Receive a weekly summary and discussion of the top papers of the week by leading researchers in the field.
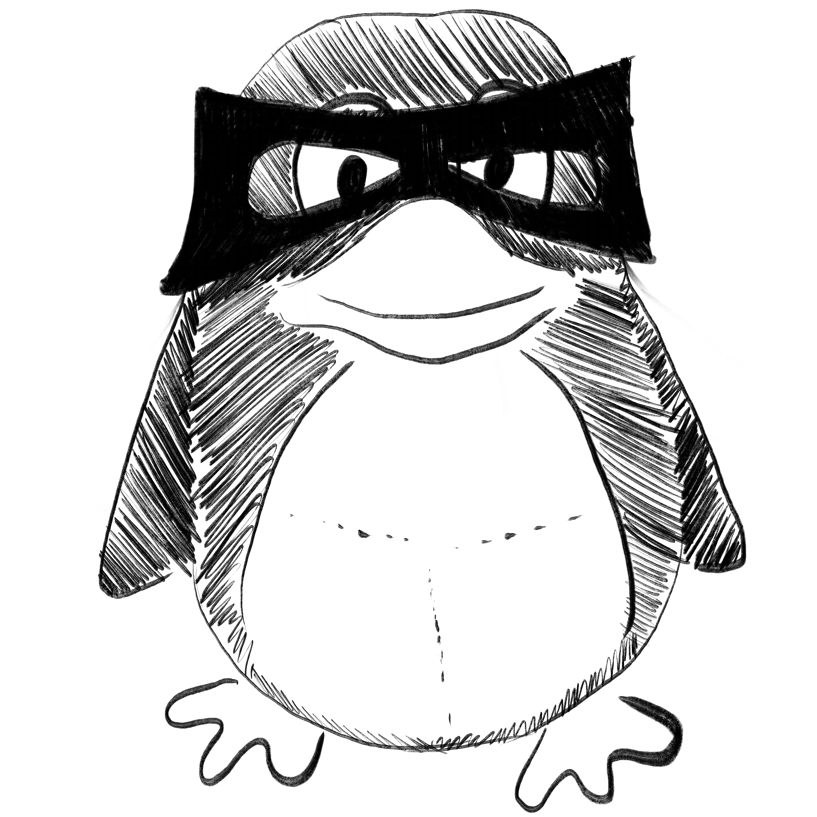
Machine learning-based prediction of candidate gene biomarkers correlated with immune infiltration in patients with idiopathic pulmonary fibrosis.
In Frontiers in medicine
OBJECTIVE :
METHODS :
RESULTS :
CONCLUSION :
Zhang Yufeng, Wang Cong, Xia Qingqing, Jiang Weilong, Zhang Huizhe, Amiri-Ardekani Ehsan, Hua Haibing, Cheng Yi
2023
CIBERSORT, gene biomarker, idiopathic pulmonary fibrosis, immune infiltration, machine learning algorithm
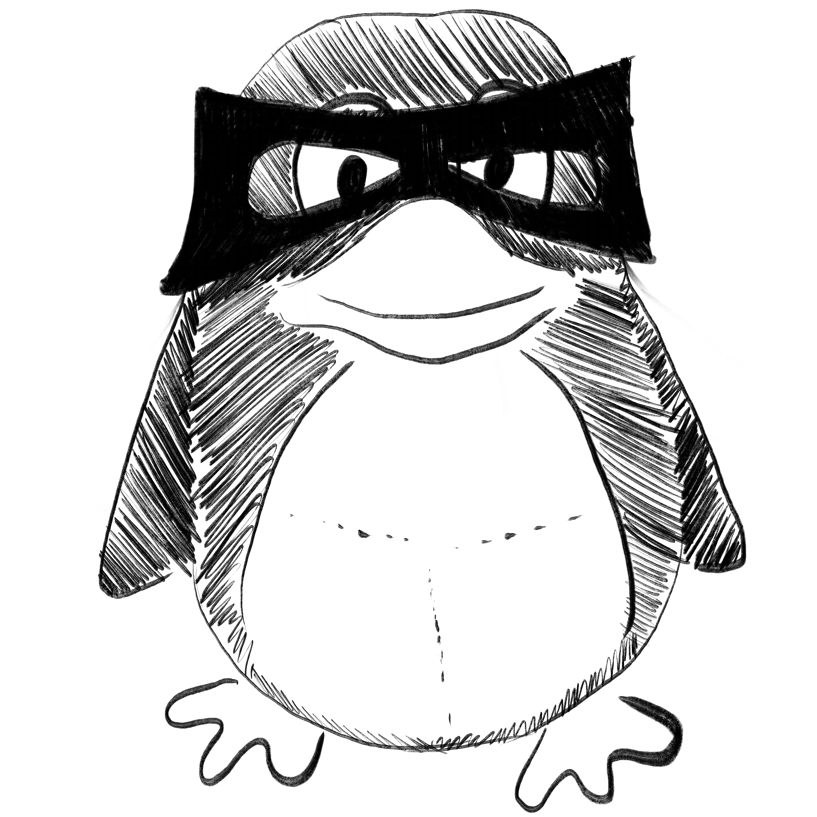
Critical Device Reliability Assessment in Healthcare Services.
In Journal of healthcare engineering
Abd Rahman Noorul Husna, Ibrahim Ayman Khallel, Hasikin Khairunnisa, Abd Razak Nasrul Anuar
2023
Lesion-specific 3D-printed moulds for image-guided tissue multi-sampling of ovarian tumours: A prospective pilot study.
In Frontiers in oncology
BACKGROUND :
METHODS :
RESULTS :
CONCLUSIONS :
Delgado-Ortet Maria, Reinius Marika A V, McCague Cathal, Bura Vlad, Woitek Ramona, Rundo Leonardo, Gill Andrew B, Gehrung Marcel, Ursprung Stephan, Bolton Helen, Haldar Krishnayan, Pathiraja Pubudu, Brenton James D, Crispin-Ortuzar Mireia, Jimenez-Linan Mercedes, Escudero Sanchez Lorena, Sala Evis
2023
3D-printing, cancer imaging, co-registration, custom tumour moulds, ovarian cancer, precision oncology, radiogenomics, tumour sampling
Predicting survival of NSCLC patients treated with immune checkpoint inhibitors: Impact and timing of immune-related adverse events and prior tyrosine kinase inhibitor therapy.
In Frontiers in oncology
INTRODUCTION :
OBJECTIVE :
METHODS :
RESULTS :
CONCLUSION :
Sayer Michael R, Mambetsariev Isa, Lu Kun-Han, Wong Chi Wah, Duche Ashley, Beuttler Richard, Fricke Jeremy, Pharoan Rebecca, Arvanitis Leonidas, Eftekhari Zahra, Amini Arya, Koczywas Marianna, Massarelli Erminia, Roosan Moom Rahman, Salgia Ravi
2023
immune-related adverse events, immunotherapy, machine learning, non-small cell lung cancer, survival analysis, tyrosine kinase inhibitors (TKI)
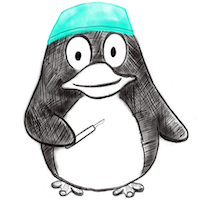
A machine learning approach for predicting descending thoracic aortic diameter.
In Frontiers in cardiovascular medicine
BACKGROUND :
METHODS :
RESULTS :
CONCLUSION :
Yu Ronghuang, Jin Min, Wang Yaohui, Cai Xiujuan, Zhang Keyin, Shi Jian, Zhou Zeyi, Fan Fudong, Pan Jun, Zhou Qing, Tang Xinlong, Wang Dongjin
2023
CTA, TEVAR, aortic diameter, machine learning, predictive model
Development of a prediction model for the Acquisition of Extended Spectrum Beta-Lactam Resistant Organisms in U.S. international travellers.
In Journal of travel medicine
BACKGROUND :
METHODS :
RESULTS :
CONCLUSIONS :
Brown D Garrett, Worby Colin J, Pender Melissa A, Brintz Ben J, Ryan Edward T, Sridhar Sushmita, Oliver Elizabeth, Harris Jason B, Turbett Sarah E, Rao Sowmya R, Earl Ashlee M, LaRocque Regina C, Leung Daniel T
2023-Mar-02
ESBL, antibiotic resistance, clinical prediction, international travel, machine learning
Weekly Summary
Receive a weekly summary and discussion of the top papers of the week by leading researchers in the field.