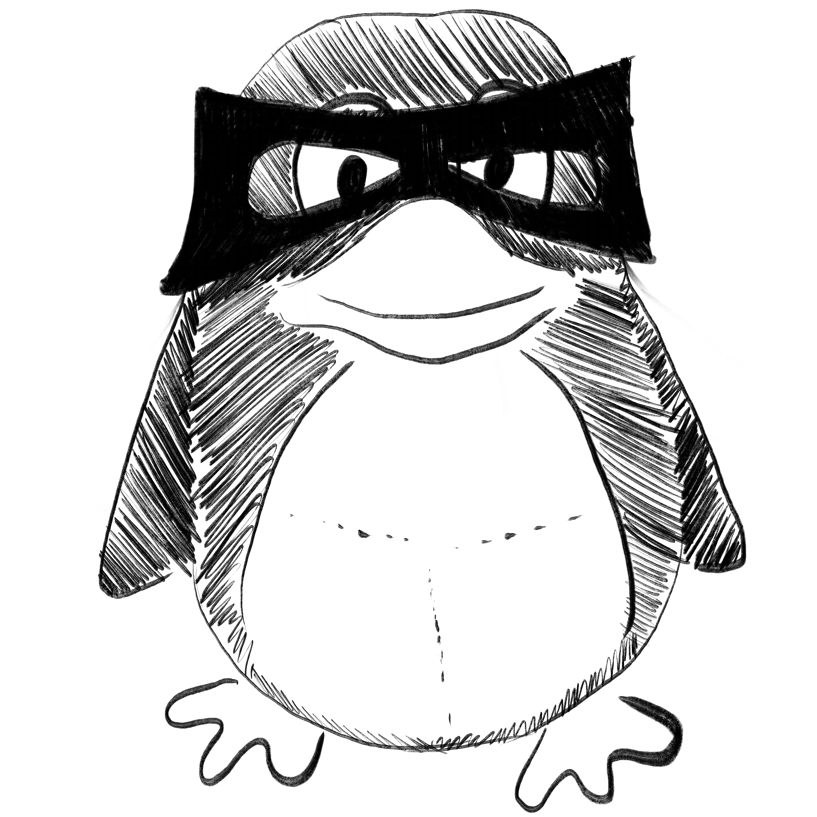
Machine Learning for Predicting Micro- and Macrovascular Complications in Individuals With Prediabetes or Diabetes: Retrospective Cohort Study.
In Journal of medical Internet research ; h5-index 88.0
BACKGROUND :
OBJECTIVE :
METHODS :
RESULTS :
CONCLUSIONS :
Schallmoser Simon, Zueger Thomas, Kraus Mathias, Saar-Tsechansky Maytal, Stettler Christoph, Feuerriegel Stefan
2023-Feb-27
diabetes, machine learning, macrovascular complications, microvascular complications, prediabetes
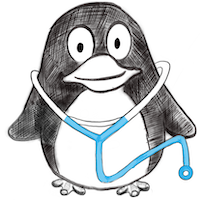
Developing supervised machine learning algorithms to evaluate the therapeutic effect and laboratory-related adverse events of cyclosporine and tacrolimus in renal transplants.
In International journal of clinical pharmacy
BACKGROUND :
AIM :
METHOD :
RESULTS :
CONCLUSION :
Sridharan Kannan, Shah Shamik
2023-Feb-27
Artificial intelligence, Cyclosporine, Immunosuppressants, Machine learning algorithms, Tacrolimus
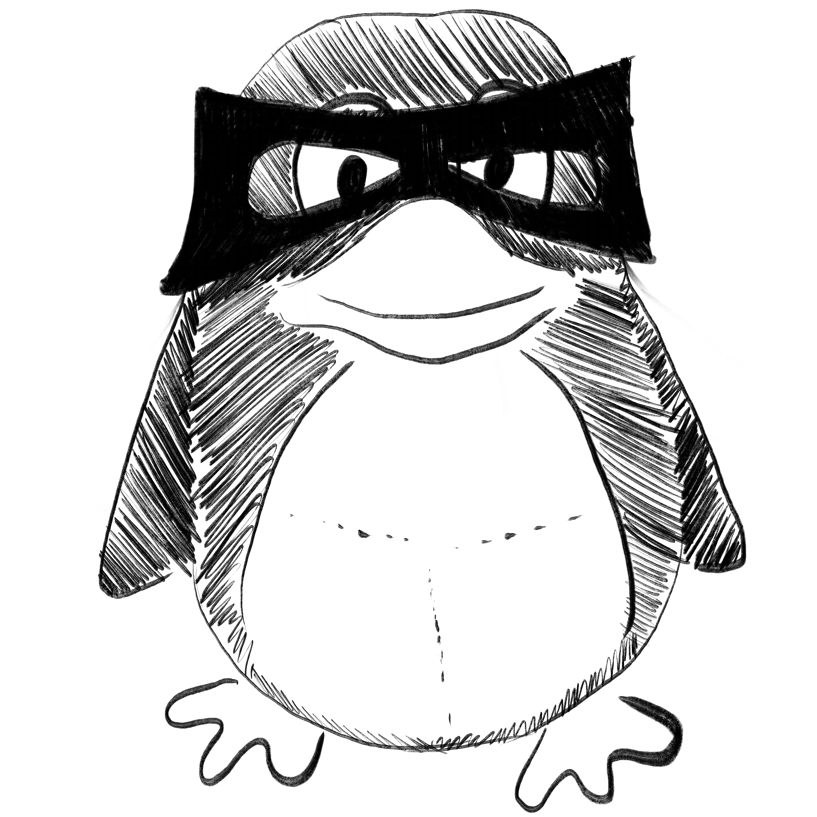
Automatic vertebral fracture and three-column injury diagnosis with fracture visualization by a multi-scale attention-guided network.
In Medical & biological engineering & computing ; h5-index 32.0
Zhang Shunan, Zhao Ziqi, Qiu Lu, Liang Duan, Wang Kun, Xu Jun, Zhao Jun, Sun Jianqi
2023-Feb-27
Deep learning, Disease attention map, Fracture visualization, Three-column injury, Vertebral fracture diagnosis
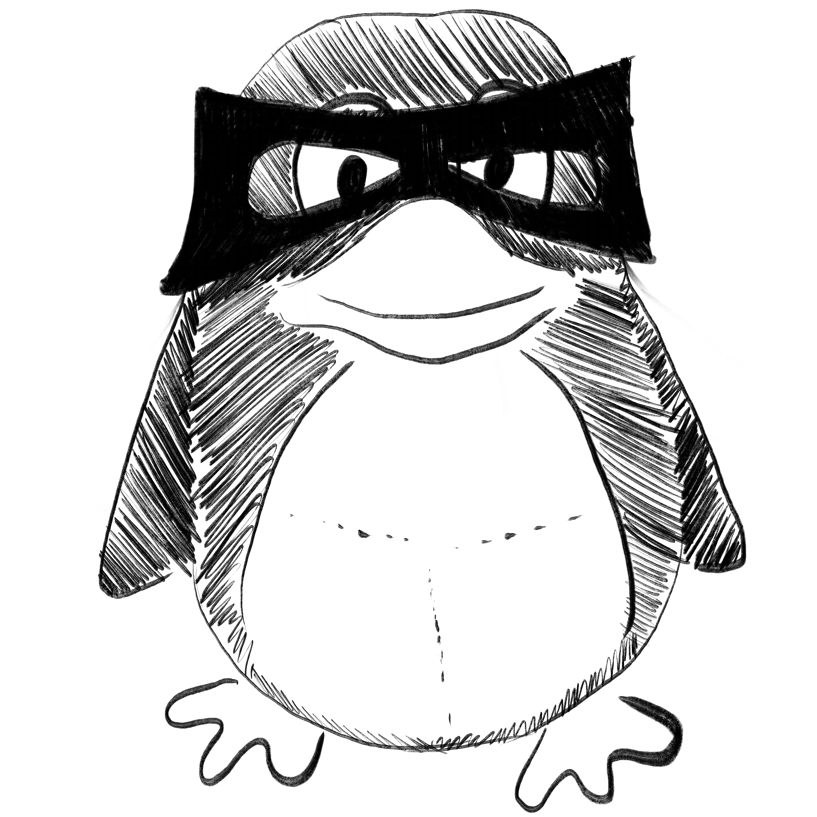
Prediction of gestational diabetes using deep learning and Bayesian optimization and traditional machine learning techniques.
In Medical & biological engineering & computing ; h5-index 32.0
Kurt Burçin, Gürlek Beril, Keskin Seda, Özdemir Sinem, Karadeniz Özlem, Kırkbir İlknur Buçan, Kurt Tuğba, Ünsal Serbülent, Kart Cavit, Baki Neslihan, Turhan Kemal
2023-Feb-27
Bayesian optimization, Clinical decision support system, Deep learning, Gestational diabetes (GD), Random forest, SVM
Deep learning image reconstruction algorithm: impact on image quality in coronary computed tomography angiography.
In La Radiologia medica
PURPOSE :
MATERIAL AND METHODS :
RESULTS :
CONCLUSION :
De Santis Domenico, Polidori Tiziano, Tremamunno Giuseppe, Rucci Carlotta, Piccinni Giulia, Zerunian Marta, Pugliese Luca, Del Gaudio Antonella, Guido Gisella, Barbato Luca, Laghi Andrea, Caruso Damiano
2023-Feb-27
Artificial intelligence, CCTA, Coronary computed tomography angiography, Deep learning, Image reconstruction
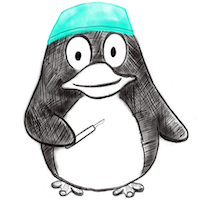
Proximity to High/Moderate vs Low Diversity Selection Food Stores and Patient Weight loss through 24 Months.
In Obesity surgery ; h5-index 57.0
PURPOSE :
MATERIALS AND METHODS :
RESULTS :
CONCLUSION :
Pratt Keeley J, Hanks Andrew S, Miller Harvey J, Swager LeeAnn C, Noria Sabrena, Brethauer Stacy, Needleman Bradley, Focht Brian C
2023-Feb-27
Bariatric surgery, Environment, Food, Walkability
Weekly Summary
Receive a weekly summary and discussion of the top papers of the week by leading researchers in the field.