Receive a weekly summary and discussion of the top papers of the week by leading researchers in the field.
An interpretable machine learning framework for measuring urban perceptions from panoramic street view images.
In iScience
Liu Yunzhe, Chen Meixu, Wang Meihui, Huang Jing, Thomas Fisher, Rahimi Kazem, Mamouei Mohammad
2023-Mar-17
Artificial Intelligence, Environmental sciences
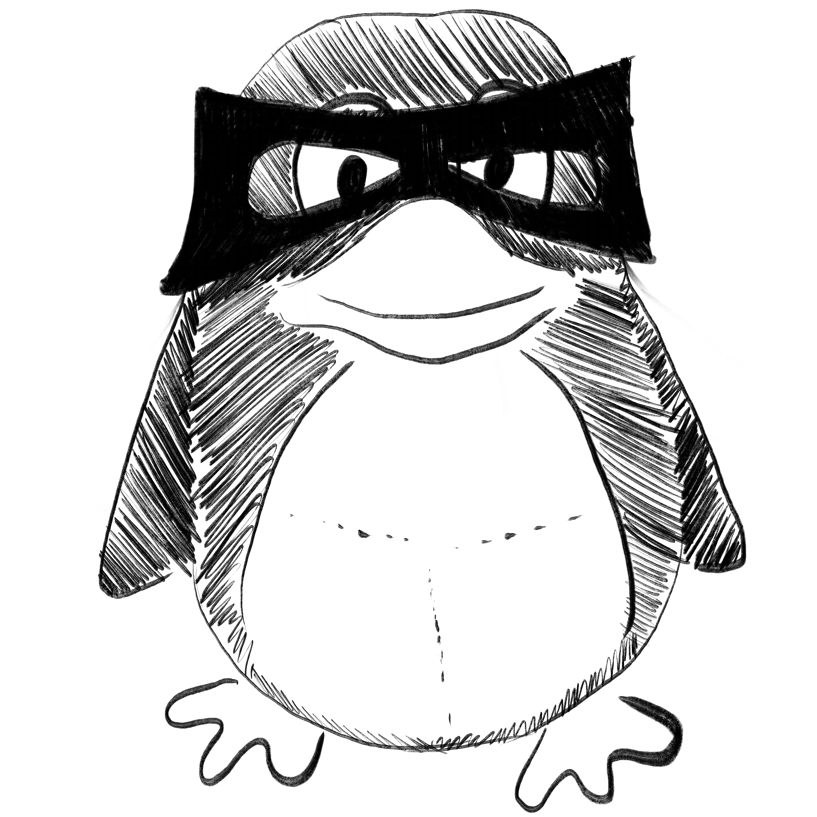
Conv-CapsNet: capsule based network for COVID-19 detection through X-Ray scans.
In Multimedia tools and applications
Sharma Pulkit, Arya Rhythm, Verma Richa, Verma Bindu
2023-Feb-21
COVID-19 detection, Capsule networks, Chest X-ray classification, Medical imaging
Noninvasive Detection of Stress by Biochemical Profiles from the Skin.
In ACS sensors
Mansour Elias, Palzur Eilam, Broza Yoav Y, Saliba Walaa, Kaisari Sharon, Goldstein Pavel, Shamir Alon, Haick Hossam
2023-Feb-27
behavioral, sensor, skin, stress, volatile organic compound
Prognostic Significance of Tumor-Infiltrating Lymphocytes Determined Using LinkNet on Colorectal Cancer Pathology Images.
In JCO precision oncology
PURPOSE :
MATERIALS AND METHODS :
RESULTS :
CONCLUSION :
Liu Anran, Li Xingyu, Wu Hongyi, Guo Bangwei, Jonnagaddala Jitendra, Zhang Hong, Xu Steven
2023-Feb
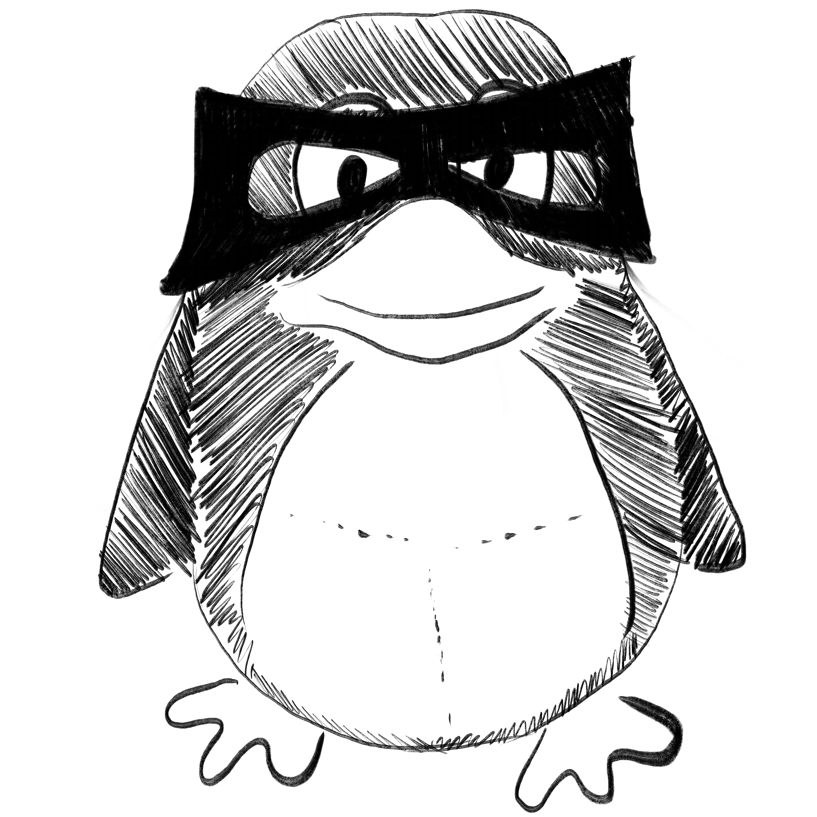
Raw Electroencephalogram-Based Cognitive Workload Classification Using Directed and Nondirected Functional Connectivity Analysis and Deep Learning.
In Big data
Gupta Anmol, Daniel Ronnie, Rao Akash, Roy Partha Pratim, Chandra Sushil, Kim Byung-Gyu
2023-Feb-27
BrainNetCNN, cognitive workload, functional connectivity analysis, mutual information, passive BCI, phase transfer entropy
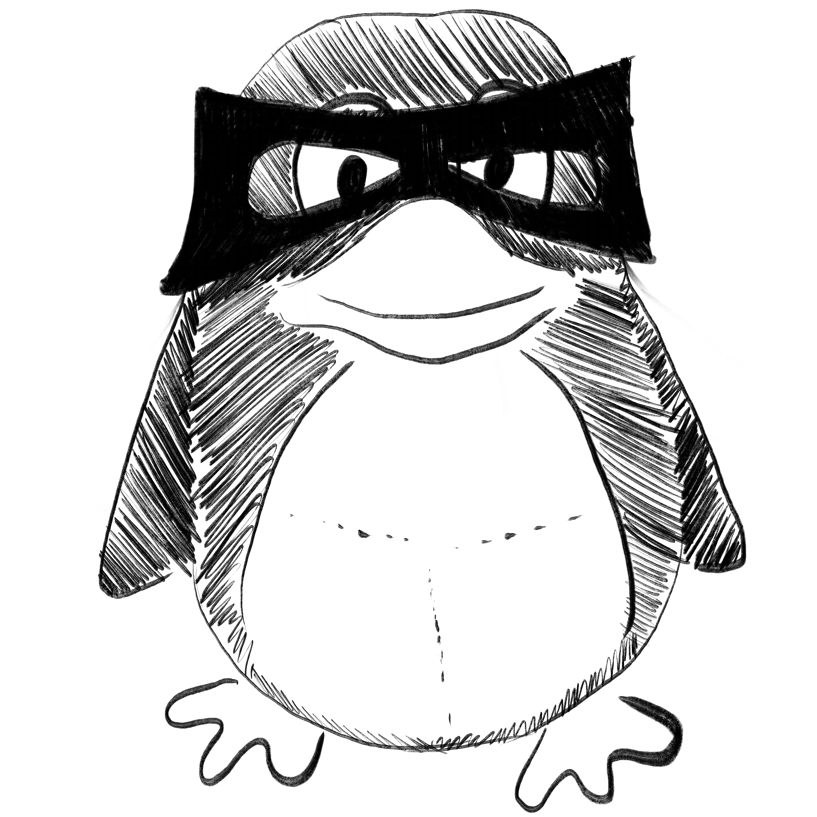
Beware of Simple Methods for Structure-Based Virtual Screening: The Critical Importance of Broader Comparisons.
In Journal of chemical information and modeling
Tran-Nguyen Viet-Khoa, Ballester Pedro J
2023-Feb-27
Weekly Summary
Receive a weekly summary and discussion of the top papers of the week by leading researchers in the field.