Receive a weekly summary and discussion of the top papers of the week by leading researchers in the field.
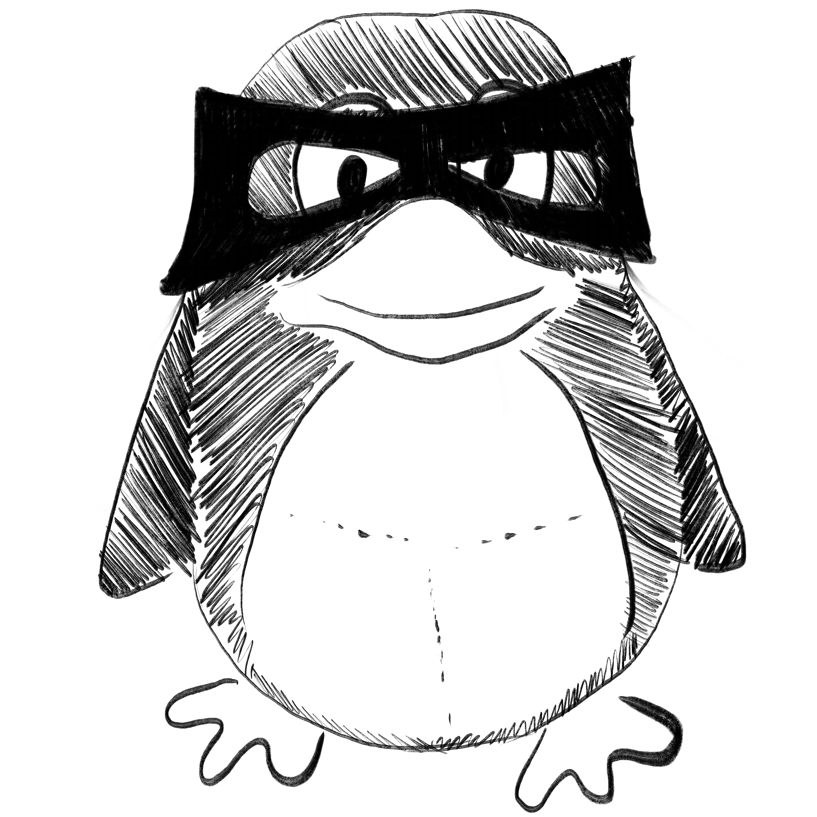
Multi-algorithms analysis for pre-treatment prediction of response to transarterial chemoembolization in hepatocellular carcinoma on multiphase MRI.
In Insights into imaging
OBJECTIVES :
METHODS :
RESULTS :
CONCLUSIONS :
Chen Mingzhen, Kong Chunli, Qiao Enqi, Chen Yaning, Chen Weiyue, Jiang Xiaole, Fang Shiji, Zhang Dengke, Chen Minjiang, Chen Weiqian, Ji Jiansong
2023-Feb-28
Deep learning, Hepatocellular carcinoma, Radiomics, Transarterial chemoembolization
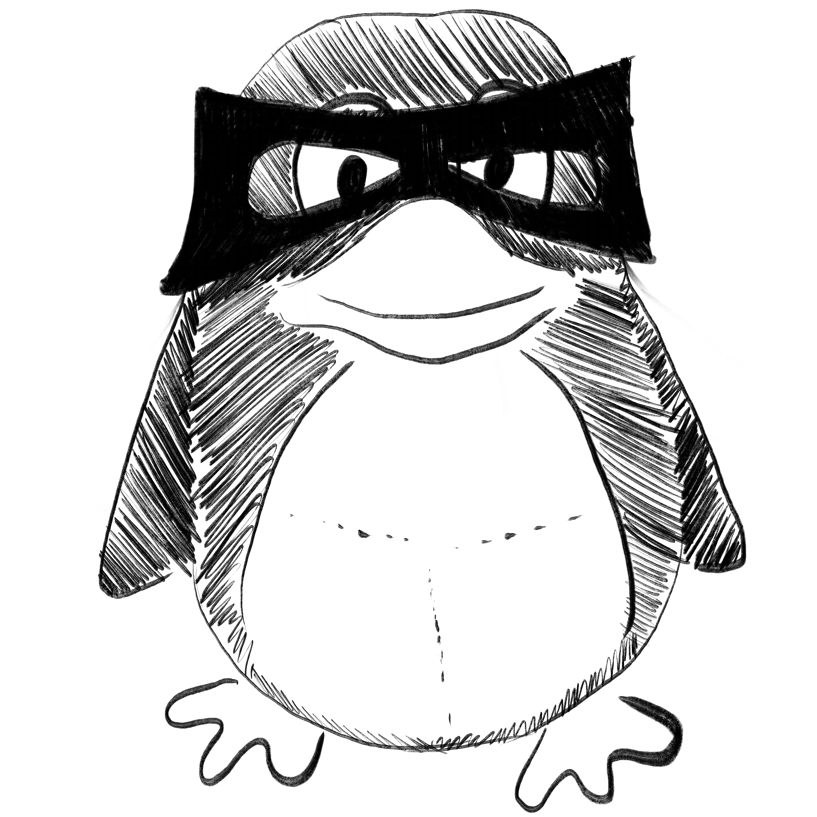
TransCode: Uncovering COVID-19 transmission patterns via deep learning.
In Infectious diseases of poverty ; h5-index 31.0
BACKGROUND :
METHODS :
RESULTS :
CONCLUSIONS :
Ren Jinfu, Liu Mutong, Liu Yang, Liu Jiming
2023-Feb-28
COVID-19, Deep transfer learning, Densely populated regions, Human mobility and contact behaviors, Meta-population, Spatiotemporal transmission dynamics and heterogeneity, TransCode
Transferring Models Trained on Natural Images to 3D MRI via Position Encoded Slice Models
ArXiv Preprint
Umang Gupta, Tamoghna Chattopadhyay, Nikhil Dhinagar, Paul M. Thompson, Greg Ver Steeg, The Alzheimer’s Disease Neuroimaging Initiative
2023-03-02
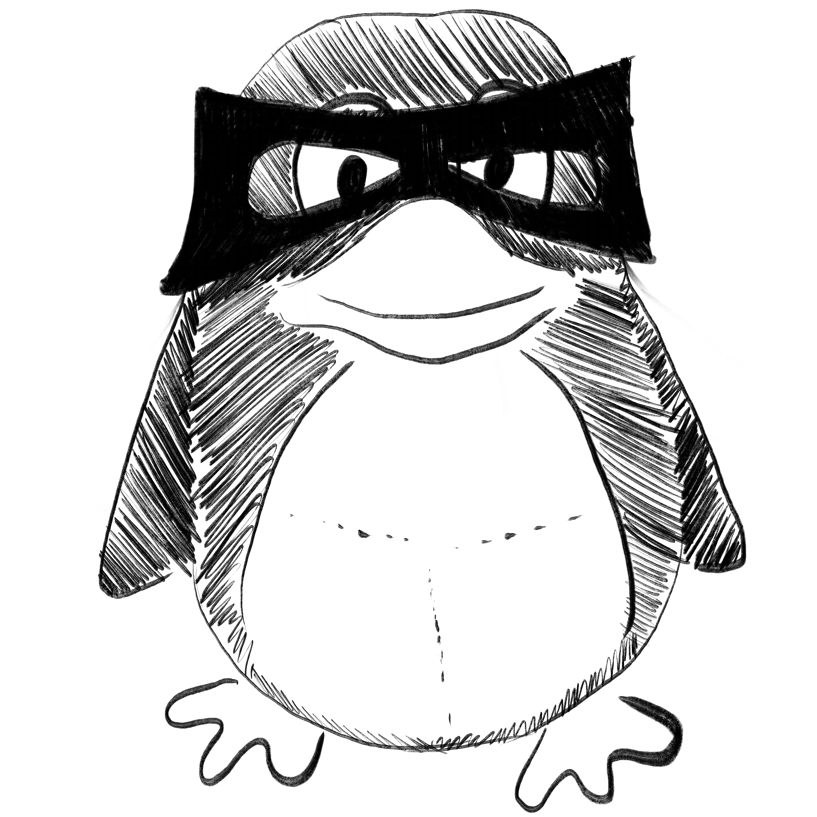
Semiclassical Dynamics on Machine-Learned Coupled Multireference Potential Energy Surfaces: Application to the Photodissociation of the Simplest Criegee Intermediate.
In The journal of physical chemistry. A
Sit Mahesh K, Das Subhasish, Samanta Kousik
2023-Mar-01
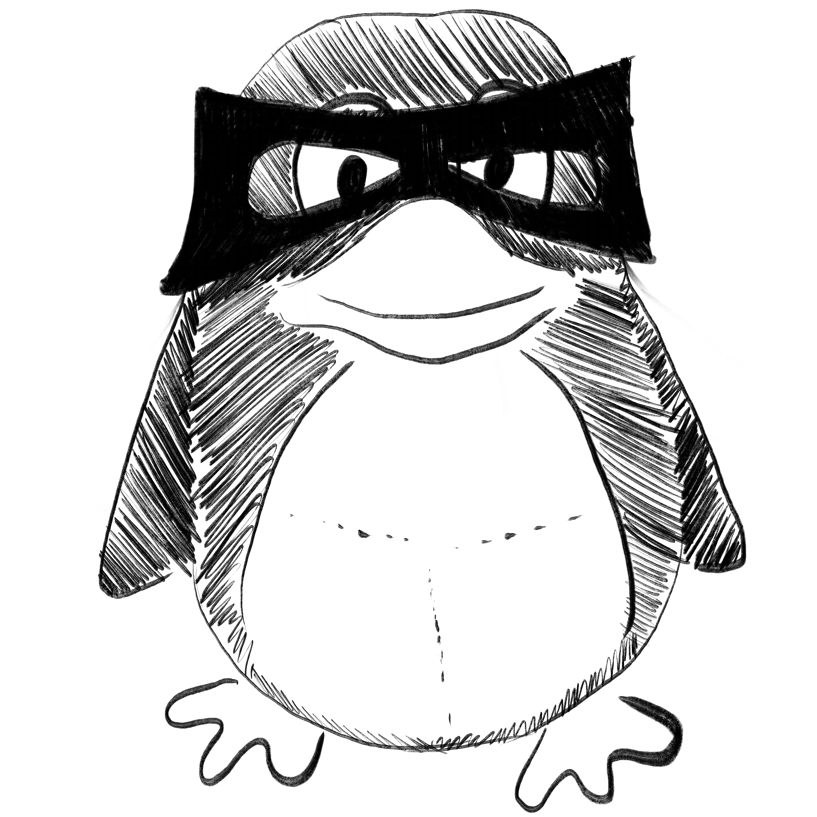
NLP systems such as ChatGPT cannot be listed as an author because these cannot fulfill widely adopted authorship criteria.
In Accountability in research
Yeo-Teh Nicole Shu Ling, Tang Bor Luen
2023-Mar-01
Aigiarism, accountability, authorship, generative AI, natural language processing (NLP) models
[Comparison of prediction ability of two extended Cox models in nonlinear survival data analysis].
In Nan fang yi ke da xue xue bao = Journal of Southern Medical University
OBJECTIVE :
METHODS :
RESULTS :
CONCLUSION :
Chen Y, Wei H, Pan J, An S
2023-Jan-20
Cox model, deep neural network, nonlinear correlation, restricted cubic spline, survival analysis
Weekly Summary
Receive a weekly summary and discussion of the top papers of the week by leading researchers in the field.