Receive a weekly summary and discussion of the top papers of the week by leading researchers in the field.
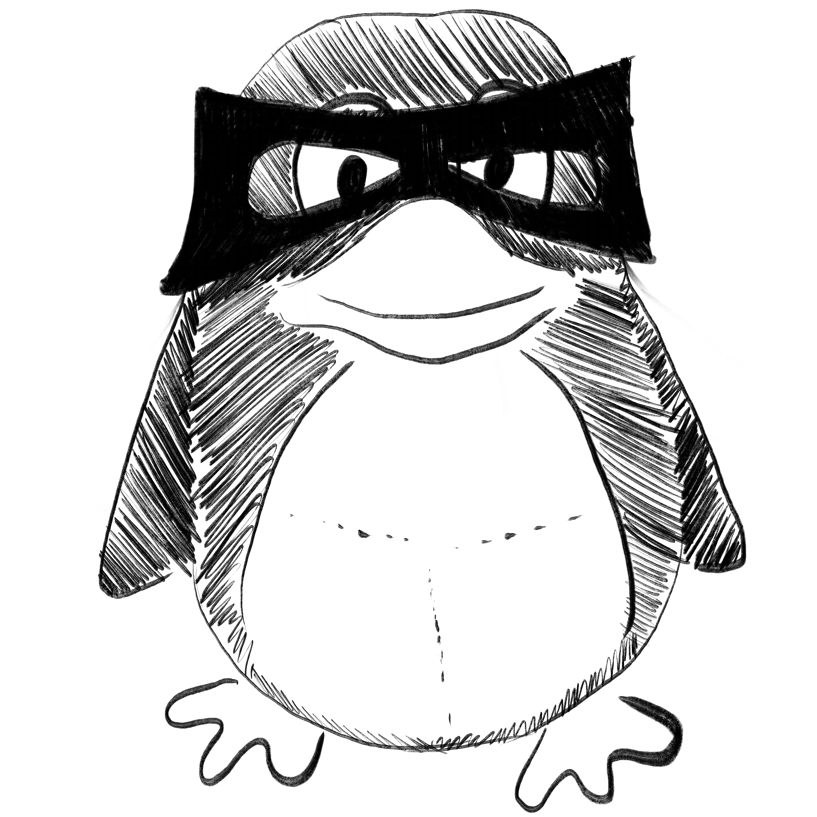
Accurate volume alignment of arbitrarily oriented tibiae based on a mutual attention network for osteoarthritis analysis.
In Computerized medical imaging and graphics : the official journal of the Computerized Medical Imaging Society
Zheng Jian-Qing, Lim Ngee Han, Papież Bartłomiej W
2023-Feb-24
Deep learning, Image registration, Mutual attention, Tibiae CT
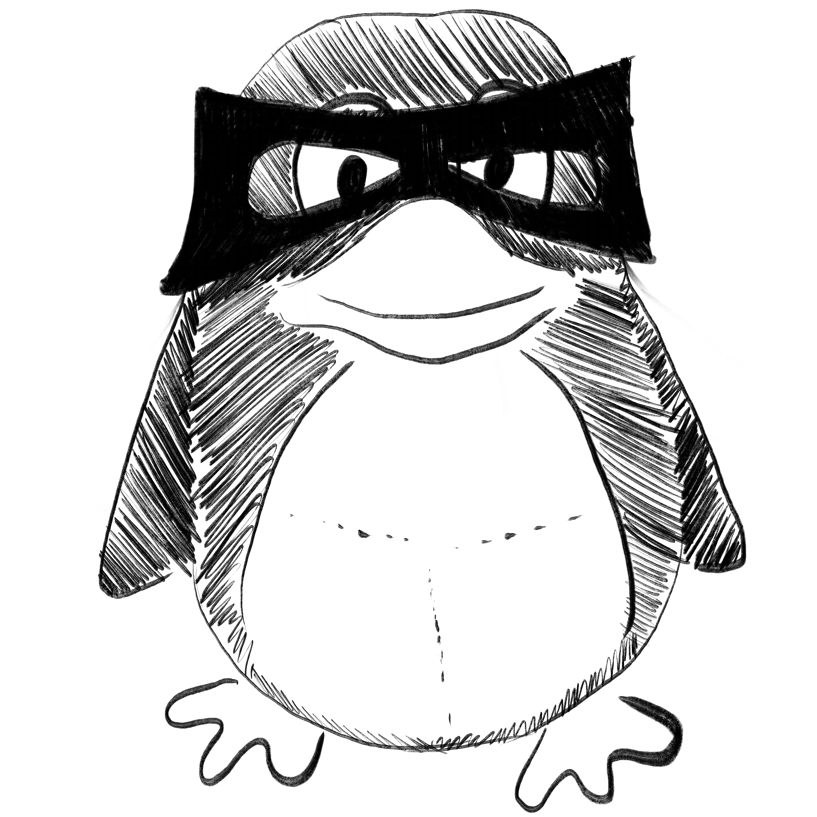
Survey of explainable artificial intelligence techniques for biomedical imaging with deep neural networks.
In Computers in biology and medicine
Nazir Sajid, Dickson Diane M, Akram Muhammad Usman
2023-Feb-18
Backpropagation, Blackbox, Diagnostic imaging, Features, Interpretable AI, Neural networks, Predictive models, Supervised learning
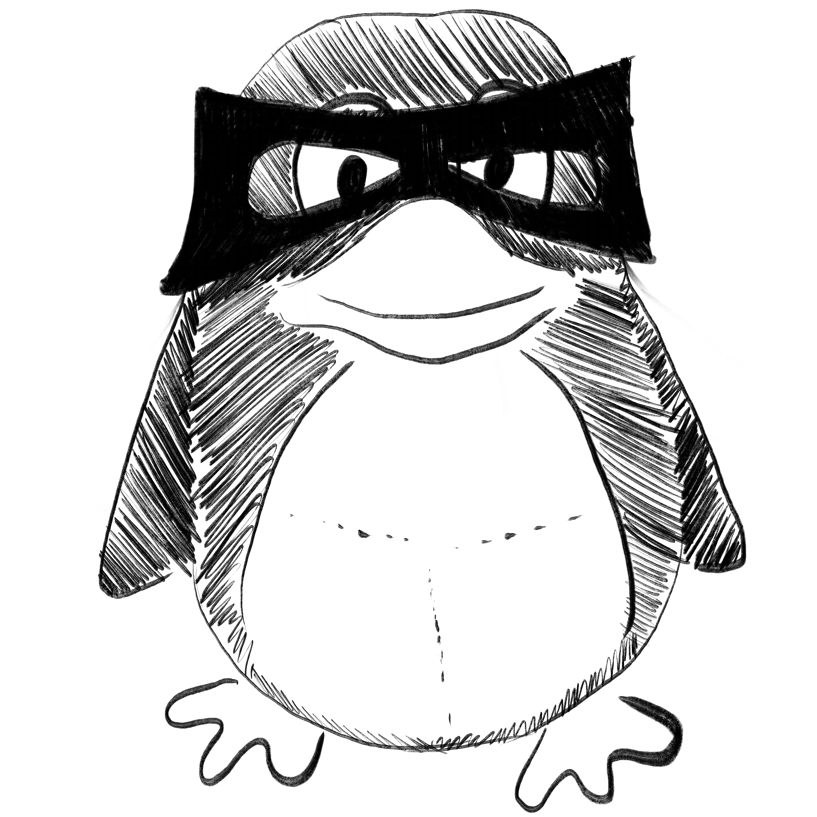
EchoEFNet: Multi-task deep learning network for automatic calculation of left ventricular ejection fraction in 2D echocardiography.
In Computers in biology and medicine
Li Honghe, Wang Yonghuai, Qu Mingjun, Cao Peng, Feng Chaolu, Yang Jinzhu
2023-Feb-24
Deep learning, Echocardiogram, Ejection fraction, Multitasking
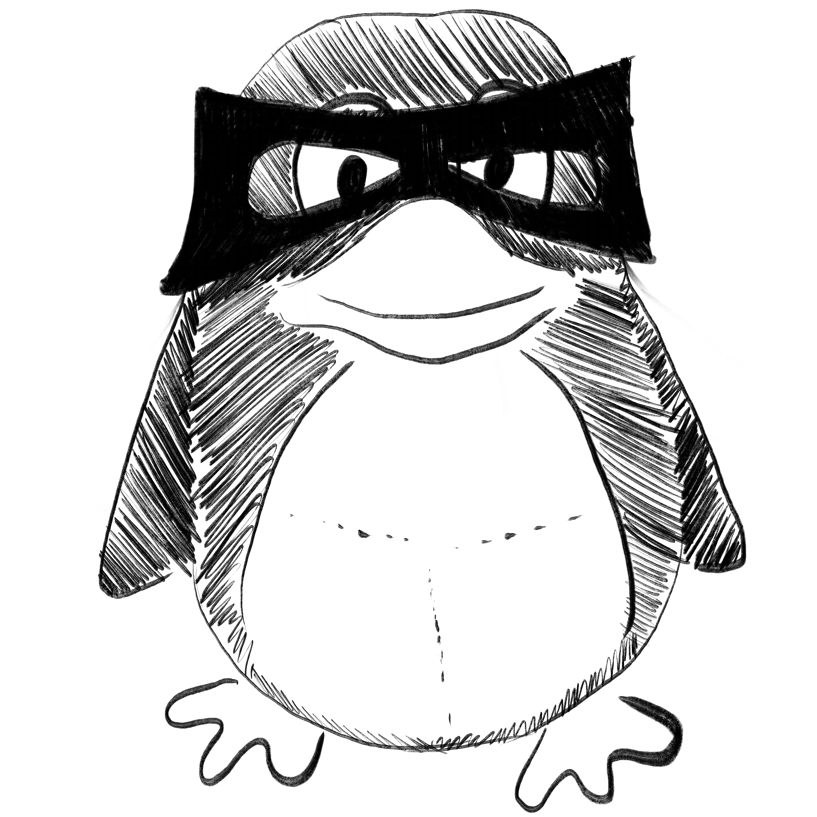
Spectroscopic measurement of the two-dimensional flame temperature based on a perovskite single photodetector.
In Optics express
Wang Jia, Hao Xiaojian, Pan Baowu, Huang Xiaodong, Sun Haoliang, Pei Pan
2023-Feb-27
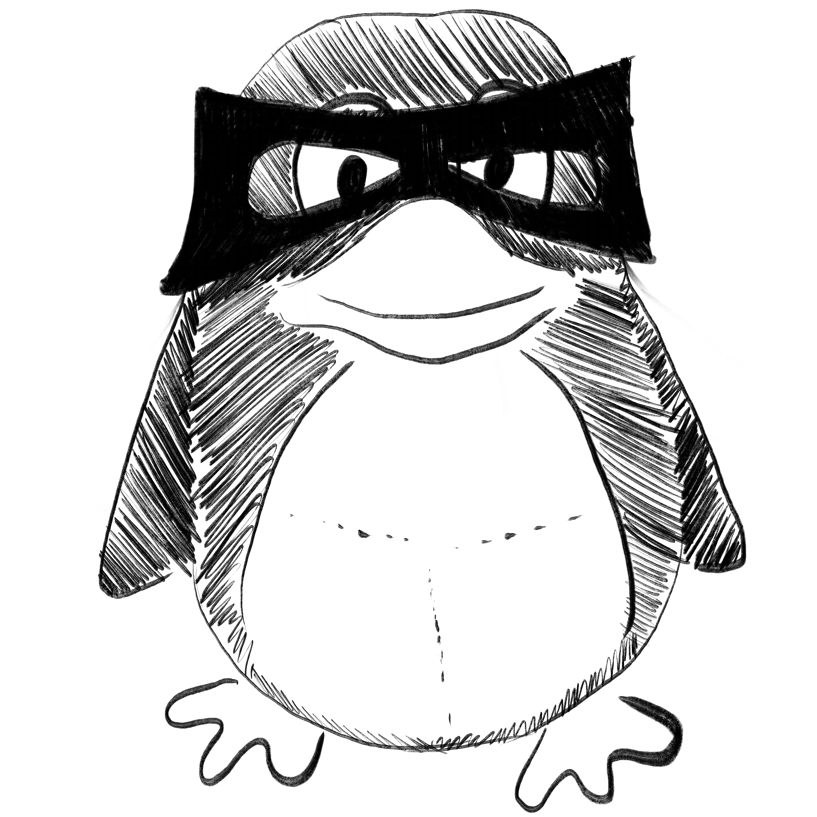
Longwave infrared multispectral image sensor system using aluminum-germanium plasmonic filter arrays
ArXiv Preprint
Noor E Karishma Shaik, Bryce Widdicombe, Dechuan Sun, Sam E John, Dongryeol Ryu, Ampalavanapillai Nirmalathas, Ranjith R Unnithan
2023-03-03
Full-length 16S rRNA gene sequencing and machine learning reveal the bacterial composition of inhalable particles from two different breeding stages in a piggery.
In Ecotoxicology and environmental safety ; h5-index 67.0
Peng Siyi, Luo Min, Long Dingbiao, Liu Zuohua, Tan Qiong, Huang Ping, Shen Jie, Pu Shihua
2023-Feb-28
Bacteria morphology, Full-length 16 S rRNA, Inhalable particles, Piggery, Potential source
Weekly Summary
Receive a weekly summary and discussion of the top papers of the week by leading researchers in the field.