Receive a weekly summary and discussion of the top papers of the week by leading researchers in the field.
Robust framework for COVID-19 identication from a multicenter dataset of chest CT scans.
In PloS one ; h5-index 176.0
Khademi Sadaf, Heidarian Shahin, Afshar Parnian, Enshaei Nastaran, Naderkhani Farnoosh, Rafiee Moezedin Javad, Oikonomou Anastasia, Shafiee Akbar, Babaki Fard Faranak, Plataniotis Konstantinos N, Mohammadi Arash
2023
The early neutrophil-committed progenitors aberrantly differentiate into immunoregulatory monocytes during emergency myelopoiesis.
In Cell reports ; h5-index 119.0
Ikeda Naoki, Kubota Hiroaki, Suzuki Risa, Morita Mitsuki, Yoshimura Ayana, Osada Yuya, Kishida Keigo, Kitamura Daiki, Iwata Ayaka, Yotsumoto Satoshi, Kurotaki Daisuke, Nishimura Koutarou, Nishiyama Akira, Tamura Tomohiko, Kamatani Takashi, Tsunoda Tatsuhiko, Murakawa Miyako, Asahina Yasuhiro, Hayashi Yoshihiro, Harada Hironori, Harada Yuka, Yokota Asumi, Hirai Hideyo, Seki Takao, Kuwahara Makoto, Yamashita Masakatsu, Shichino Shigeyuki, Tanaka Masato, Asano Kenichi
2023-Feb-28
CP: Immunology, CXCR1, G-CSF, Ym1, demand-adapted myelopoiesis, emergency myelopoiesis, machine learning, monocyte, neutrophil-like monocyte, ontogeny
Unassisted Clinicians Versus Deep Learning-Assisted Clinicians in Image-Based Cancer Diagnostics: Systematic Review With Meta-analysis.
In Journal of medical Internet research ; h5-index 88.0
BACKGROUND :
OBJECTIVE :
METHODS :
RESULTS :
CONCLUSIONS :
TRIAL REGISTRATION :
Xue Peng, Si Mingyu, Qin Dongxu, Wei Bingrui, Seery Samuel, Ye Zichen, Chen Mingyang, Wang Sumeng, Song Cheng, Zhang Bo, Ding Ming, Zhang Wenling, Bai Anying, Yan Huijiao, Dang Le, Zhao Yuqian, Rezhake Remila, Zhang Shaokai, Qiao Youlin, Qu Yimin, Jiang Yu
2023-Mar-02
cancer diagnosis, deep learning, meta-analysis, systematic review
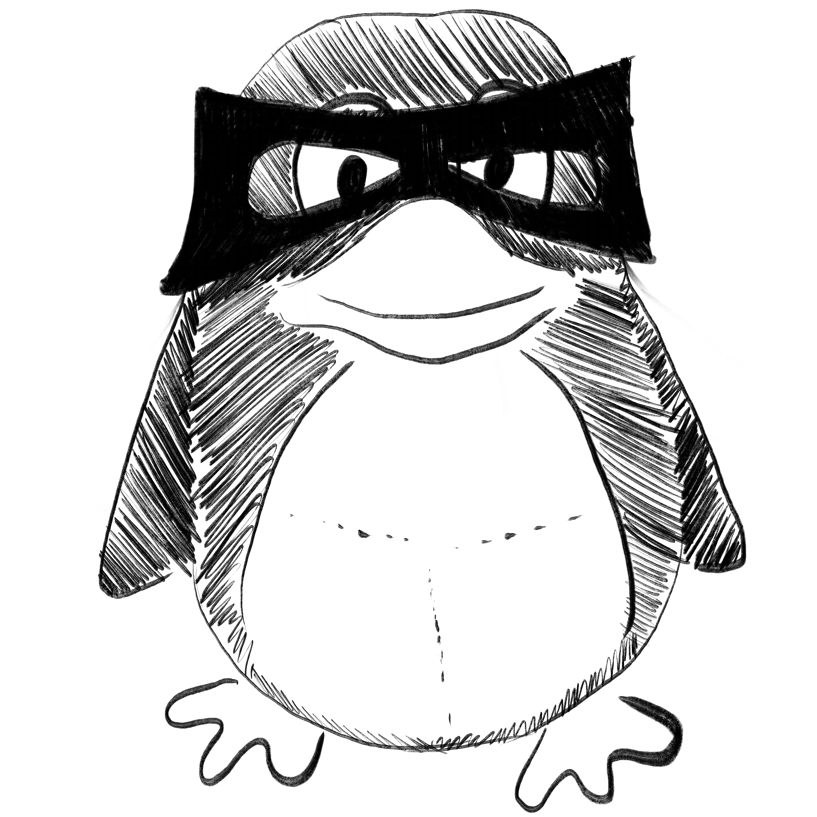
Smartphone Global Positioning System-Based System to Assess Mobility in Health Research: Development, Accuracy, and Usability Study.
In JMIR rehabilitation and assistive technologies
BACKGROUND :
OBJECTIVE :
METHODS :
RESULTS :
CONCLUSIONS :
INTERNATIONAL REGISTERED REPORT IDENTIFIER (IRRID) :
Spang Robert P, Haeger Christine, Mümken Sandra A, Brauer Max, Voigt-Antons Jan-Niklas, Gellert Paul
2023-Mar-02
geographic information system, geoinformatics, out-of-home mobility, prevention medicine, rehabilitation
The Health Care Utilization and Medical Costs in Long-Term Follow-Up of Children Diagnosed With Leukemia, Solid Tumor, or Brain Tumor: Population-Based Study Using the National Health Insurance Claims Data.
In JMIR public health and surveillance
BACKGROUND :
OBJECTIVE :
METHODS :
RESULTS :
CONCLUSIONS :
Miser James S, Shia Ben-Chang, Kao Yi-Wei, Liu Yen-Lin, Chen Shih-Yen, Ho Wan-Ling
2023-Mar-02
brain tumor, cancer survivor, children, cost of care, health care, health resource, leukemia, long-term follow-up, population-based study, solid tumor
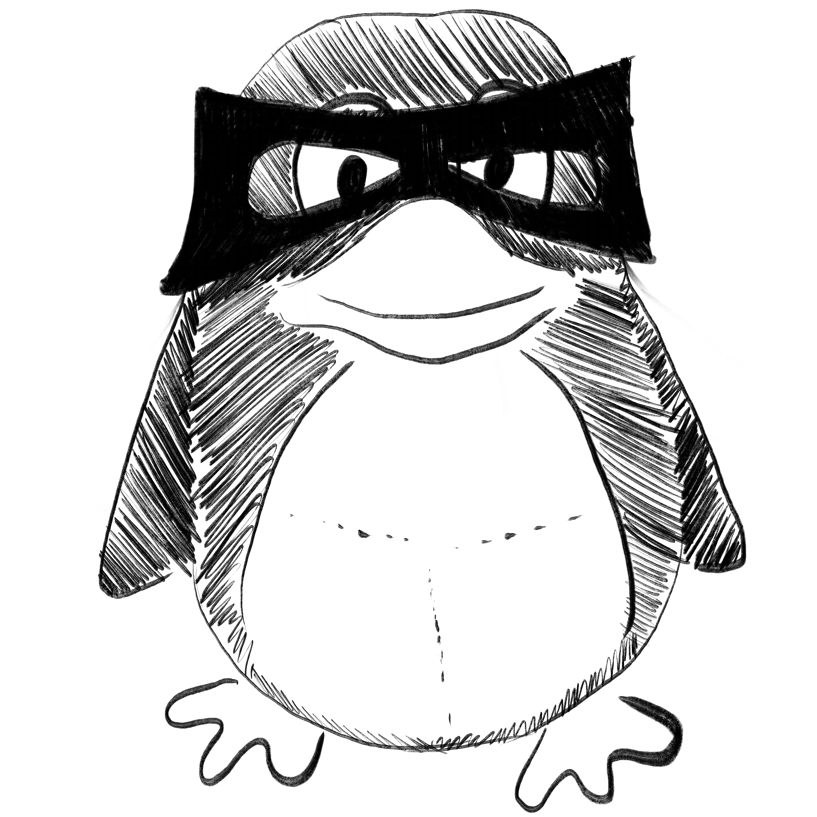
Assessment of Natural Language Processing of Electronic Health Records to Measure Goals-of-Care Discussions as a Clinical Trial Outcome.
In JAMA network open
IMPORTANCE :
OBJECTIVE :
DESIGN, SETTING, AND PARTICIPANTS :
MAIN OUTCOMES AND MEASURES :
RESULTS :
CONCLUSIONS AND RELEVANCE :
Lee Robert Y, Kross Erin K, Torrence Janaki, Li Kevin S, Sibley James, Cohen Trevor, Lober William B, Engelberg Ruth A, Curtis J Randall
2023-Mar-01
Weekly Summary
Receive a weekly summary and discussion of the top papers of the week by leading researchers in the field.