Receive a weekly summary and discussion of the top papers of the week by leading researchers in the field.
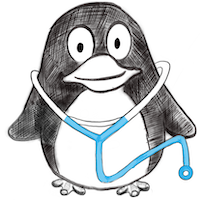
Evaluation of EfficientNet models for COVID-19 detection using lung parenchyma.
In Neural computing & applications
Kurt Zuhal, Işık Şahin, Kaya Zeynep, Anagün Yıldıray, Koca Nizameddin, Çiçek Sümeyye
2023-Feb-20
COVID-19 detection, CT scan, Deep learning, EfficientNet, K-means, Lung parenchyma
Can we predict critical care mortality with non-conventional inflammatory markers in SARS-CoV-2 infected patients?
In Clinical hemorheology and microcirculation
BACKGROUND :
OBJECTIVE :
METHODS :
RESULTS :
CONCLUSIONS :
Rozanovic Martin, Domokos Kamilla, Márovics Gergő, Rohonczi Mirtill, Csontos Csaba, Bogár Lajos, Rendeki Szilárd, Kiss Tamás, Rozanovic Melánia Nacira, Loibl Csaba
2023-Feb-18
C-reactive protein, COVID-19, infection, inflammatory response, leukocyte antisedimentation rate, neutrophil-lymphocyte ratio, procalcitonin
Molecular and functional imaging in cancer-targeted therapy: current applications and future directions.
In Signal transduction and targeted therapy
Bai Jing-Wen, Qiu Si-Qi, Zhang Guo-Jun
2023-Feb-27
A case-control study on predicting population risk of suicide using health administrative data: a research protocol.
In BMJ open
INTRODUCTION :
METHODS AND ANALYSIS :
ETHICS AND DISSEMINATION :
Wang JianLi, Gholi Zadeh Kharrat Fatemeh, Pelletier Jean-François, Rochette Louis, Pelletier Eric, Lévesque Pascale, Massamba Victoria, Brousseau-Paradis Camille, Mohammed Mada, Gariépy Geneviève, Gagné Christian, Lesage Alain
2023-Feb-27
HEALTH SERVICES ADMINISTRATION & MANAGEMENT, PSYCHIATRY, PUBLIC HEALTH, Suicide & self-harm
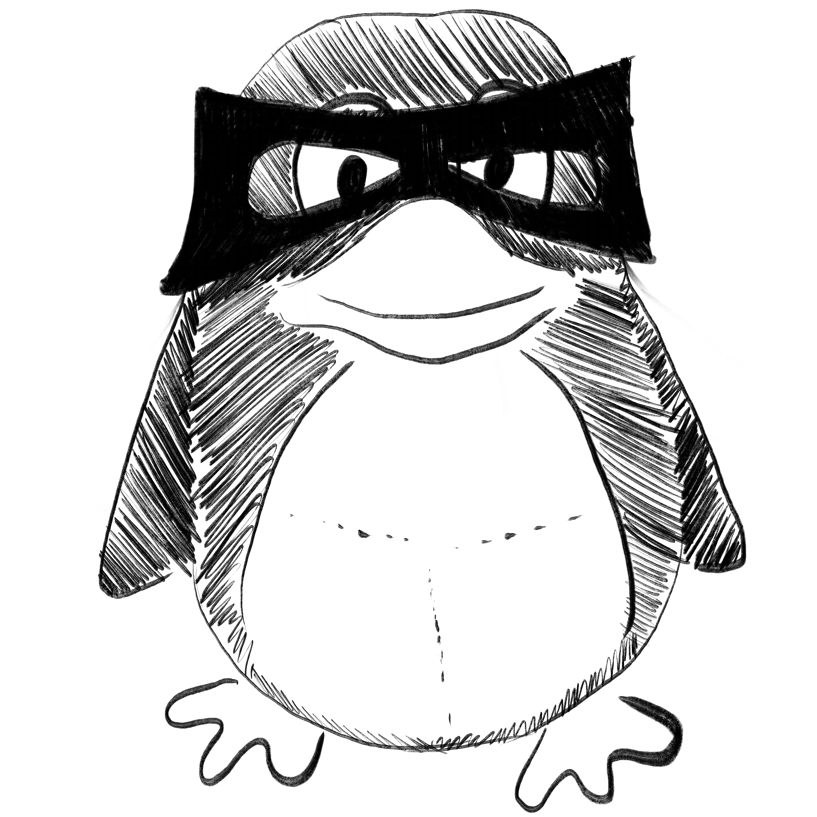
Complex hierarchical structures in single-cell genomics data unveiled by deep hyperbolic manifold learning.
In Genome research ; h5-index 99.0
Tian Tian, Zhong Cheng, Lin Xiang, Wei Zhi, Hakonarson Hakon
2023-Feb-27
Image Quality Assessment of Deep Learning Image Reconstruction in Torso Computed Tomography Using Tube Current Modulation.
In Acta medica Okayama
Takeuchi Kazuhiro, Ide Yasuhiro, Mori Yuichiro, Uehara Yusuke, Sukeishi Hiroshi, Goto Sachiko
2023-Feb
computed tomography, deep learning, image reconstruction, object size, tube current modulation
Weekly Summary
Receive a weekly summary and discussion of the top papers of the week by leading researchers in the field.