Receive a weekly summary and discussion of the top papers of the week by leading researchers in the field.
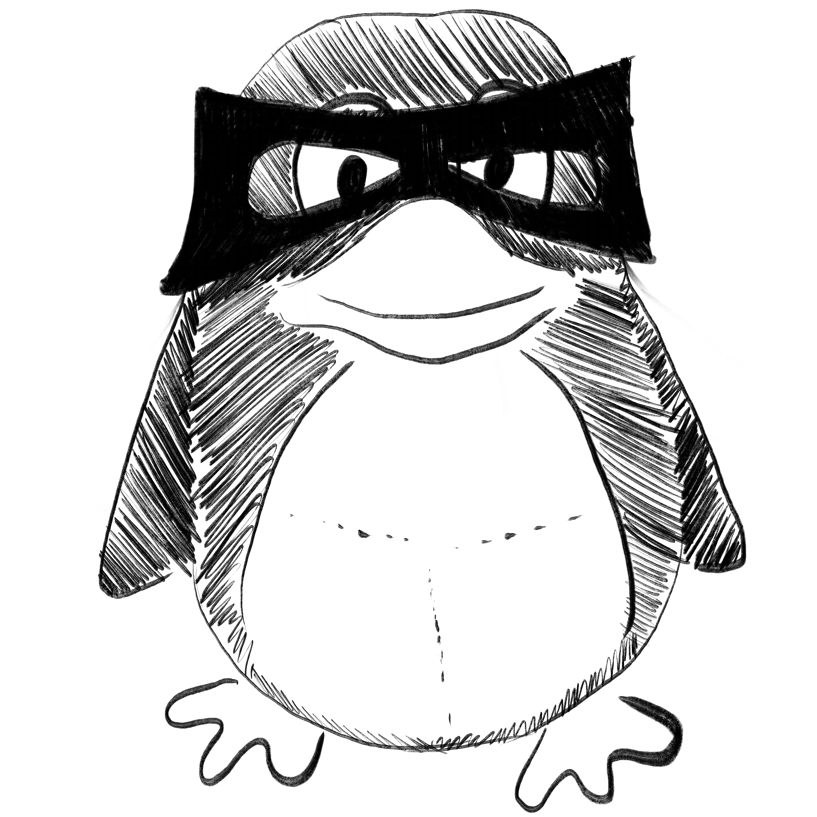
Stain color translation of multi-domain OSCC histopathology images using attention gated cGAN.
In Computerized medical imaging and graphics : the official journal of the Computerized Medical Imaging Society
Barua Barun, Bora Kangkana, Kr Das Anup, Ahmed Gazi N, Rahman Tashnin
2023-Feb-24
Attention gated generator, Conditional generative adversarial network (cGAN), H&E stain normalization, Histopathology, OSCC, Stain translation
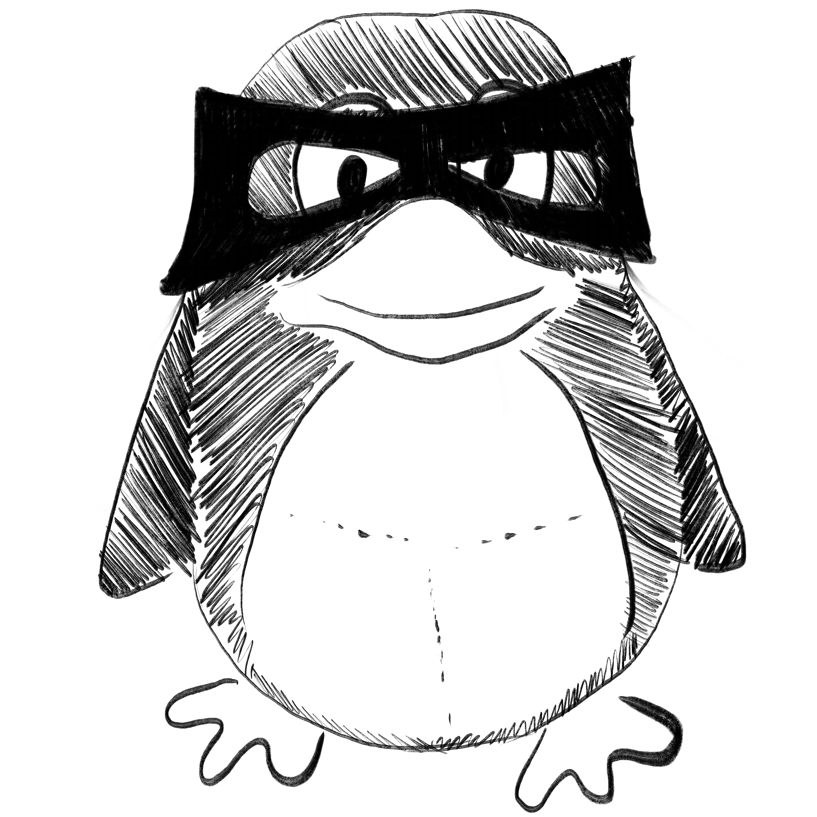
Dual-domain accelerated MRI reconstruction using transformers with learning-based undersampling.
In Computerized medical imaging and graphics : the official journal of the Computerized Medical Imaging Society
Hong Guan Qiu, Wei Yuan Tao, Morley William A W, Wan Matthew, Mertens Alexander J, Su Yang, Cheng Hai-Ling Margaret
2023-Feb-23
Acceleration, Cardiac, Magnetic resonance imaging (MRI), Neural network, Transformer, Undersampling
SynthSeg: Segmentation of brain MRI scans of any contrast and resolution without retraining.
In Medical image analysis
Billot Benjamin, Greve Douglas N, Puonti Oula, Thielscher Axel, Van Leemput Koen, Fischl Bruce, Dalca Adrian V, Iglesias Juan Eugenio
2023-Feb-25
CNN, Contrast and resolution invariance, Domain randomisation, Segmentation
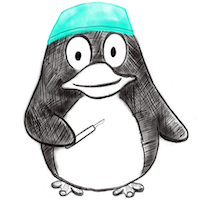
Prediction of epilepsy surgery outcome using foramen ovale EEG - A machine learning approach.
In Epilepsy research
INTRODUCTION :
METHODS :
RESULTS :
CONCLUSION :
Miron Gadi, Müller Paul Manuel, Holtkamp Martin, Meisel Christian
2023-Feb-17
Epilepsy surgery, Foramen ovale, Presurgical evaluation, Surgical outcome prediction
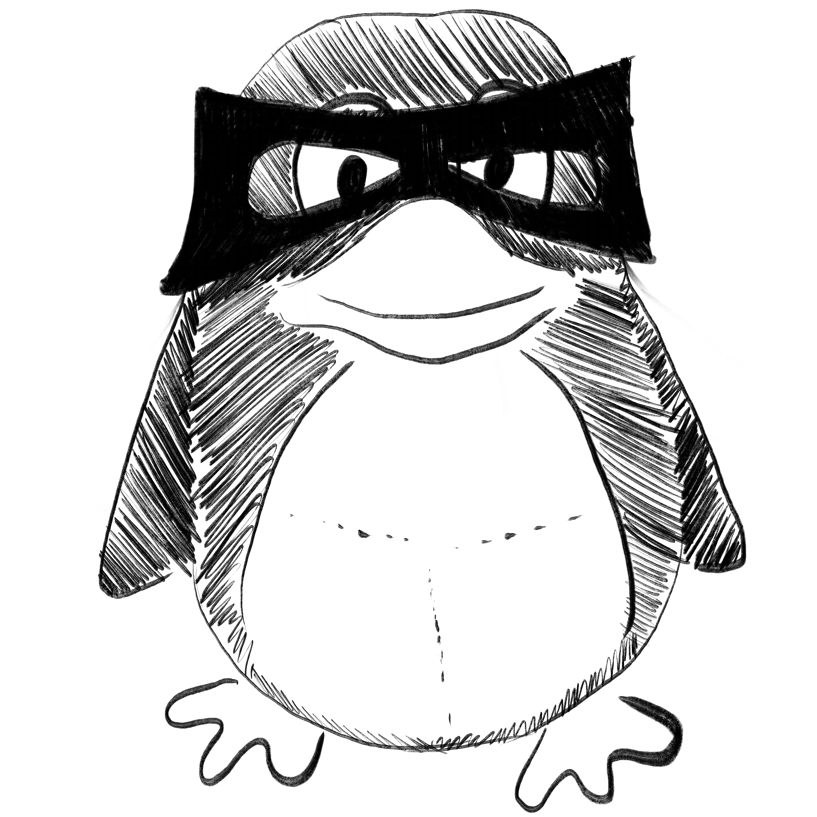
Liquid-solid ratio during hydrothermal carbonization affects hydrochar application potential in soil: Based on characteristics comparison and economic benefit analysis.
In Journal of environmental management
Si Hongyu, Zhao Changkai, Wang Bing, Liang Xiaohui, Gao Mingjie, Jiang Zhaoxia, Yu Hewei, Yang Yuanyuan, Gu Zhijie, Ogino Kenji, Chen Xiuxiu
2023-Feb-27
Corn straw hydrochar, Cost-benefit analysis, Hydrothermal carbonization, Liquid-solid ratio, Soil application
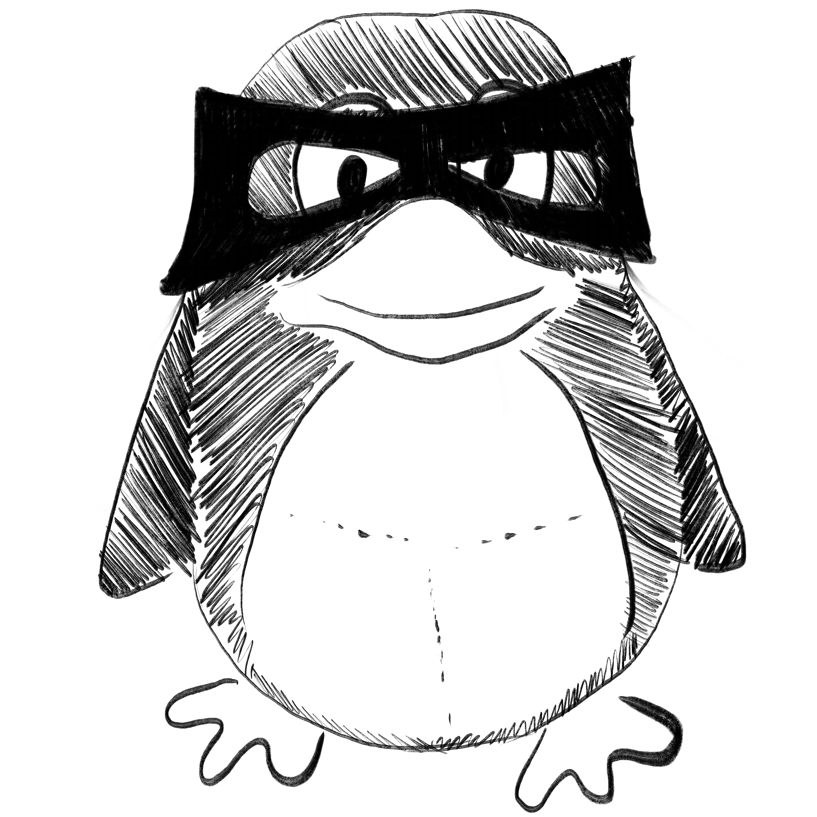
Identifying subtypes of chronic kidney disease with machine learning: development, internal validation and prognostic validation using linked electronic health records in 350,067 individuals.
In EBioMedicine
BACKGROUND :
METHODS :
FINDINGS :
MEDICATIONS :
INTERPRETATION :
FUNDING :
Dashtban Ashkan, Mizani Mehrdad A, Pasea Laura, Denaxas Spiros, Corbett Richard, Mamza Jil B, Gao He, Morris Tamsin, Hemingway Harry, Banerjee Amitava
2023-Feb-27
CKD subtype, Cluster analysis, Machine learning, Survival analysis, Unsupervised clustering
Weekly Summary
Receive a weekly summary and discussion of the top papers of the week by leading researchers in the field.