Receive a weekly summary and discussion of the top papers of the week by leading researchers in the field.
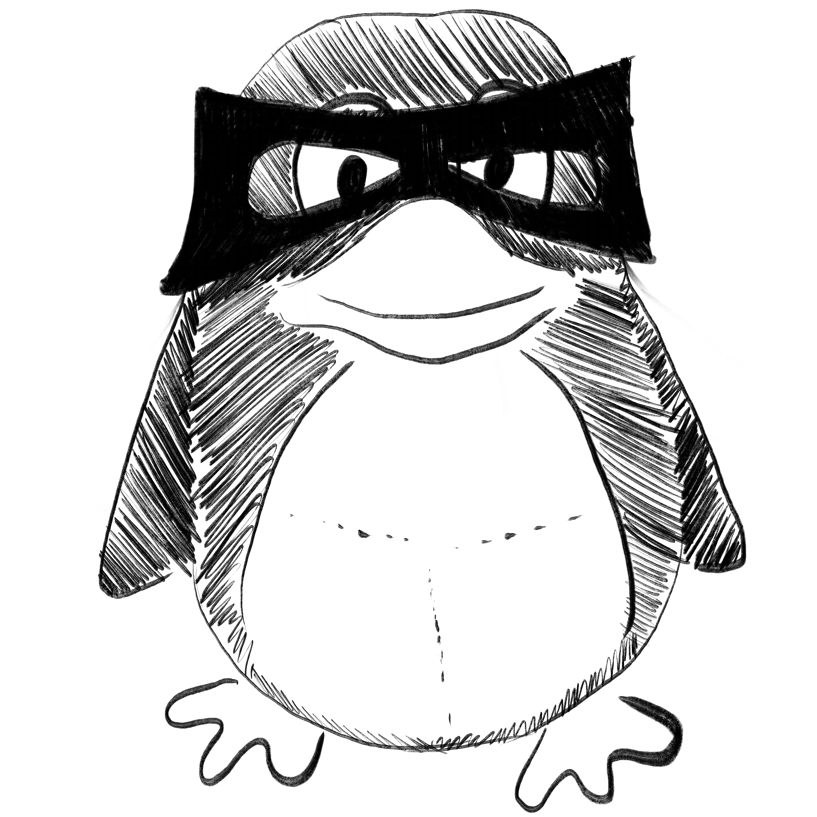
From sonority hierarchy to posterior probability as a measure of lenition: The case of Spanish stops.
In The Journal of the Acoustical Society of America
Tang Kevin, Wayland Ratree, Wang Fenqi, Vellozzi Sophia, Sengupta Rahul, Altmann Lori
2023-Feb
Precision information extraction for rare disease epidemiology at scale.
In Journal of translational medicine
BACKGROUND :
METHODS :
RESULTS :
CONCLUSIONS :
Kariampuzha William Z, Alyea Gioconda, Qu Sue, Sanjak Jaleal, Mathé Ewy, Sid Eric, Chatelaine Haley, Yadaw Arjun, Xu Yanji, Zhu Qian
2023-Feb-28
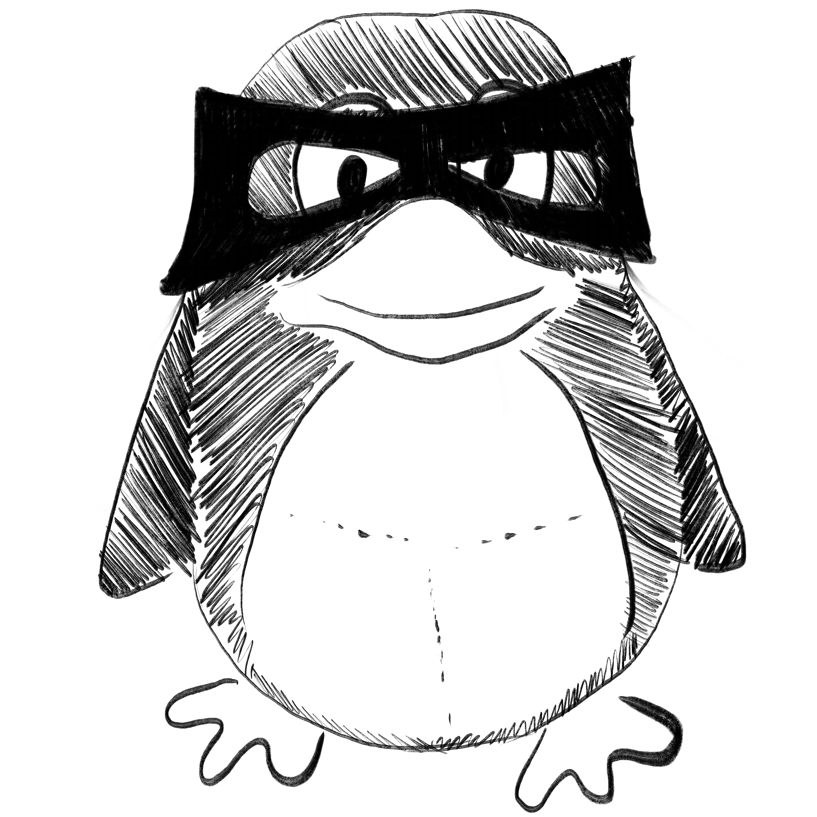
Potential and limitations of machine meta-learning (ensemble) methods for predicting COVID-19 mortality in a large inhospital Brazilian dataset.
In Scientific reports ; h5-index 158.0
de Paiva Bruno Barbosa Miranda, Pereira Polianna Delfino, de Andrade Claudio Moisés Valiense, Gomes Virginia Mara Reis, Souza-Silva Maira Viana Rego, Martins Karina Paula Medeiros Prado, Sales Thaís Lorenna Souza, de Carvalho Rafael Lima Rodrigues, Pires Magda Carvalho, Ramos Lucas Emanuel Ferreira, Silva Rafael Tavares, de Freitas Martins Vieira Alessandra, Nunes Aline Gabrielle Sousa, de Oliveira Jorge Alzira, de Oliveira Maurílio Amanda, Scotton Ana Luiza Bahia Alves, da Silva Carla Thais Candida Alves, Cimini Christiane Corrêa Rodrigues, Ponce Daniela, Pereira Elayne Crestani, Manenti Euler Roberto Fernandes, Rodrigues Fernanda d’Athayde, Anschau Fernando, Botoni Fernando Antônio, Bartolazzi Frederico, Grizende Genna Maira Santos, Noal Helena Carolina, Duani Helena, Gomes Isabela Moraes, Costa Jamille Hemétrio Salles Martins, di Sabatino Santos Guimarães Júlia, Tupinambás Julia Teixeira, Rugolo Juliana Machado, Batista Joanna d’Arc Lyra, de Alvarenga Joice Coutinho, Chatkin José Miguel, Ruschel Karen Brasil, Zandoná Liege Barella, Pinheiro Lílian Santos, Menezes Luanna Silva Monteiro, de Oliveira Lucas Moyses Carvalho, Kopittke Luciane, Assis Luisa Argolo, Marques Luiza Margoto, Raposo Magda Cesar, Floriani Maiara Anschau, Bicalho Maria Aparecida Camargos, Nogueira Matheus Carvalho Alves, de Oliveira Neimy Ramos, Ziegelmann Patricia Klarmann, Paraiso Pedro Gibson, de Lima Martelli Petrônio José, Senger Roberta, Menezes Rochele Mosmann, Francisco Saionara Cristina, Araújo Silvia Ferreira, Kurtz Tatiana, Fereguetti Tatiani Oliveira, de Oliveira Thainara Conceição, Ribeiro Yara Cristina Neves Marques Barbosa, Ramires Yuri Carlotto, Lima Maria Clara Pontello Barbosa, Carneiro Marcelo, Bezerra Adriana Falangola Benjamin, Schwarzbold Alexandre Vargas, de Moura Costa André Soares, Farace Barbara Lopes, Silveira Daniel Vitorio, de Almeida Cenci Evelin Paola, Lucas Fernanda Barbosa, Aranha Fernando Graça, Bastos Gisele Alsina Nader, Vietta Giovanna Grunewald, Nascimento Guilherme Fagundes, Vianna Heloisa Reniers, Guimarães Henrique Cerqueira, de Morais Julia Drumond Parreiras, Moreira Leila Beltrami, de Oliveira Leonardo Seixas, de Deus Sousa Lucas, de Souza Viana Luciano, de Souza Cabral Máderson Alvares, Ferreira Maria Angélica Pires, de Godoy Mariana Frizzo, de Figueiredo Meire Pereira, Guimarães-Junior Milton Henriques, de Paula de Sordi Mônica Aparecida, da Cunha Severino Sampaio Natália, Assaf Pedro Ledic, Lutkmeier Raquel, Valacio Reginaldo Aparecido, Finger Renan Goulart, de Freitas Rufino, Guimarães Silvana Mangeon Meirelles, Oliveira Talita Fischer, Diniz Thulio Henrique Oliveira, Gonçalves Marcos André, Marcolino Milena Soriano
2023-Mar-01
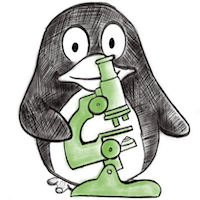
Cluster-Guided Semi-Supervised Domain Adaptation for Imbalanced Medical Image Classification
ArXiv Preprint
Shota Harada, Ryoma Bise, Kengo Araki, Akihiko Yoshizawa, Kazuhiro Terada, Mariyo Kurata, Naoki Nakajima, Hiroyuki Abe, Tetsuo Ushiku, Seiichi Uchida
2023-03-02
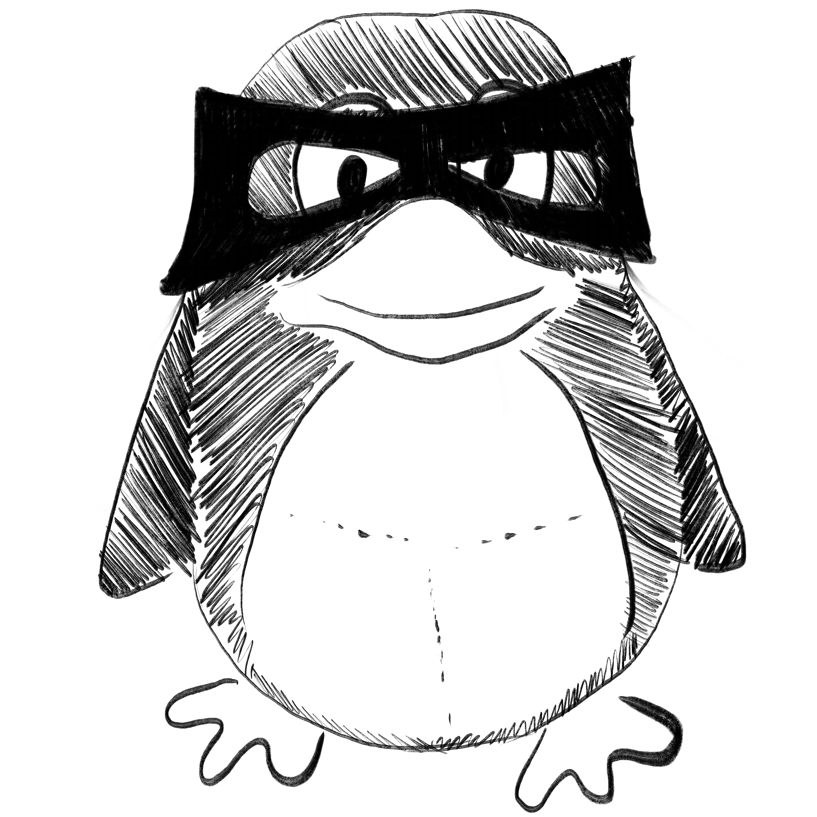
BOTAN: BOnd TArgeting Network for prediction of slow glassy dynamics by machine learning relative motion.
In The Journal of chemical physics
Shiba Hayato, Hanai Masatoshi, Suzumura Toyotaro, Shimokawabe Takashi
2023-Feb-28
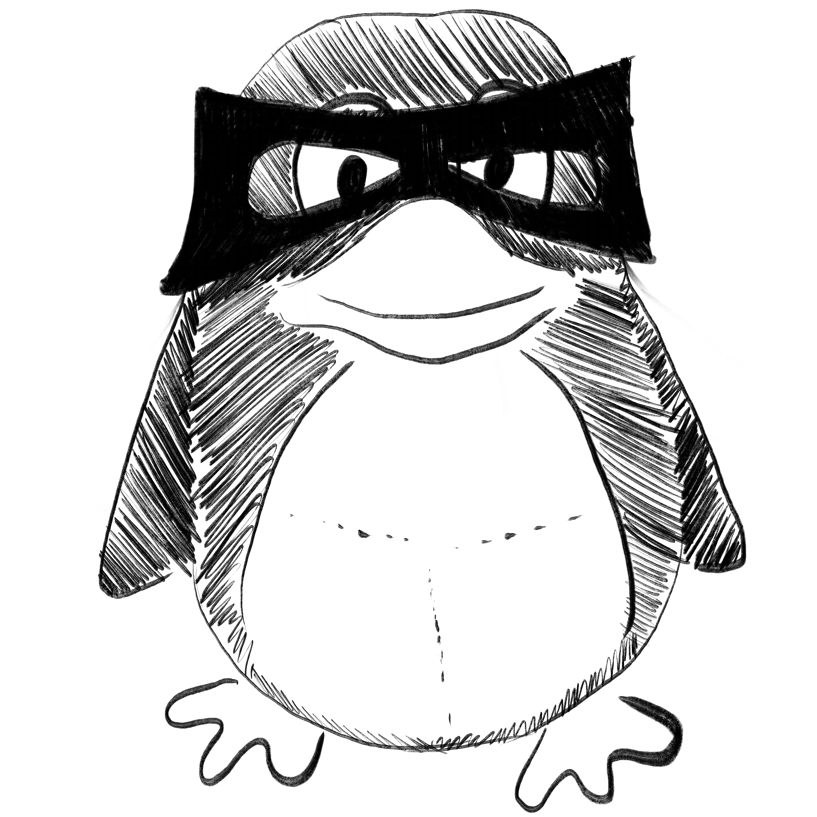
A flexible proton beam imaging energy spectrometer (PROBIES) for high repetition rate or single-shot high energy density (HED) experiments (invited).
In The Review of scientific instruments
Mariscal D A, Djordjević B Z, Anirudh R, Bremer T, Campbell P C, Feister S, Folsom E, Grace E S, Hollinger R, Jacobs S A, Kailkhura B, Kalantar D, Kemp A J, Kim J, Kur E, Liu S, Ludwig J, Morrison J, Nedbailo R, Ose N, Park J, Rocca J J, Scott G G, Simpson R A, Song H, Spears B, Sullivan B, Swanson K K, Thiagarajan J, Wang S, Williams G J, Wilks S C, Wyatt M, Van Essen B, Zacharias R, Zeraouli G, Zhang J, Ma T
2023-Feb-01
Weekly Summary
Receive a weekly summary and discussion of the top papers of the week by leading researchers in the field.