Receive a weekly summary and discussion of the top papers of the week by leading researchers in the field.
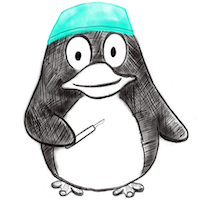
Machine Learning and Artificial Intelligence in Surgical Research.
In The Surgical clinics of North America
Srinivas Shruthi, Young Andrew J
2023-Apr
Artificial intelligence, Machine learning, Surgical research
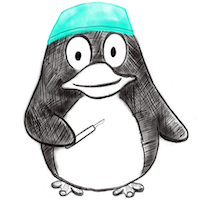
Generation Learning Differences in Surgery: Why They Exist, Implication, and Future Directions.
In The Surgical clinics of North America
Weykamp Mike, Bingham Jason
2023-Apr
Artificial intelligence, Asynchronous learning, Connectivism, Generational learning, Learning disparities, Learning theory, Machine learning, Medical education
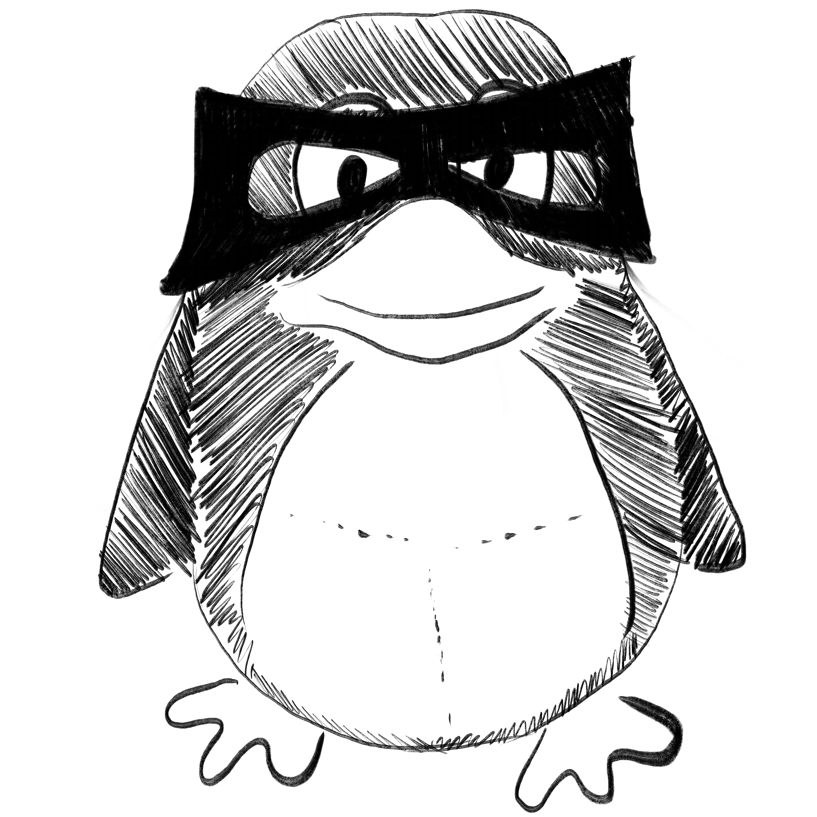
Dynamic 3D imaging of cerebral blood flow in awake mice using self-supervised-learning-enhanced optical coherence Doppler tomography.
In Communications biology
Pan Yingtian, Park Kicheon, Ren Jiaxiang, Volkow Nora D, Ling Haibin, Koretsky Alan P, Du Congwu
2023-Mar-21
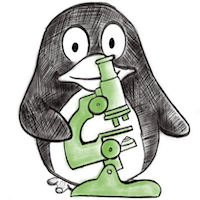
Medical diffusion on a budget: textual inversion for medical image generation
ArXiv Preprint
Bram de Wilde, Anindo Saha, Richard P. G. ten Broek, Henkjan Huisman
2023-03-23
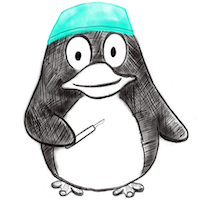
Big Data in Surgery.
In The Surgical clinics of North America
Prien Christopher, Lincango Eddy P, Holubar Stefan D
2023-Apr
Artificial intelligence, Big data, Machine learning, Natural language processing, Quality, Registries, Surgery, outcomes research
Radiologist's Disease: Imaging for Renal Cancer.
In The Urologic clinics of North America
Chung Alex, Raman Steven S
2023-May
CT, MRI, Multiphasic contrast imaging, Ultrasound
Weekly Summary
Receive a weekly summary and discussion of the top papers of the week by leading researchers in the field.