Receive a weekly summary and discussion of the top papers of the week by leading researchers in the field.
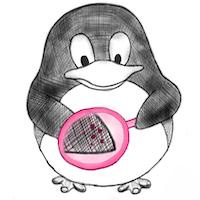
A deep-learning algorithm to classify skin lesions from mpox virus infection.
In Nature medicine ; h5-index 170.0
Thieme Alexander H, Zheng Yuanning, Machiraju Gautam, Sadee Chris, Mittermaier Mirja, Gertler Maximilian, Salinas Jorge L, Srinivasan Krithika, Gyawali Prashnna, Carrillo-Perez Francisco, Capodici Angelo, Uhlig Maximilian, Habenicht Daniel, Löser Anastassia, Kohler Maja, Schuessler Maximilian, Kaul David, Gollrad Johannes, Ma Jackie, Lippert Christoph, Billick Kendall, Bogoch Isaac, Hernandez-Boussard Tina, Geldsetzer Pascal, Gevaert Olivier
2023-Mar-02
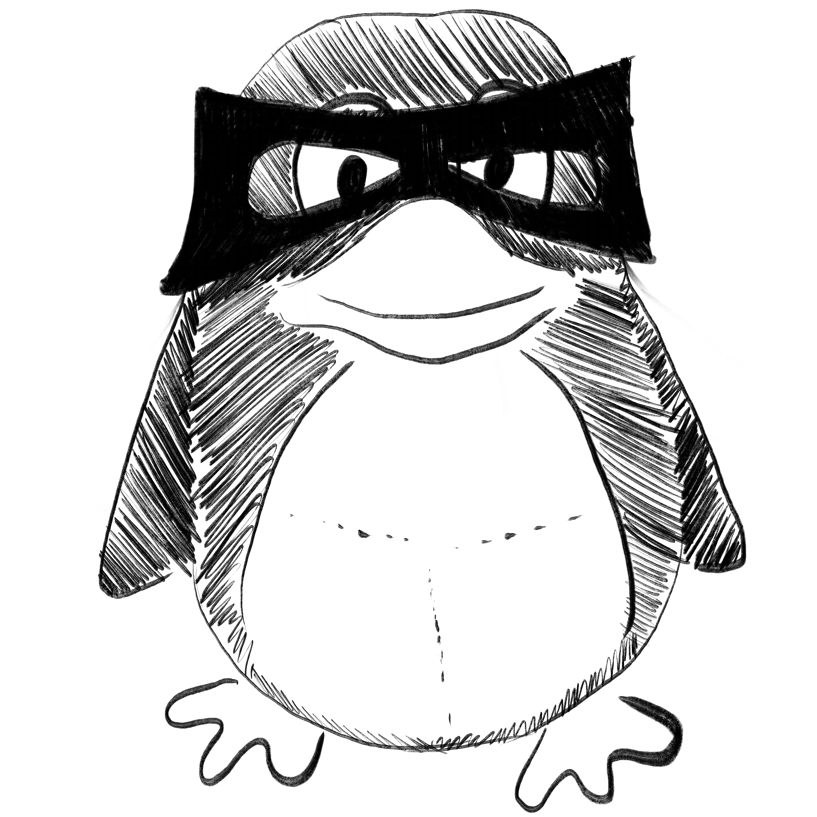
New machine learning-based automatic high-throughput video tracking system for assessing water toxicity using Daphnia Magna locomotory responses.
In Scientific reports ; h5-index 158.0
Kim Jaehoon, Yuk Hyeonseop, Choi Byeongwook, Yang MiSuk, Choi SongBum, Lee Kyoung-Jin, Lee Sungjong, Heo Tae-Young
2023-Mar-02
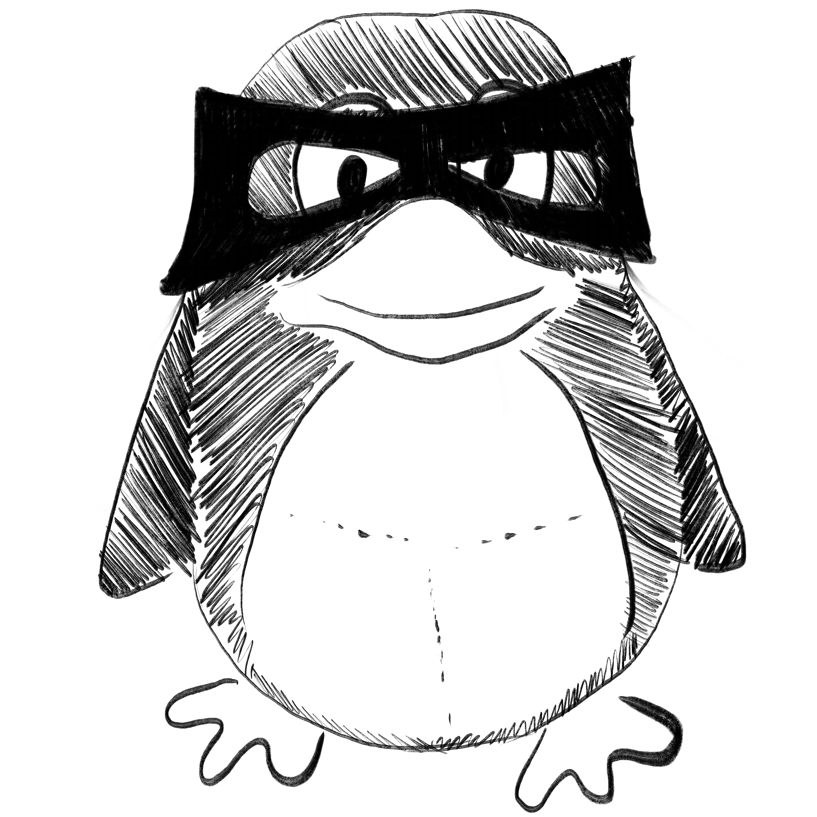
Rare CNVs and phenome-wide profiling highlight brain structural divergence and phenotypical convergence.
In Nature human behaviour
Kopal Jakub, Kumar Kuldeep, Saltoun Karin, Modenato Claudia, Moreau Clara A, Martin-Brevet Sandra, Huguet Guillaume, Jean-Louis Martineau, Martin Charles-Olivier, Saci Zohra, Younis Nadine, Tamer Petra, Douard Elise, Maillard Anne M, Rodriguez-Herreros Borja, Pain Aurèlie, Richetin Sonia, Kushan Leila, Silva Ana I, van den Bree Marianne B M, Linden David E J, Owen Michael J, Hall Jeremy, Lippé Sarah, Draganski Bogdan, Sønderby Ida E, Andreassen Ole A, Glahn David C, Thompson Paul M, Bearden Carrie E, Jacquemont Sébastien, Bzdok Danilo
2023-Mar-02
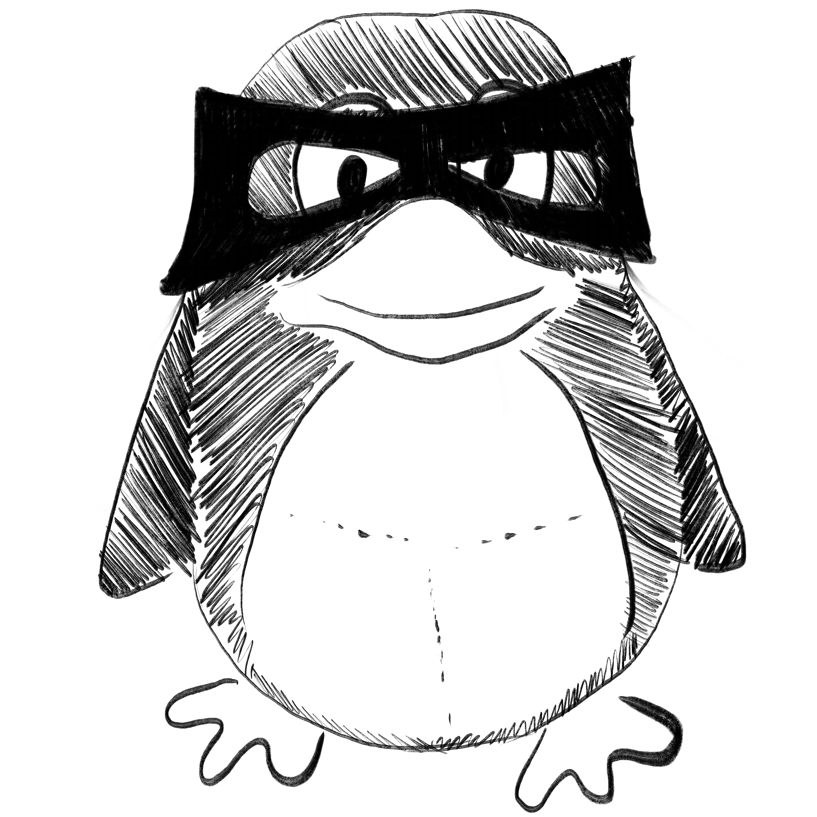
Evidence of a predictive coding hierarchy in the human brain listening to speech.
In Nature human behaviour
Caucheteux Charlotte, Gramfort Alexandre, King Jean-Rémi
2023-Mar-02
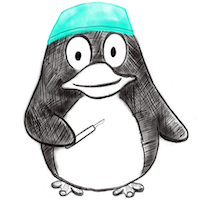
Predicting anterior cruciate ligament failure load with T2* relaxometry and machine learning as a prospective imaging biomarker for revision surgery.
In Scientific reports ; h5-index 158.0
Flannery Sean W, Beveridge Jillian E, Proffen Benedikt L, Walsh Edward G, Kramer Dennis E, Murray Martha M, Kiapour Ata M, Fleming Braden C
2023-Mar-02
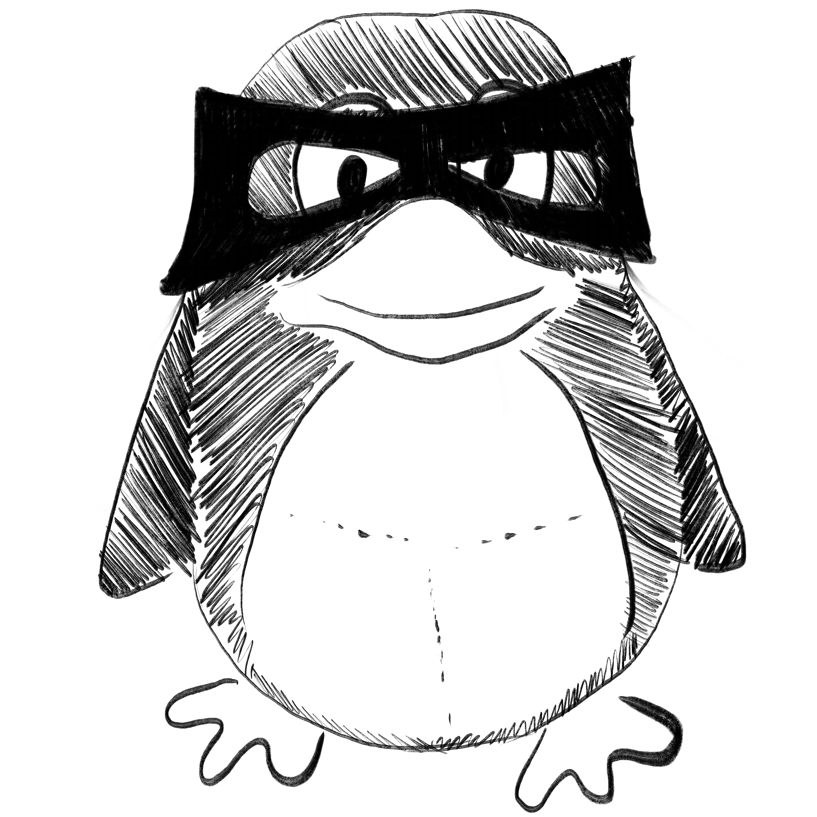
Efficient end-to-end learning for cell segmentation with machine generated weak annotations.
In Communications biology
Shrestha Prem, Kuang Nicholas, Yu Ji
2023-Mar-02
Weekly Summary
Receive a weekly summary and discussion of the top papers of the week by leading researchers in the field.