Receive a weekly summary and discussion of the top papers of the week by leading researchers in the field.
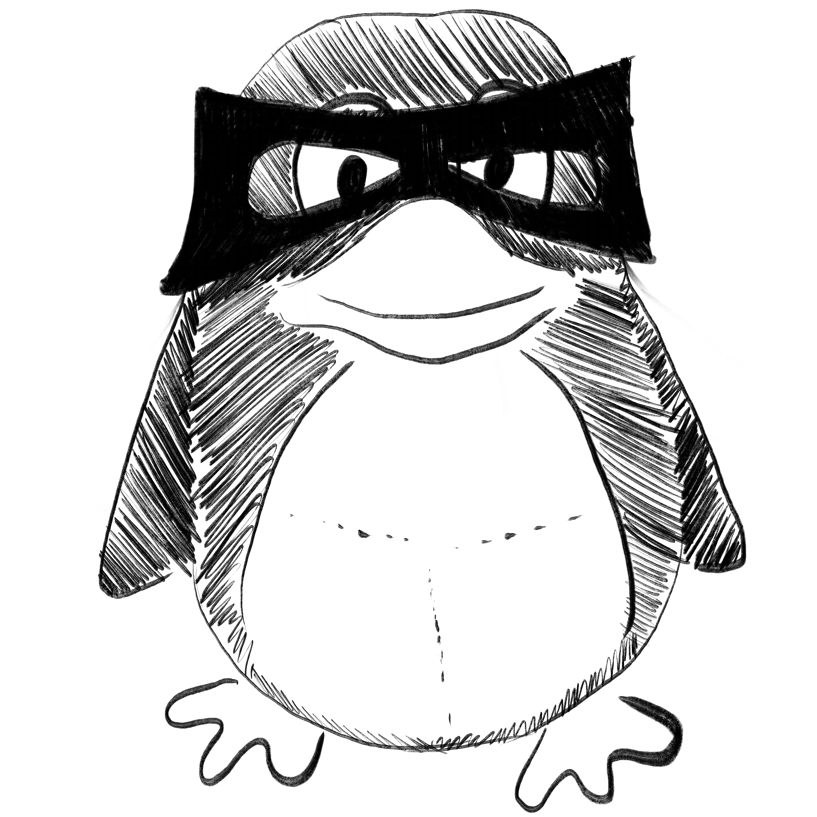
Artificial intelligence-based snakebite identification using snake images, snakebite wound images, and other modalities of information: A systematic review.
In International journal of medical informatics ; h5-index 49.0
BACKGROUND AND OBJECTIVE :
METHODS :
RESULTS :
CONCLUSIONS :
Zhang Jun, Chen Xin, Song Aiguo, Li Xin
2023-Feb-24
Active perception, Machine learning, Medical decision support system, Multi-modal fusion, Snake image, Snakebite identification
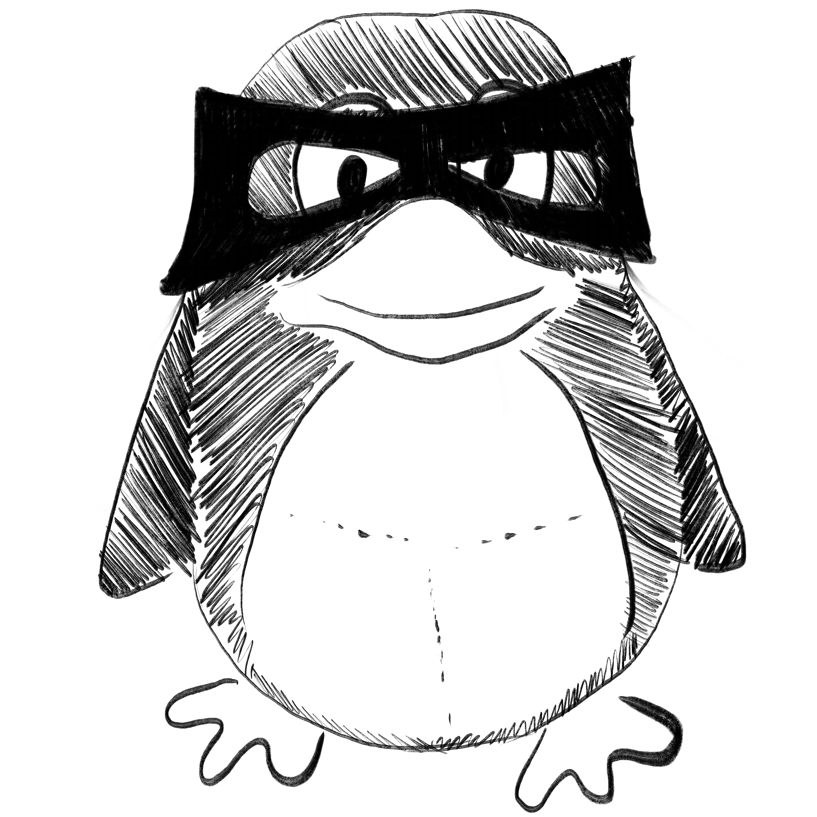
Image-based estimation of the left ventricular cavity volume using deep learning and Gaussian process with cardio-mechanical applications.
In Computerized medical imaging and graphics : the official journal of the Computerized Medical Imaging Society
Rabbani Arash, Gao Hao, Lazarus Alan, Dalton David, Ge Yuzhang, Mangion Kenneth, Berry Colin, Husmeier Dirk
2023-Feb-24
Cardiac magnetic resonance imaging, Deep learning, Gaussian process
Centerline depth world for left atrial appendage orifice localization using reinforcement learning.
In Computerized medical imaging and graphics : the official journal of the Computerized Medical Imaging Society
Al Walid Abdullah, Yun Il Dong, Chun Eun Ju
2023-Feb-24
Appendage occlusion, Centerline depth, Left atrial appendage, Orifice detection, Orifice localization, Reinforcement learning
Does water temperature influence in microcystin production? A case study of Billings Reservoir, São Paulo, Brazil.
In Journal of contaminant hydrology
Godoy Rodrigo Felipe Bedim, Trevisan Elias, Battistelli André Aguiar, Crisigiovanni Enzo Luigi, do Nascimento Elynton Alves, da Fonseca Machado Artur Lourival
2023-Feb-17
Aquatic ecosystem, Extreme events, Machine learning, Public health, Toxin
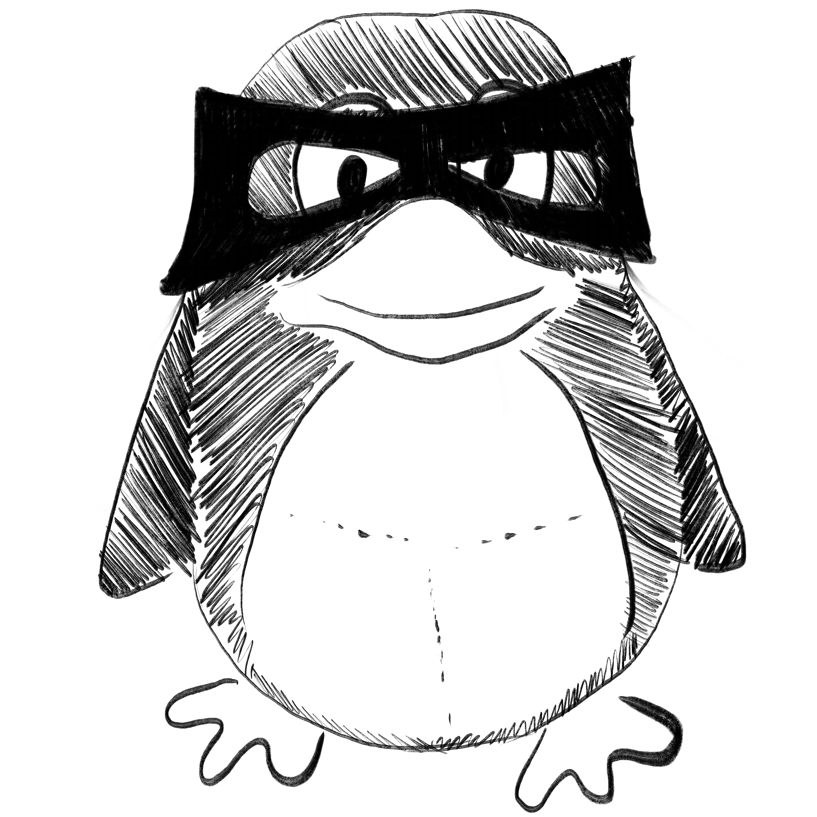
Reflections on measuring disordered thoughts as expressed via language.
In Psychiatry research ; h5-index 64.0
Elvevåg Brita
2023-Feb-06
Assessment, Language, Memory
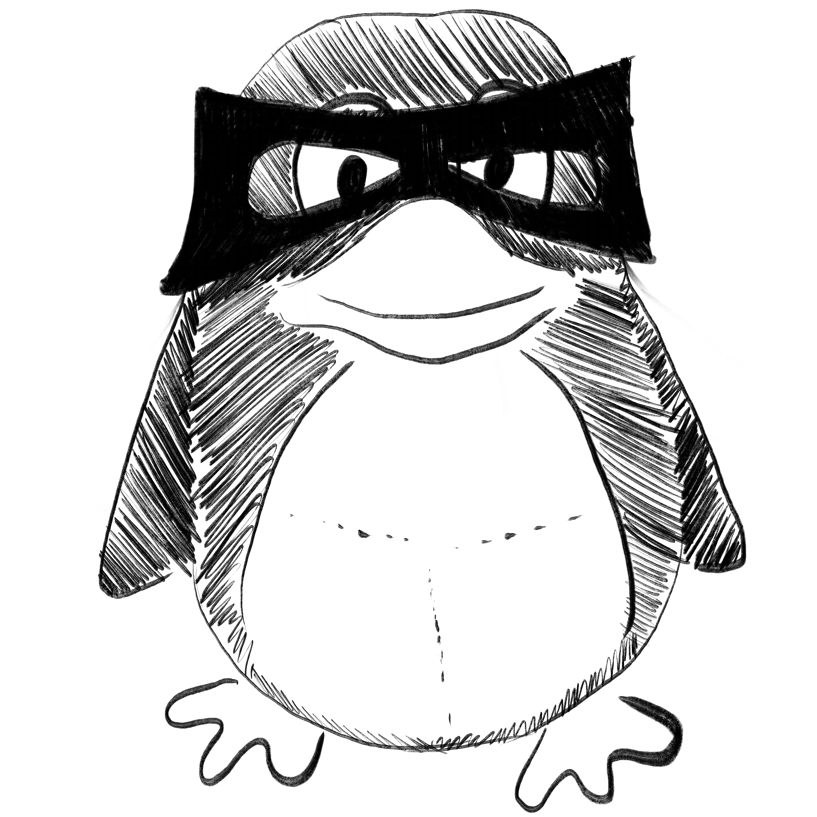
AlphaFold-multimer predicts ATG8 protein binding motifs crucial for autophagy research.
In PLoS biology
Olsvik Hallvard Lauritz, Johansen Terje
2023-Feb
Weekly Summary
Receive a weekly summary and discussion of the top papers of the week by leading researchers in the field.