Receive a weekly summary and discussion of the top papers of the week by leading researchers in the field.
Automatic reorientation by deep learning to generate short-axis SPECT myocardial perfusion images.
In Journal of nuclear cardiology : official publication of the American Society of Nuclear Cardiology
BACKGROUND :
METHODS :
RESULTS :
CONCLUSIONS :
Zhu Fubao, Wang Guojie, Zhao Chen, Malhotra Saurabh, Zhao Min, He Zhuo, Shi Jianzhou, Jiang Zhixin, Zhou Weihua
2023-Mar-01
SPECT MPI, convolutional neural networks, deep learning, reorientation
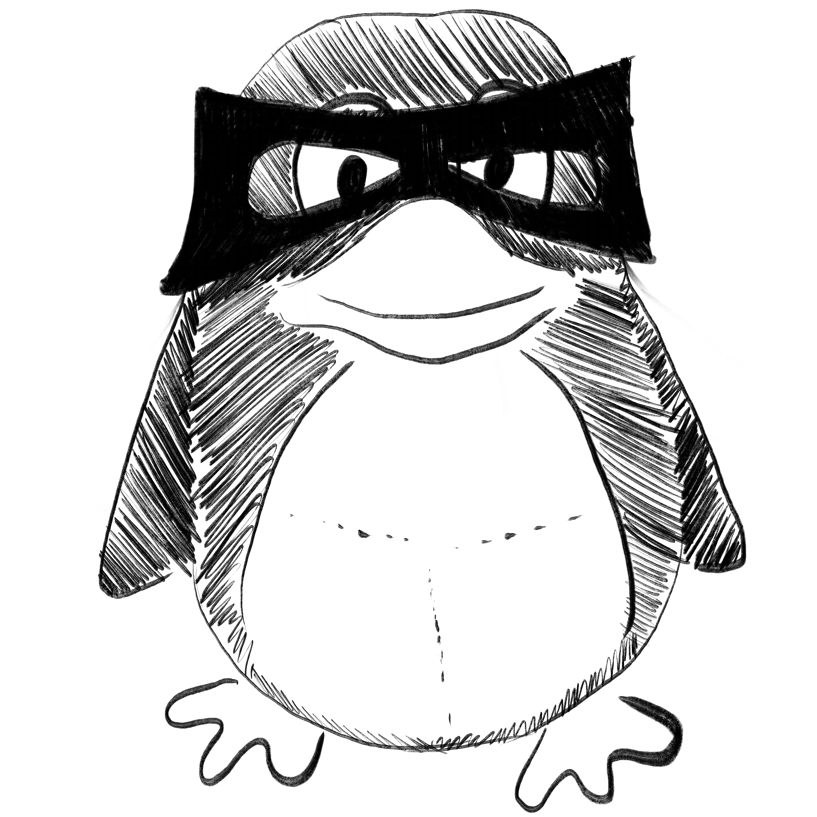
Sub-continental-scale carbon stocks of individual trees in African drylands.
In Nature ; h5-index 368.0
Tucker Compton, Brandt Martin, Hiernaux Pierre, Kariryaa Ankit, Rasmussen Kjeld, Small Jennifer, Igel Christian, Reiner Florian, Melocik Katherine, Meyer Jesse, Sinno Scott, Romero Eric, Glennie Erin, Fitts Yasmin, Morin August, Pinzon Jorge, McClain Devin, Morin Paul, Porter Claire, Loeffler Shane, Kergoat Laurent, Issoufou Bil-Assanou, Savadogo Patrice, Wigneron Jean-Pierre, Poulter Benjamin, Ciais Philippe, Kaufmann Robert, Myneni Ranga, Saatchi Sassan, Fensholt Rasmus
2023-Mar
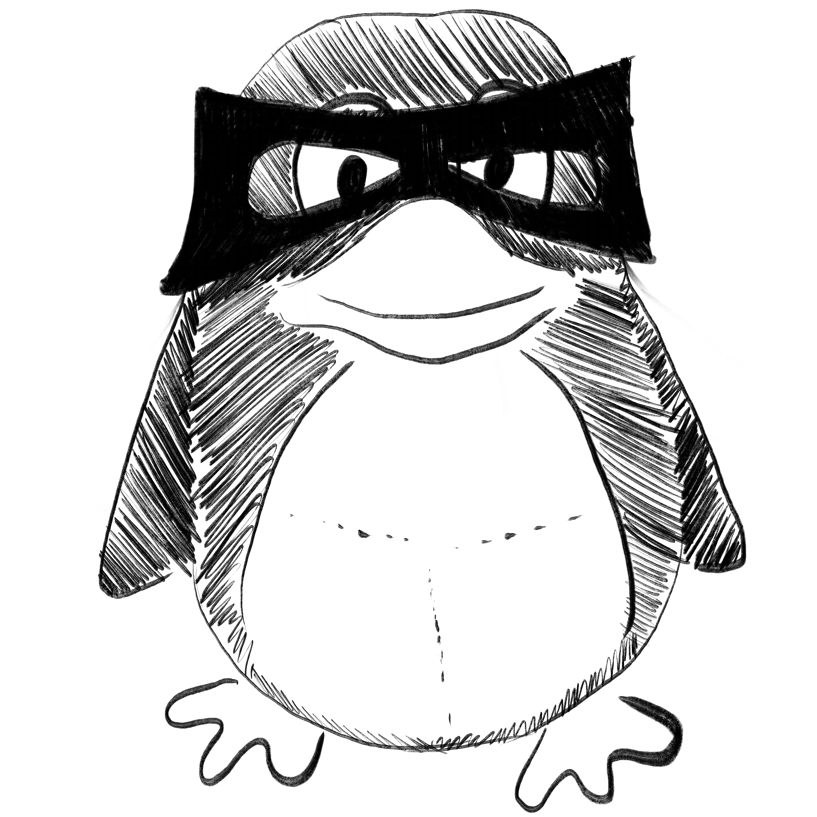
Radar-based human activity recognition with adaptive thresholding towards resource constrained platforms.
In Scientific reports ; h5-index 158.0
Li Zhenghui, Le Kernec Julien, Abbasi Qammer, Fioranelli Francesco, Yang Shufan, Romain Olivier
2023-Mar-01
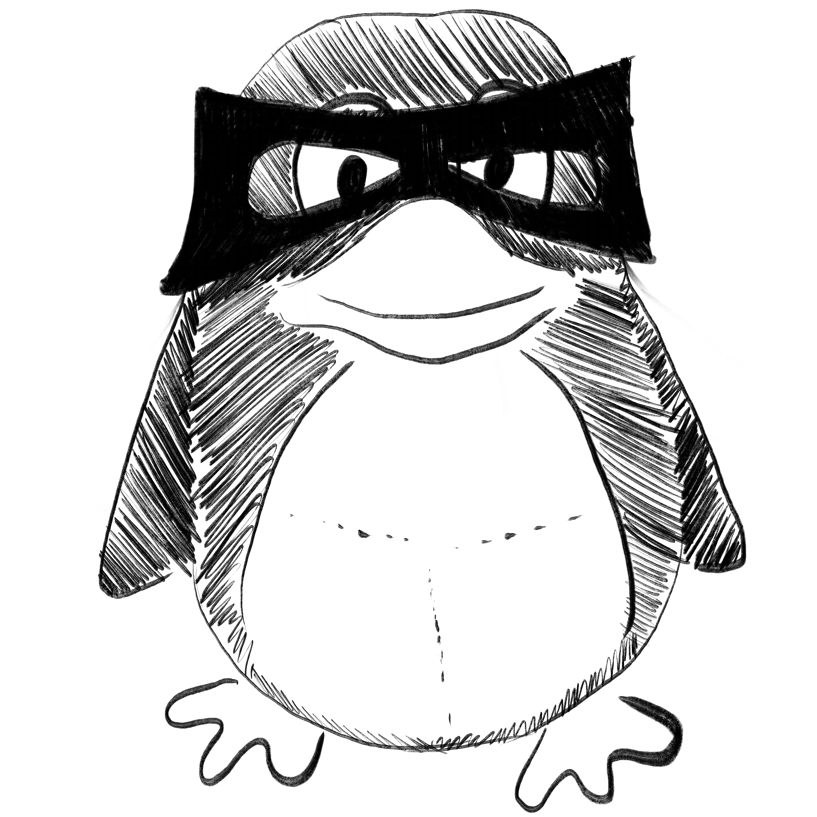
Abnormal cerebellum connectivity patterns related to motor subtypes of Parkinson's disease.
In Journal of neural transmission (Vienna, Austria : 1996)
Chen Zhenzhen, He Chentao, Zhang Piao, Cai Xin, Huang Wenlin, Chen Xi, Xu Mingze, Wang Lijuan, Zhang Yuhu
2023-Mar-01
Cerebellar volume, Functional connectivity, Neural correlates, Parkinson’s disease subtype
Comparative validation of AI and non-AI methods in MRI volumetry to diagnose Parkinsonian syndromes.
In Scientific reports ; h5-index 158.0
Song Joomee, Hahm Juyoung, Lee Jisoo, Lim Chae Yeon, Chung Myung Jin, Youn Jinyoung, Cho Jin Whan, Ahn Jong Hyeon, Kim Kyungsu
2023-Mar-01
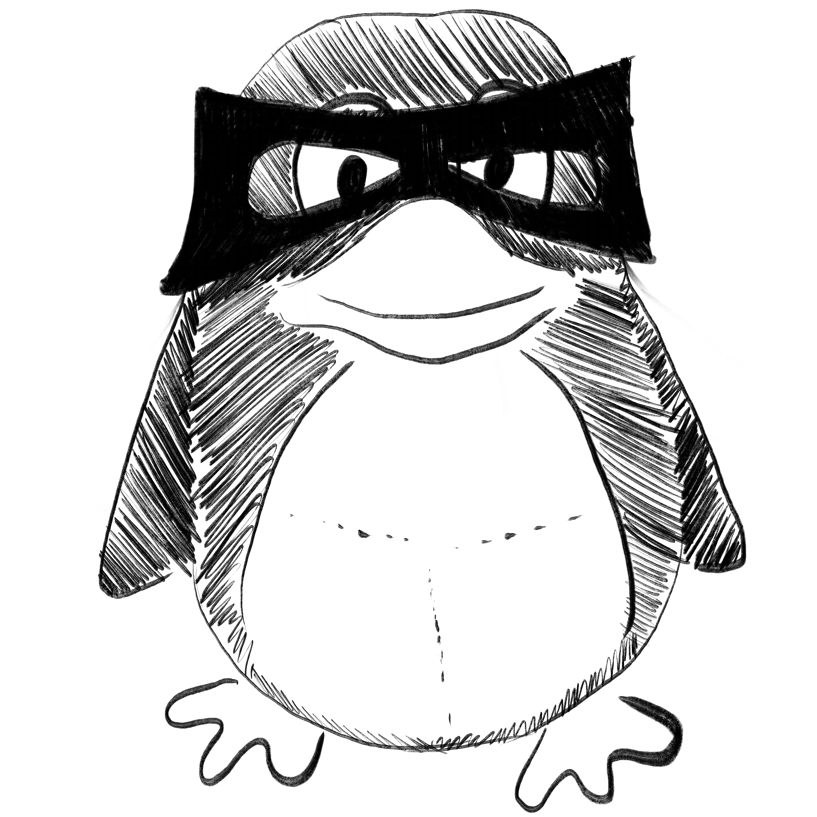
Prediction of transition state structures of gas-phase chemical reactions via machine learning.
In Nature communications ; h5-index 260.0
Choi Sunghwan
2023-Mar-01
Weekly Summary
Receive a weekly summary and discussion of the top papers of the week by leading researchers in the field.