Receive a weekly summary and discussion of the top papers of the week by leading researchers in the field.
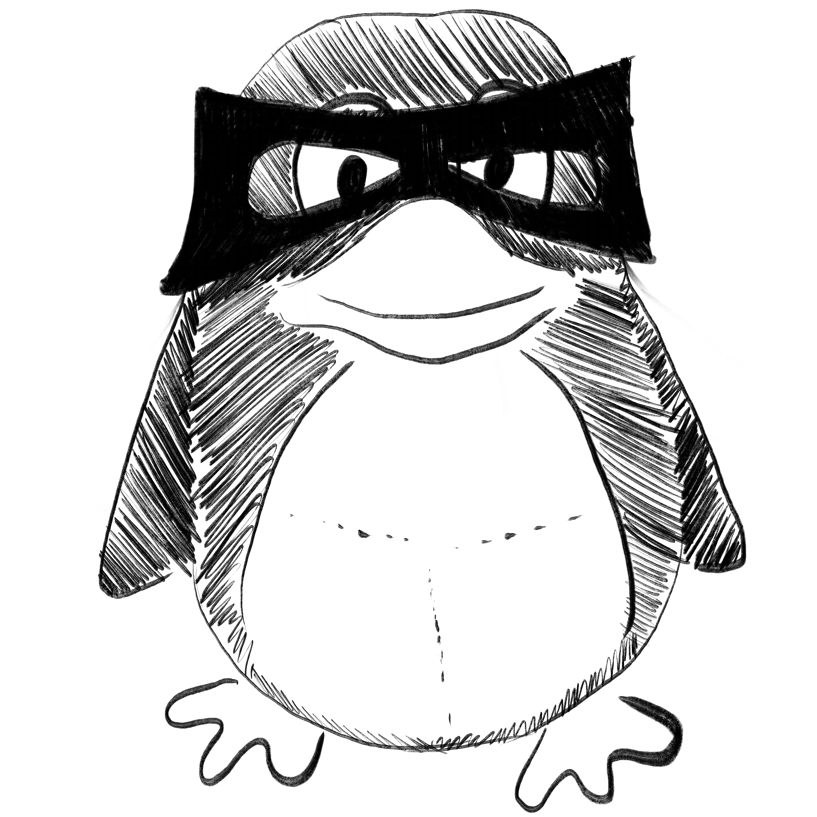
Development of evaluation system for cerebral artery occlusion in emergency medical services: noninvasive measurement and utilization of pulse waves.
In Scientific reports ; h5-index 158.0
Shimada Takuma, Matsubara Kazumasa, Koyama Daisuke, Matsukawa Mami, Ohsaki Miho, Kobayashi Yasuyo, Saito Kozue, Yamagami Hiroshi
2023-Feb-27
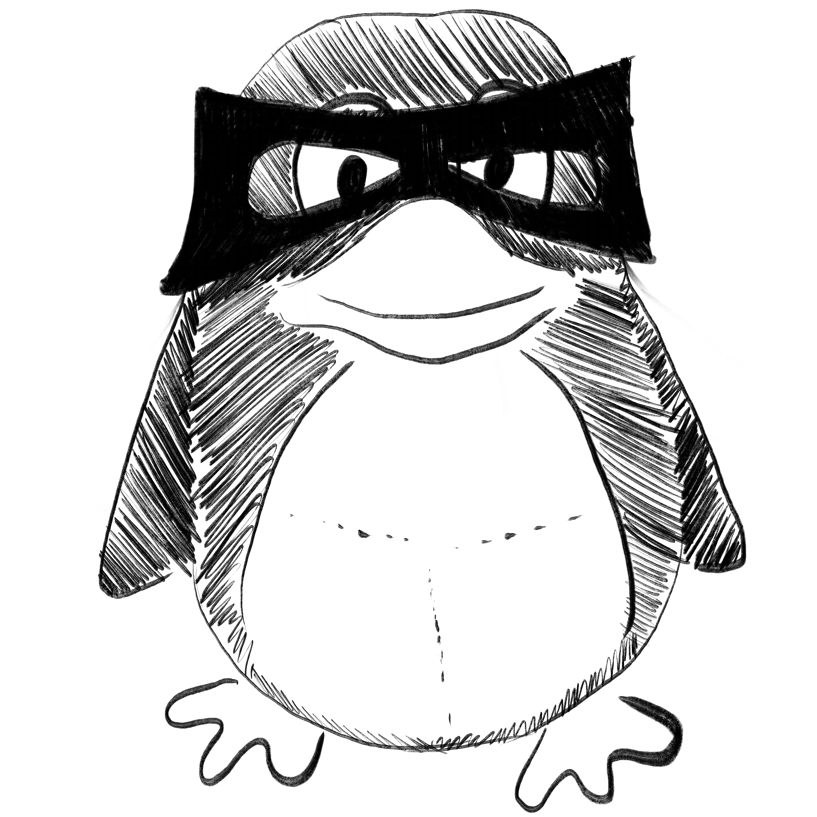
Pet dog facial expression recognition based on convolutional neural network and improved whale optimization algorithm.
In Scientific reports ; h5-index 158.0
Mao Yan, Liu Yaqian
2023-Feb-27
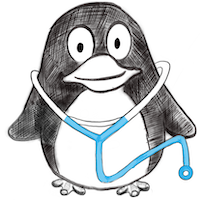
Current situation of telemedicine research for cardiovascular risk in Japan.
In Hypertension research : official journal of the Japanese Society of Hypertension
Shibata Shigeru, Hoshide Satoshi
2023-Feb-27
Digital hypertension, High blood pressure, ICT, Online medical counseling, Telehealth
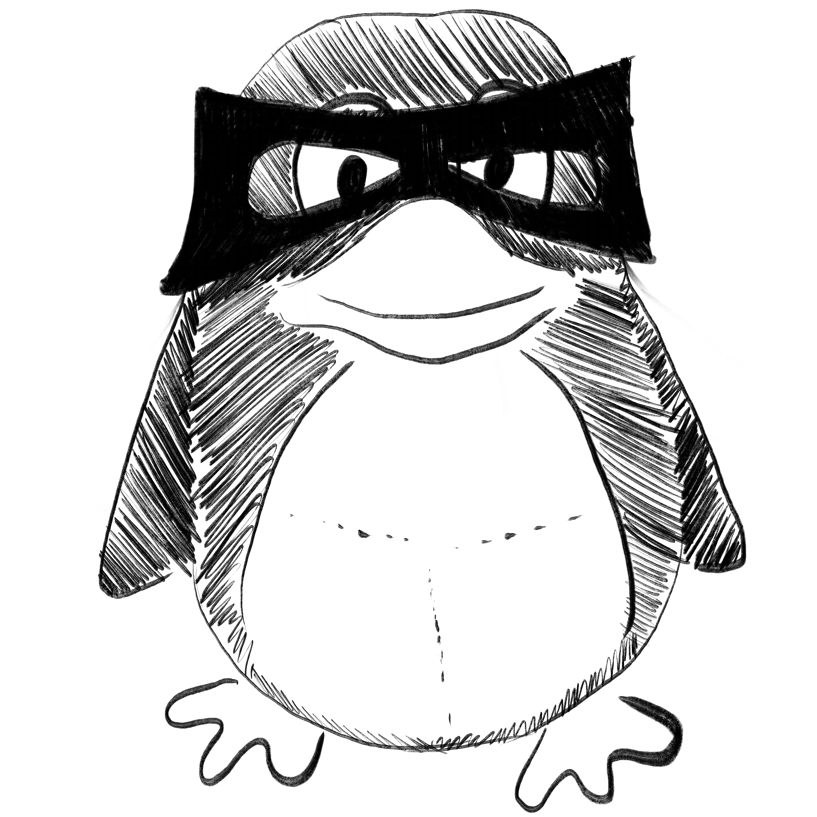
Using machine learning with optical profilometry for GaN wafer screening.
In Scientific reports ; h5-index 158.0
Gallagher James C, Mastro Michael A, Ebrish Mona A, Jacobs Alan G, Gunning Brendan P, Kaplar Robert J, Hobart Karl D, Anderson Travis J
2023-Feb-27
Deep learning augmented ECG analysis to identify biomarker-defined myocardial injury.
In Scientific reports ; h5-index 158.0
Chaudhari Gunvant R, Mayfield Jacob J, Barrios Joshua P, Abreau Sean, Avram Robert, Olgin Jeffrey E, Tison Geoffrey H
2023-Feb-27
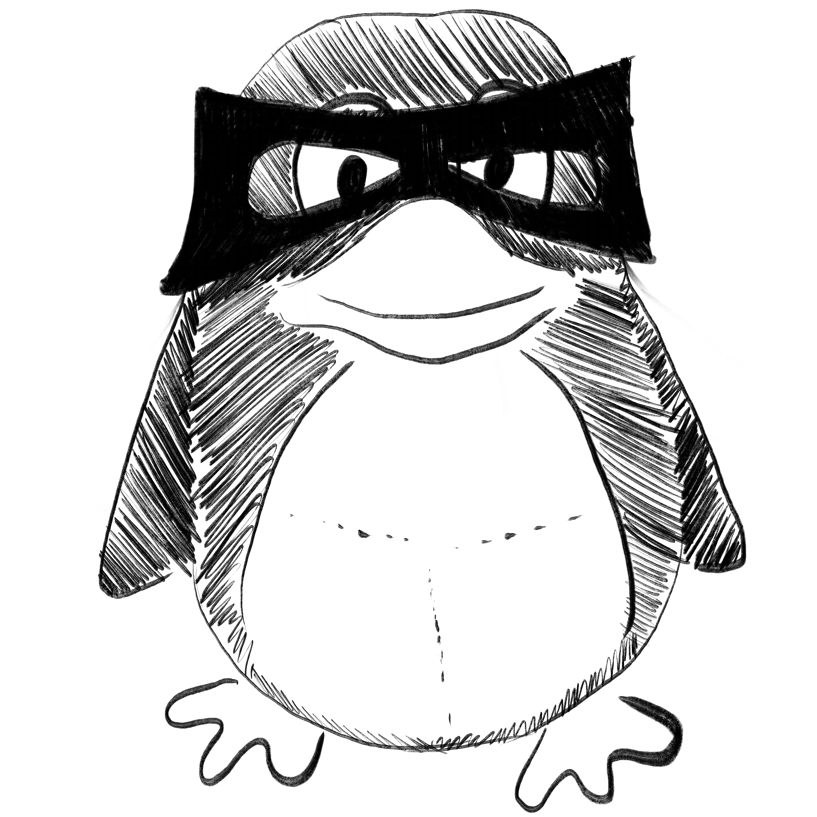
SBAS-InSAR based validated landslide susceptibility mapping along the Karakoram Highway: a case study of Gilgit-Baltistan, Pakistan.
In Scientific reports ; h5-index 158.0
Kulsoom Isma, Hua Weihua, Hussain Sadaqat, Chen Qihao, Khan Garee, Shihao Dai
2023-Feb-27
Weekly Summary
Receive a weekly summary and discussion of the top papers of the week by leading researchers in the field.