Receive a weekly summary and discussion of the top papers of the week by leading researchers in the field.
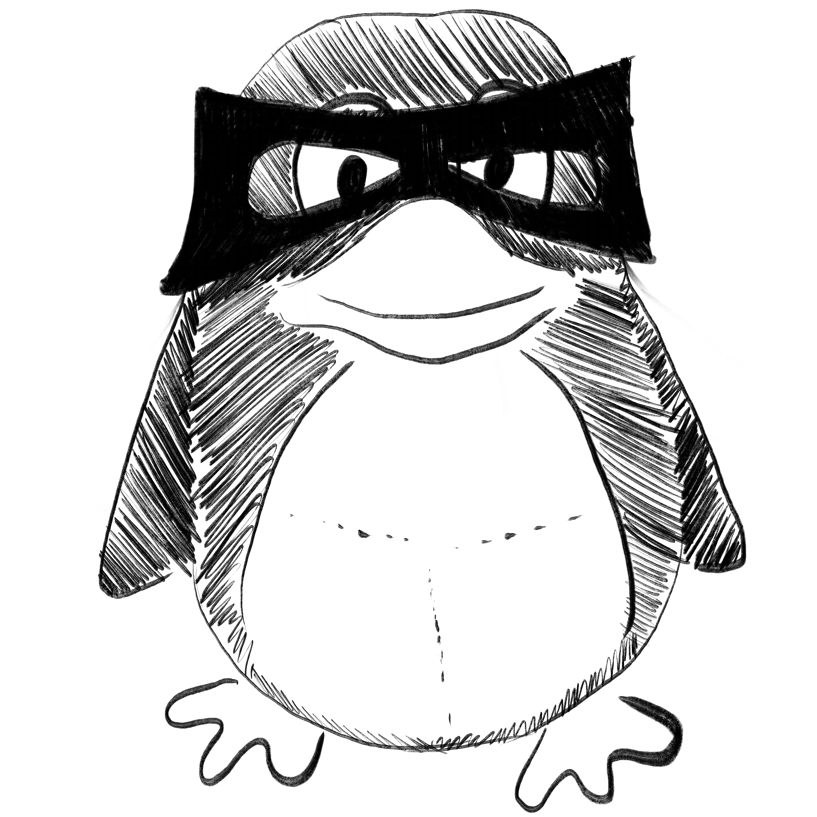
Dual-Phase Inspired Soft Electronic Sensors with Programmable and Tunable Mechanical Properties.
In ACS nano ; h5-index 203.0
Deng Yun, Guo Xiaogang, Lin Yongshui, Huang Zhixin, Li Ying
2023-Mar-02
dual-phase design, flexible display, motion monitoring, programmable metamaterial, skin electronic sensor
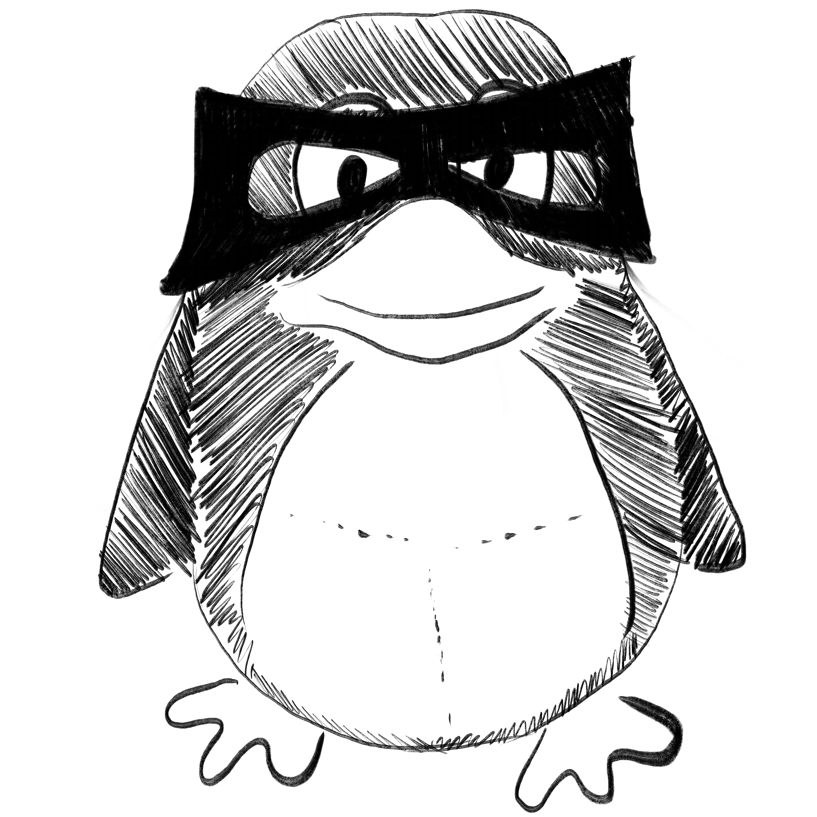
What is the best method for long-term survival analysis?
In Indian journal of cancer
Bekiroglu G Nural, Avci Esin, Ozgur Emrah G
2022
Cox regression, area under the survival curve method, long-term follow-up, restricted mean survival time analysis, survival analysis
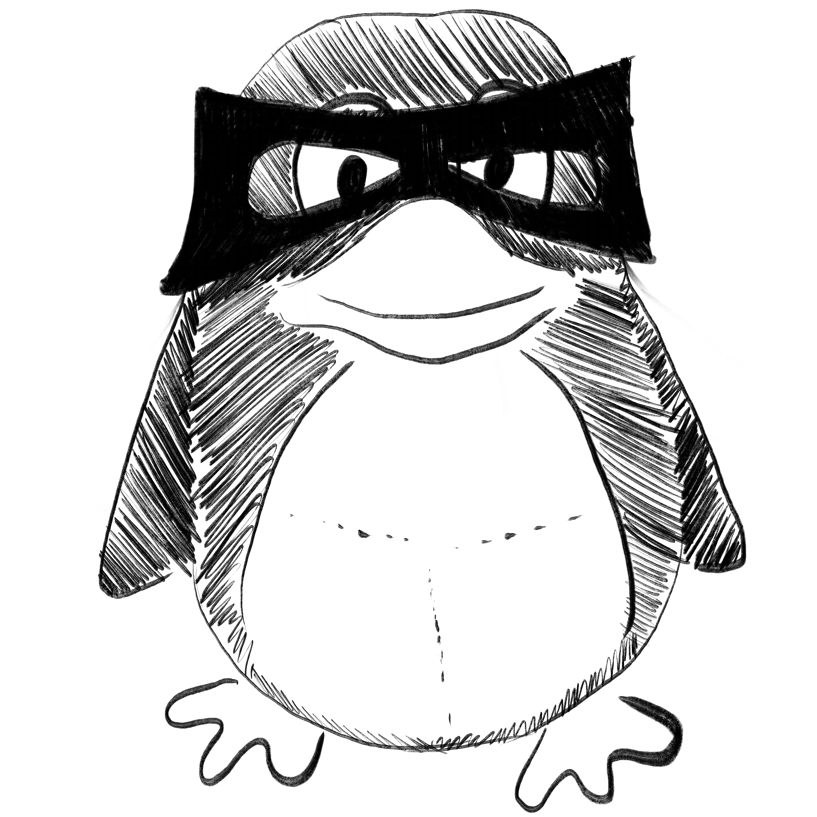
Self-supervised learning for inter-laboratory variation minimization in surface-enhanced Raman scattering spectroscopy.
In The Analyst
Park Seongyong, Wahab Abdul, Kim Minseok, Khan Shujaat
2023-Mar-02
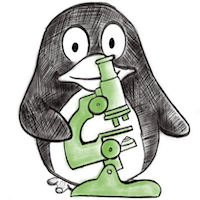
Label-free cleared tissue microscopy and machine learning for 3D histopathology of biomaterial implants.
In Journal of biomedical materials research. Part A
Ngo Tran B, DeStefano Sabrina, Liu Jiamin, Su Yijun, Shroff Hari, Vishwasrao Harshad D, Sadtler Kaitlyn
2023-Mar-02
3D imaging, biomaterials, image segmentation, light-sheet microscopy, optical tissue clearing, pathology, tissue-biomaterial interactions
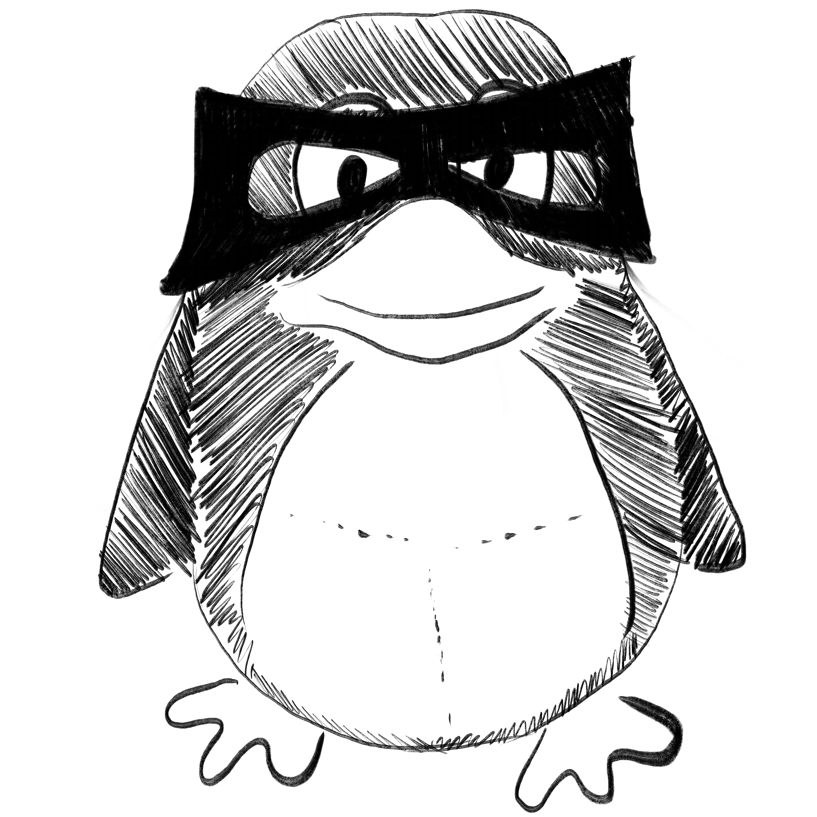
From Basic Sciences and Engineering to Epileptology: A Translational Approach.
In Epilepsia
Bou Assi Elie, Schindler Kaspar, de Bézenac Christophe, Denison Timothy, Desai Sharanya, Keller Simon S, Lemoine Émile, Rahimi Abbas, Shoaran Mahsa, Rummel Christian
2023-Mar-02
Electroencephalography, hyperdimensional computing, intelligent neural prostheses, magnetic resonance imaging, scientific platforms
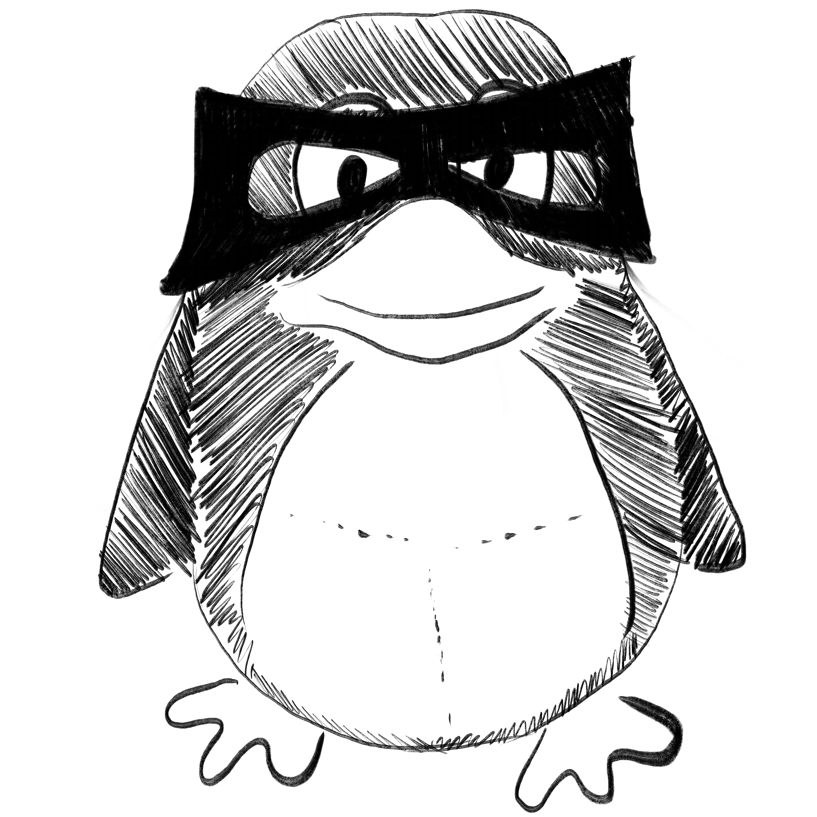
Machine learning within the Parkinson's progression markers initiative: Review of the current state of affairs.
In Frontiers in aging neuroscience ; h5-index 64.0
Gerraty Raphael T, Provost Allison, Li Lin, Wagner Erin, Haas Magali, Lancashire Lee
2023
PD progression, Parkinson’s Disease, data analysis methods, machine learning, multi-omic analyses
Weekly Summary
Receive a weekly summary and discussion of the top papers of the week by leading researchers in the field.