Receive a weekly summary and discussion of the top papers of the week by leading researchers in the field.
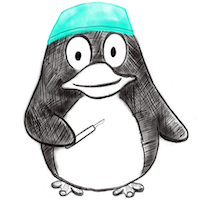
Machine learning-based prediction of intraoperative hypoxemia for pediatric patients.
In PloS one ; h5-index 176.0
BACKGROUND :
METHODS :
RESULTS :
CONCLUSIONS :
Park Jung-Bin, Lee Ho-Jong, Yang Hyun-Lim, Kim Eun-Hee, Lee Hyung-Chul, Jung Chul-Woo, Kim Hee-Soo
2023
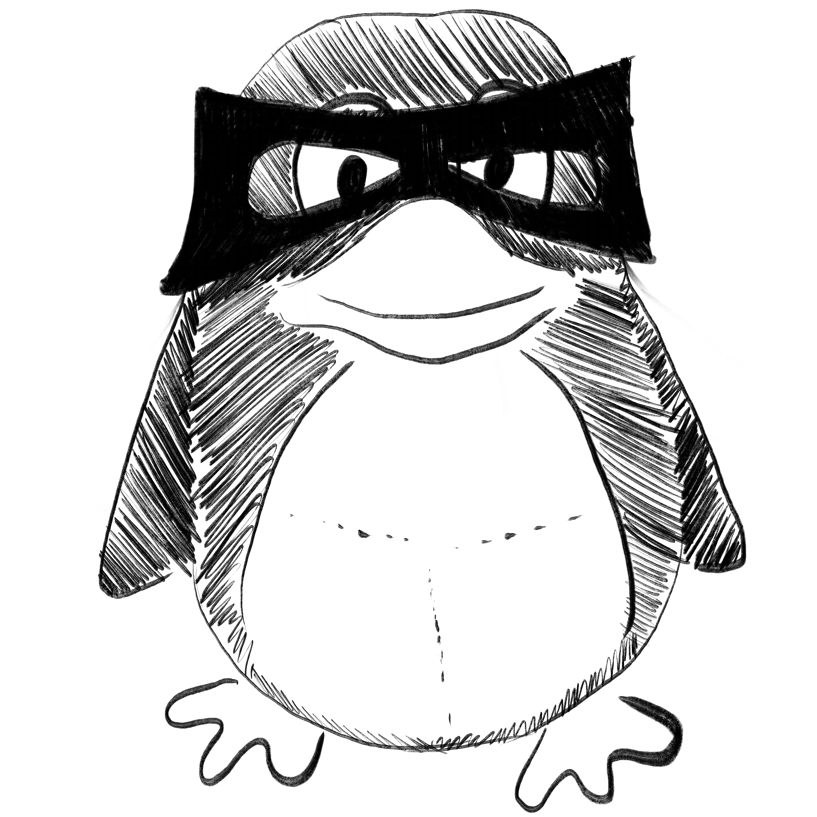
Predicting the development of T1D and identifying its Key Performance Indicators in children; a case-control study in Saudi Arabia.
In PloS one ; h5-index 176.0
Alazwari Ahood, Johnstone Alice, Tafakori Laleh, Abdollahian Mali, AlEidan Ahmed M, Alfuhigi Khalid, Alghofialy Mazen M, Albunyan Abdulhameed A, Al Abbad Hawra, AlEssa Maryam H, Alareefy Abdulaziz K H, Alshamrani Mohammad A
2023
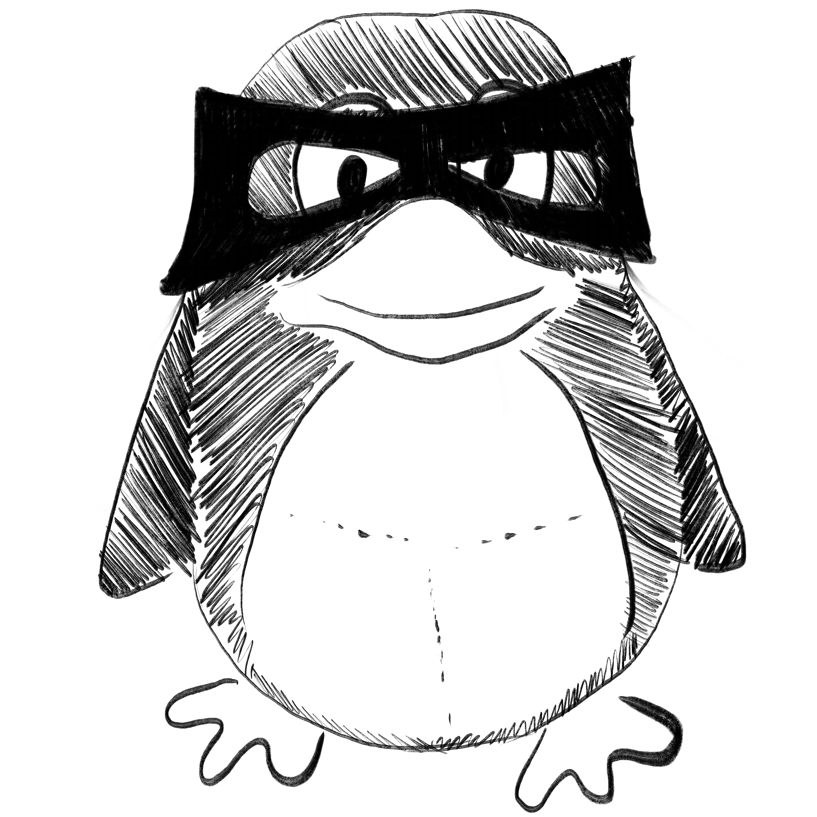
Macaques recognize features in synthetic images derived from ventral stream neurons.
In Proceedings of the National Academy of Sciences of the United States of America
Mueller Katherine N, Carter Mary C, Kansupada Jeevun A, Ponce Carlos R
2023-Mar-07
inferotemporal cortex, machine learning, primary visual cortex, rhesus monkey, visual recognition
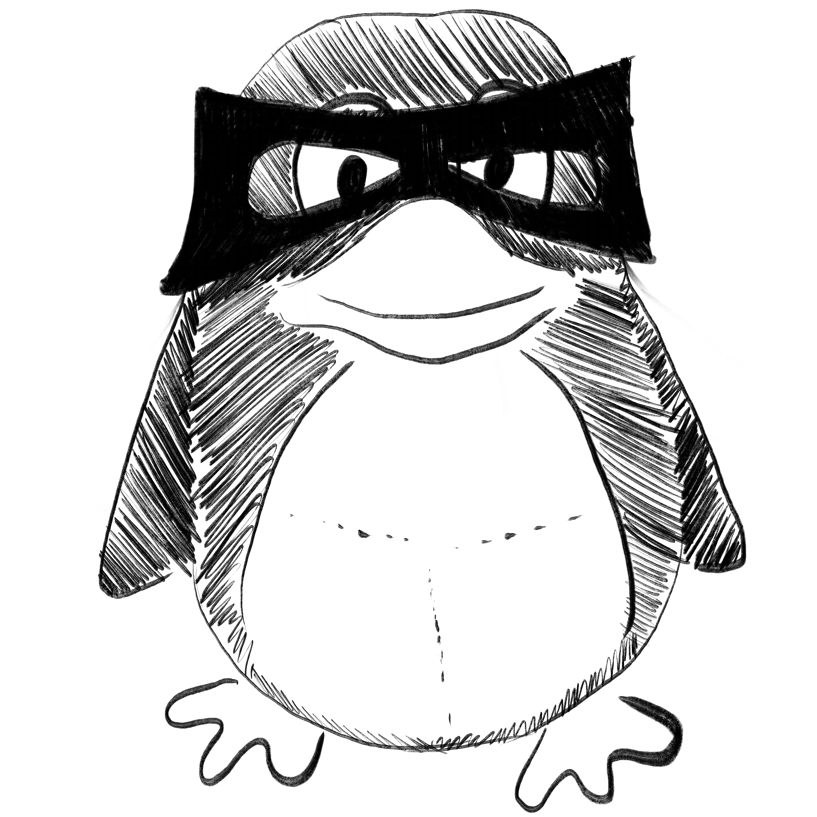
Rett syndrome severity estimation with the BioStamp nPoint using interactions between heart rate variability and body movement.
In PloS one ; h5-index 176.0
Suresha Pradyumna Byappanahalli, O’Leary Heather, Tarquinio Daniel C, Von Hehn Jana, Clifford Gari D
2023
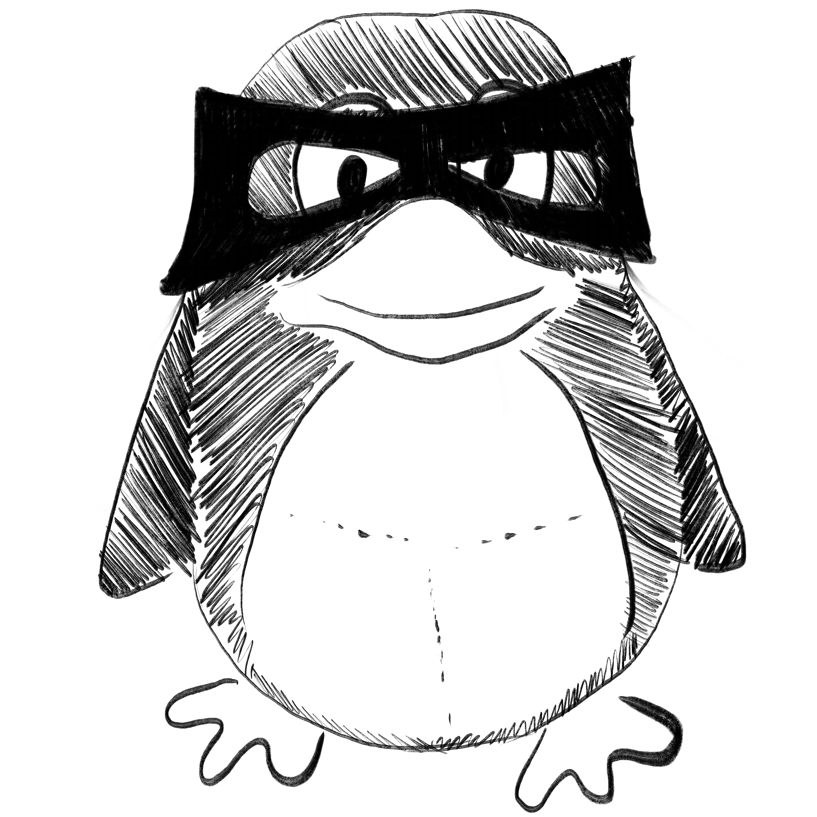
Toward multimode-fiber shape sensing.
In Optics letters
Hadad Barak, Marima Daniel, Magal Nadav, Eyal Avishay, Bahabad Alon
2023-Mar-01
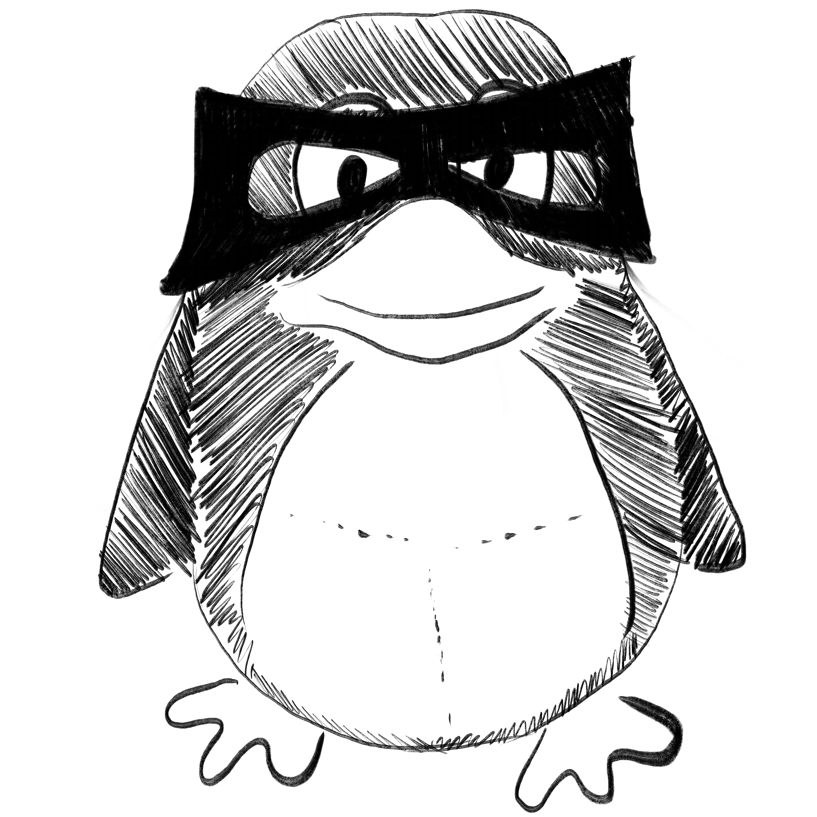
New, fast, and precise method of COVID-19 detection in nasopharyngeal and tracheal aspirate samples combining optical spectroscopy and machine learning.
In Brazilian journal of microbiology : [publication of the Brazilian Society for Microbiology]
Ceccon Denny M, Amaral Paulo Henrique R, Andrade Lídia M, da Silva Maria I N, Andrade Luis A F, Moraes Thais F S, Bagno Flavia F, Rocha Raissa P, de Almeida Marques Daisymara Priscila, Ferreira Geovane Marques, Lourenço Alice Aparecida, Ribeiro Ágata Lopes, Coelho-Dos-Reis Jordana G A, da Fonseca Flavio G, Gonzalez J C
2023-Feb-28
Artificial intelligence, COVID-19, Label-free diagnosis, Machine learning, Optical spectroscopy
Weekly Summary
Receive a weekly summary and discussion of the top papers of the week by leading researchers in the field.