Receive a weekly summary and discussion of the top papers of the week by leading researchers in the field.
DNA methylation-based classification of glioneuronal tumours synergises with histology and radiology to refine accurate molecular stratification.
In Neuropathology and applied neurobiology ; h5-index 39.0
AIMS :
METHODS :
RESULTS :
CONCLUSIONS :
Stone Thomas J, Mankad Kshitij, Tan A I Peng, Jan Wajanat, Pickles Jessica C, Gogou Maria, Chalker Jane, Slodkowska Iwona, Pang Emily, Kristiansen Mark, Madhan Gaganjit K, Forrest Leysa, Hughes Deborah, Koutroumanidou Eleni, Mistry Talisa, Ogunbiyi Olumide, Ahmed Saira W, Cross J Helen, Hubank Mike, Hargrave Darren, Jacques Thomas S
2023-Feb-26
dysembryoplastic neuroepithelial tumour, ganglioglioma, glioneuronal tumour, machine learning, molecular pathology
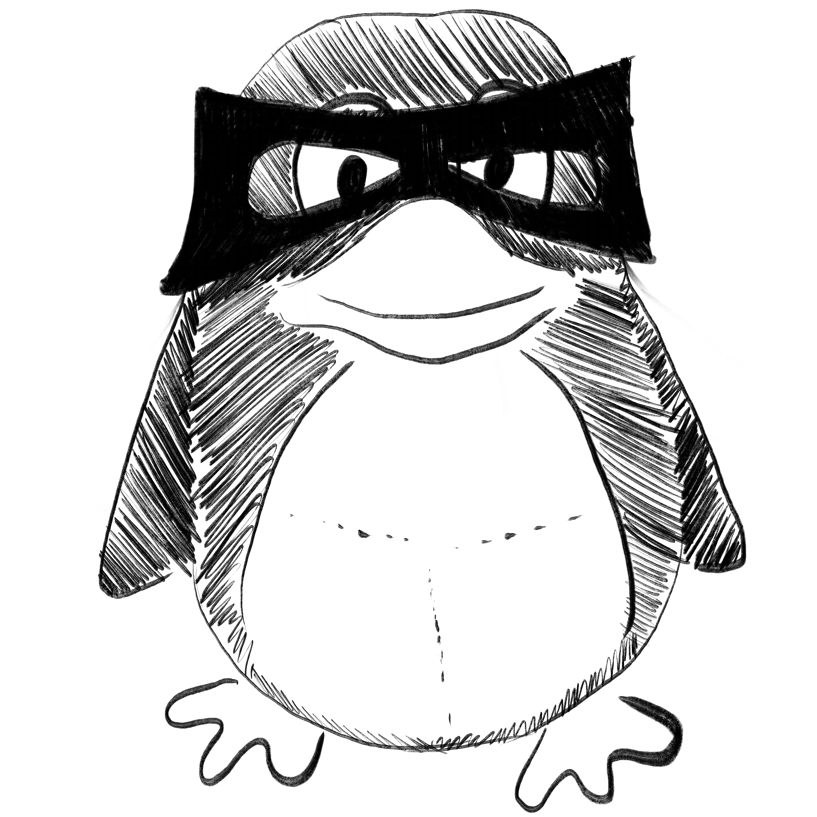
Detection of COVID-19 Case from Chest CT Images Using Deformable Deep Convolutional Neural Network.
In Journal of healthcare engineering
Foysal Md, Hossain A B M Aowlad, Yassine Abdulsalam, Hossain M Shamim
2023
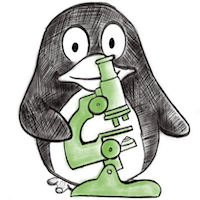
Kartezio: Evolutionary Design of Explainable Pipelines for Biomedical Image Analysis
ArXiv Preprint
Kévin Cortacero, Brienne McKenzie, Sabina Müller, Roxana Khazen, Fanny Lafouresse, Gaëlle Corsaut, Nathalie Van Acker, François-Xavier Frenois, Laurence Lamant, Nicolas Meyer, Béatrice Vergier, Dennis G. Wilson, Hervé Luga, Oskar Staufer, Michael L. Dustin, Salvatore Valitutti, Sylvain Cussat-Blanc
2023-02-28
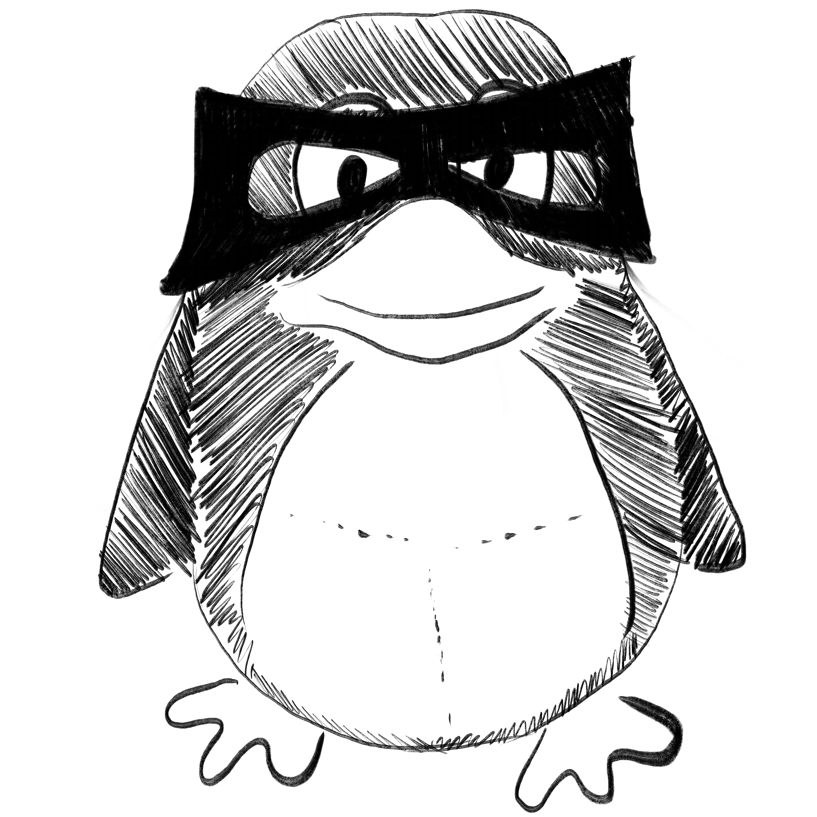
Digitally embodied lifespan neurocognitive development and Tactile Internet: Transdisciplinary challenges and opportunities.
In Frontiers in human neuroscience ; h5-index 79.0
Li Shu-Chen, Fitzek Frank H P
2023
Tactile Internet, aging, development, multisensory, neuromodulation, perception, sensory augmentation, signal-to-noise
Resistance-resistant antibacterial treatment strategies.
In Frontiers in antibiotics
Batchelder Jonathan I, Hare Patricia J, Mok Wendy W K
2023
antibiotic resistance, combination therapies, evolutionary steering, mutation, stress response
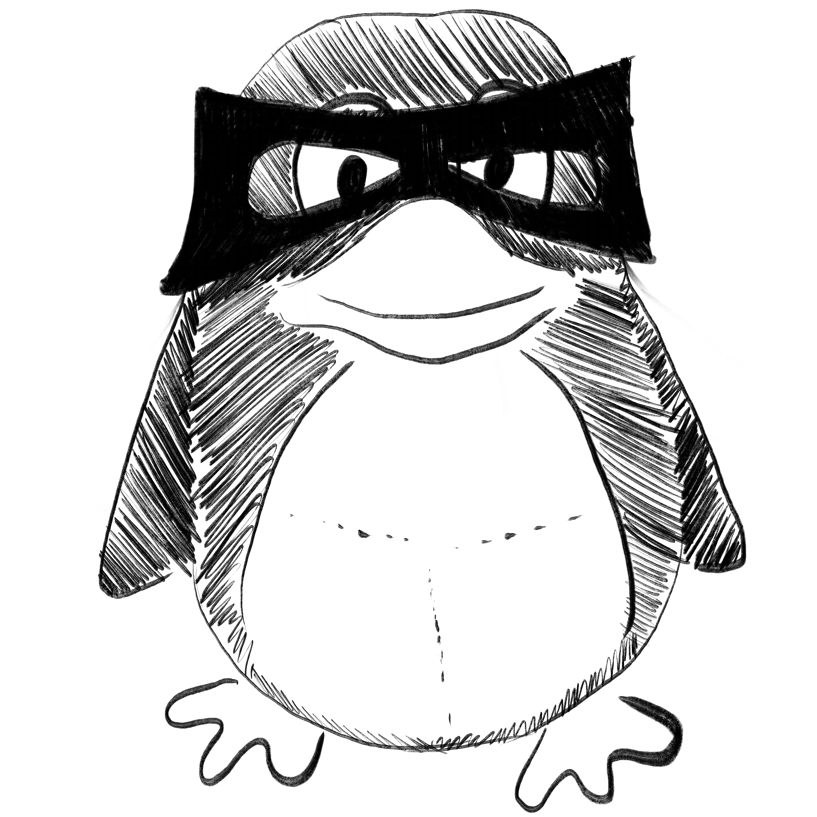
Machine learning aided jump height estimate democratization through smartphone measures.
In Frontiers in sports and active living
INTRODUCTION :
METHODS :
RESULTS :
DISCUSSION :
Mascia Guido, De Lazzari Beatrice, Camomilla Valentina
2023
accelerometer, gyroscope, modal analysis, neural network, strength and conditioning
Weekly Summary
Receive a weekly summary and discussion of the top papers of the week by leading researchers in the field.