Receive a weekly summary and discussion of the top papers of the week by leading researchers in the field.
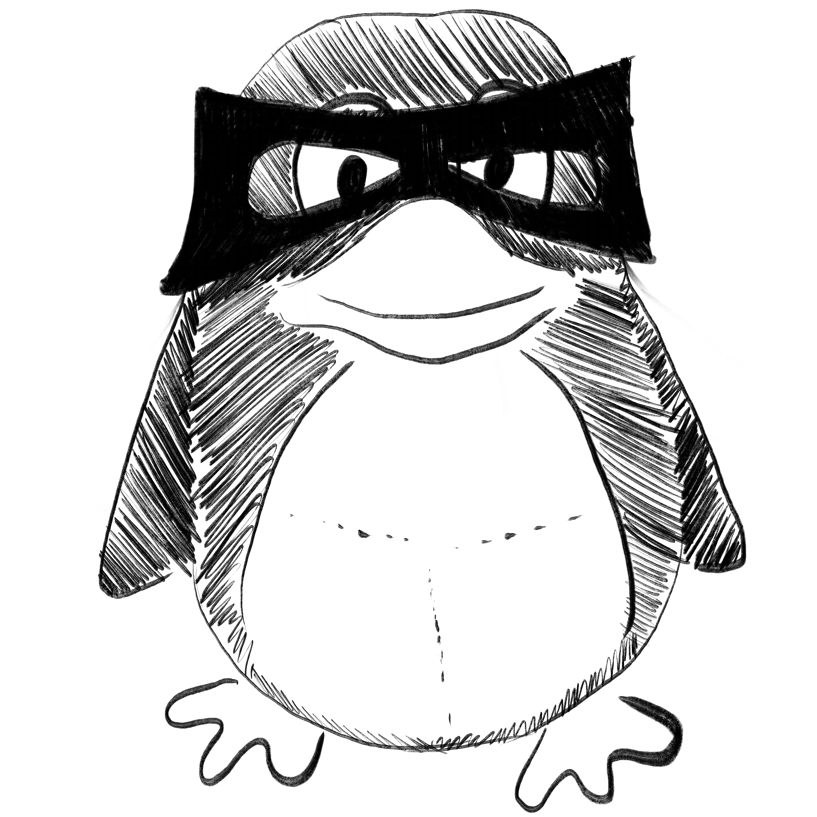
[Construction of an epileptic seizure prediction model using a semi-supervised method of generative adversarial and long short term memory network combined with Stockwell transform].
In Nan fang yi ke da xue xue bao = Journal of Southern Medical University
OBJECTIVE :
METHODS :
RESULTS :
CONCLUSION :
Liao J, Li H, Zhan C, Yang F
2023-Jan-20
Stockwell transform, bi-directional long short term memory network, epileptic seizure prediction, generative adversarial network, scalp EEG
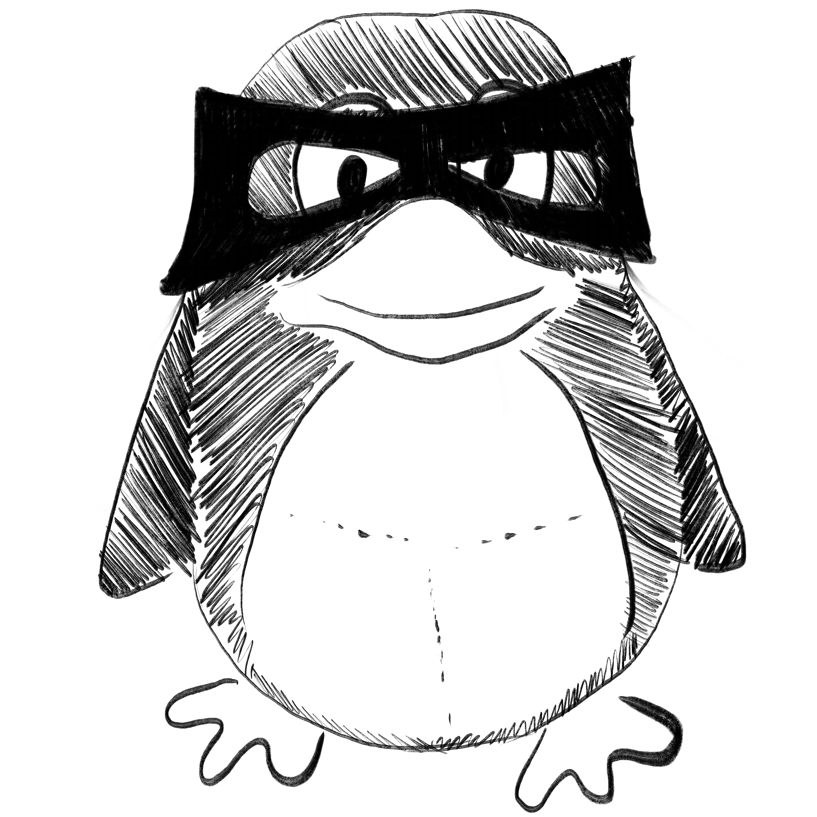
Prediction of dose deposition matrix using Voxel features driven machine learning approach.
In The British journal of radiology
OBJECTIVES :
METHODS :
RESULTS :
CONCLUSIONS :
ADVANCES IN KNOWLEDGE :
Jiao Shengxiu, Zhao Xiaoqian, Yao Shuzhan
2023-Mar-01
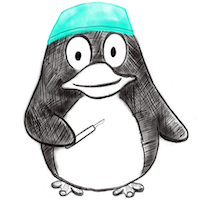
The Utility of Machine Learning for Predicting Donor Discard in Abdominal Transplantation.
In Clinical transplantation ; h5-index 26.0
BACKGROUND :
METHODS :
RESULTS :
CONCLUSIONS :
Pettit Rowland W, Marlatt Britton B, Miles Travis J, Uzgoren Selim, Corr Stuart J, Shetty Anil, Havelka Jim, Rana Abbas
2023-Mar-01
XGBoost, discard, machine learning, outcomes, prediction, random forest, transplantation
Application of artificial intelligence in the diagnosis of sleep apnea.
In Journal of clinical sleep medicine : JCSM : official publication of the American Academy of Sleep Medicine
STUDY OBJECTIVES :
METHODS :
RESULTS :
CONCLUSIONS :
Bazoukis George, Bollepalli Sandeep Chandra, Chung Cheuk To, Li Xinmu, Tse Gary, Bartley Bethany L, Batool-Anwar Salma, Quan Stuart F, Armoundas Antonis A
2023-Mar-01
artificial intelligence, machine learning, sleep apnea
Myocardial Metabolomics of Human Heart Failure With Preserved Ejection Fraction.
In Circulation ; h5-index 165.0
BACKGROUND :
METHODS :
RESULTS :
CONCLUSIONS :
Hahn Virginia S, Petucci Christopher, Kim Min-Soo, Bedi Kenneth C, Wang Hanghang, Mishra Sumita, Koleini Navid, Yoo Edwin J, Margulies Kenneth B, Arany Zoltan, Kelly Daniel P, Kass David A, Sharma Kavita
2023-Mar-01
HFpEF, branched chain amino acid, fatty acid oxidation, human, metabolism, metabolomics
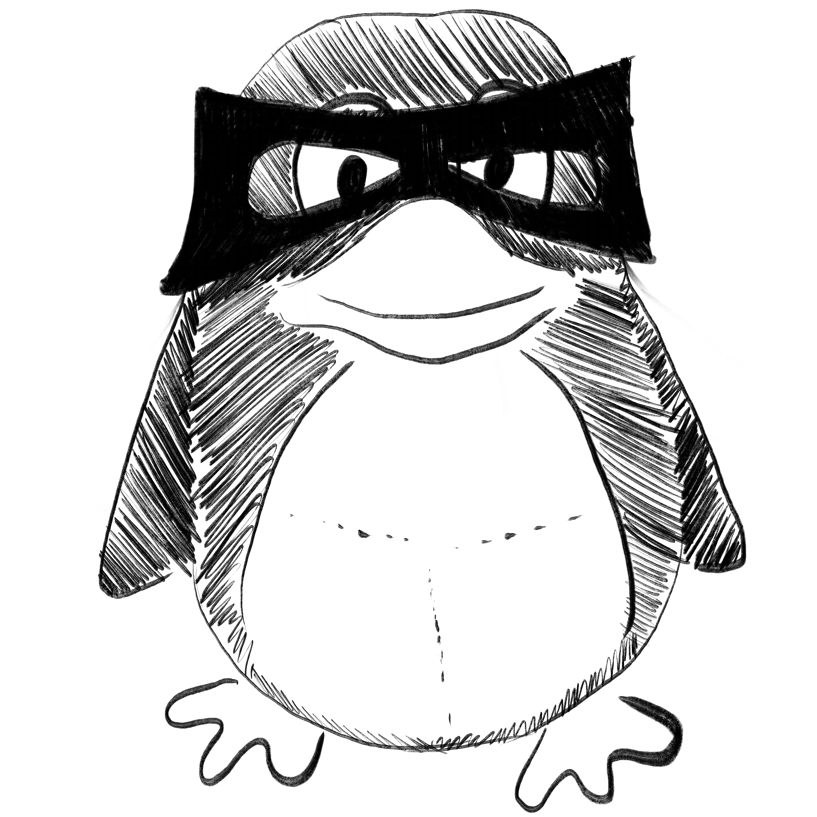
Elabela-apelin-12, 17, 36/APJ system promotes platelet aggregation and thrombosis via activating the PANX1-P2X7 signaling pathway.
In Journal of cellular biochemistry
Chen Zhe, Luo Xuling, Liu Meiqing, Jiang Jinyong, Li Yao, Huang Zhen, Wang Lingzhi, Cao Jiangang, He Lu, Huang Shifang, Hu Haoliang, Li Lanfang, Chen Linxi
2023-Mar-01
Schisandrin A, cobicistat, elabela-apelin/APJ system, pannexin1, thrombosis
Weekly Summary
Receive a weekly summary and discussion of the top papers of the week by leading researchers in the field.