Receive a weekly summary and discussion of the top papers of the week by leading researchers in the field.
Detecting Tweets Containing Cannabidiol-Related COVID-19 Misinformation Using Transformer Language Models and Warning Letters From Food and Drug Administration: Content Analysis and Identification.
In JMIR infodemiology
BACKGROUND :
OBJECTIVE :
METHODS :
RESULTS :
CONCLUSIONS :
Turner Jason, Kantardzic Mehmed, Vickers-Smith Rachel, Brown Andrew G
2023
COVID-19, Twitter, cannabidiol, content analysis, deep learning, health information, infodemic, infodemiology, language model, misinformation, pandemic, sentence vector, social media, transformer
Impact of human mobility on COVID-19 transmission according to mobility distance, locations and demographic factors in the Greater Bay area of China:a population-based study.
In JMIR public health and surveillance
BACKGROUND :
OBJECTIVE :
METHODS :
RESULTS :
CONCLUSIONS :
Xia Jizhe, Yin Kun, Yue Yang, Li Qingquan, Wang Xiling, Hu Dongsheng, Wang Xiong, Du Zhanwei, Cowling Ben J, Chen Erzhen, Zhou Ying
2023-Feb-23
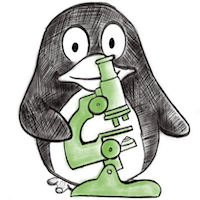
3D bioprinting and the revolution in experimental cancer model systems-A review of developing new models and experiences with in vitro 3D bioprinted breast cancer tissue-mimetic structures.
In Pathology oncology research : POR
Sztankovics Dániel, Moldvai Dorottya, Petővári Gábor, Gelencsér Rebeka, Krencz Ildikó, Raffay Regina, Dankó Titanilla, Sebestyén Anna
2023
3D bioprinting, biofabrication, breast cancer, cancer, disease models
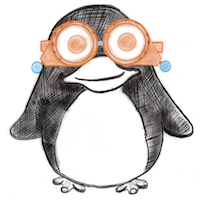
Glaucoma and Telemedicine.
In Journal of glaucoma
Brandão-de-Resende Camilo, de Alcântara Liliane de Abreu Rosa, Vasconcelos-Santos Daniel Vítor, Diniz-Filho Alberto
2023-Feb-28
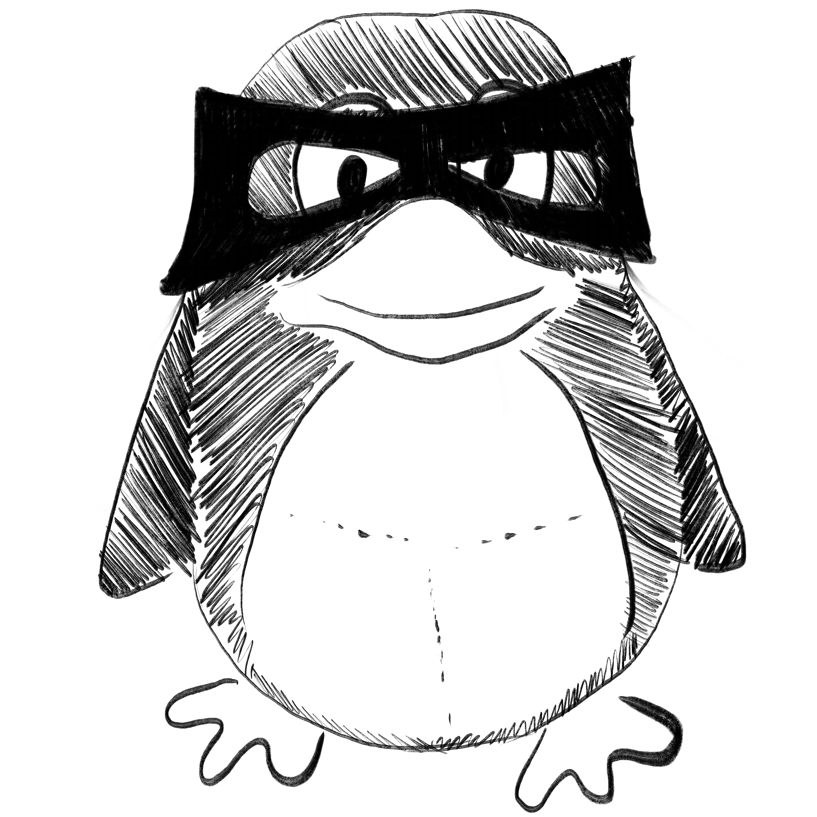
Neural text generation in regulatory medical writing.
In Frontiers in pharmacology
Meyer Claudia, Adkins Daniel, Pal Koyena, Galici Ruggero, Garcia-Agundez Augusto, Eickhoff Carsten
2023
abstractive summarization, artificial intelligence-AI, drug labeling, medication guide, natural language generation, pointer generator network
Artificial Intelligence Functionalities During the COVID-19 Pandemic.
In Disaster medicine and public health preparedness
BACKGROUND :
METHODS :
RESULTS :
CONCLUSIONS :
Ahmadi Marzaleh Milad, Peyravi Mahmoudreza, Mousavi Shahrokh, Sarpourian Fatemeh, Seyedi Milad, Shalyari Naseh
2023-Feb-27
COVID-19, artificial intelligence, deep learning, machine learning, neural networks
Weekly Summary
Receive a weekly summary and discussion of the top papers of the week by leading researchers in the field.