Receive a weekly summary and discussion of the top papers of the week by leading researchers in the field.
Plasma metabolites with mechanistic and clinical links to the neurovascular disease cavernous angioma.
In Communications medicine
BACKGROUND :
METHODS :
RESULTS :
CONCLUSIONS :
Srinath Abhinav, Xie Bingqing, Li Ying, Sone Je Yeong, Romanos Sharbel, Chen Chang, Sharma Anukriti, Polster Sean, Dorrestein Pieter C, Weldon Kelly C, DeBiasse Dorothy, Moore Thomas, Lightle Rhonda, Koskimäki Janne, Zhang Dongdong, Stadnik Agnieszka, Piedad Kristina, Hagan Matthew, Shkoukani Abdallah, Carrión-Penagos Julián, Bi Dehua, Shen Le, Shenkar Robert, Ji Yuan, Sidebottom Ashley, Pamer Eric, Gilbert Jack A, Kahn Mark L, D’Souza Mark, Sulakhe Dinanath, Awad Issam A, Girard Romuald
2023-Mar-03
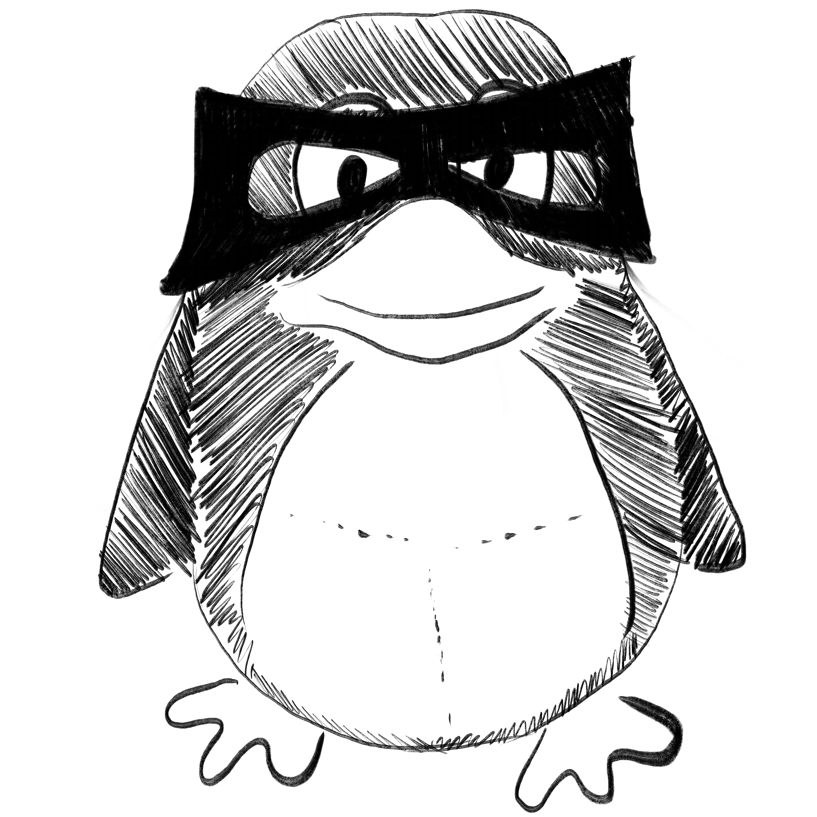
Consecutive multiscale feature learning-based image classification model.
In Scientific reports ; h5-index 158.0
Olimov Bekhzod, Subramanian Barathi, Ugli Rakhmonov Akhrorjon Akhmadjon, Kim Jea-Soo, Kim Jeonghong
2023-Mar-03
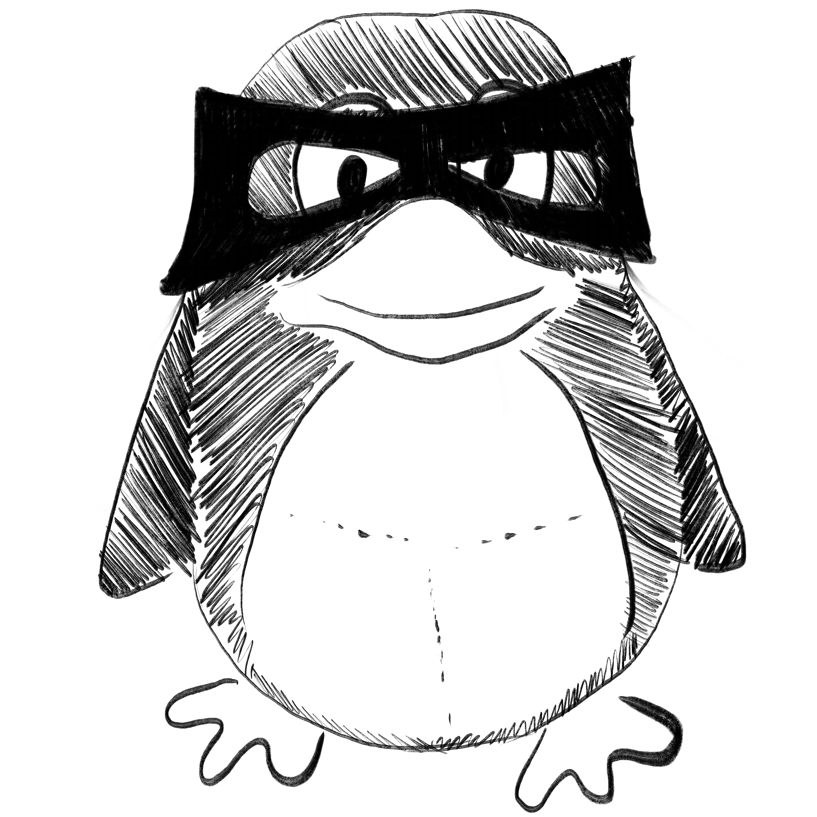
Machine learning identifies straightforward early warning rules for human Puumala hantavirus outbreaks.
In Scientific reports ; h5-index 158.0
Kazasidis Orestis, Jacob Jens
2023-Mar-03
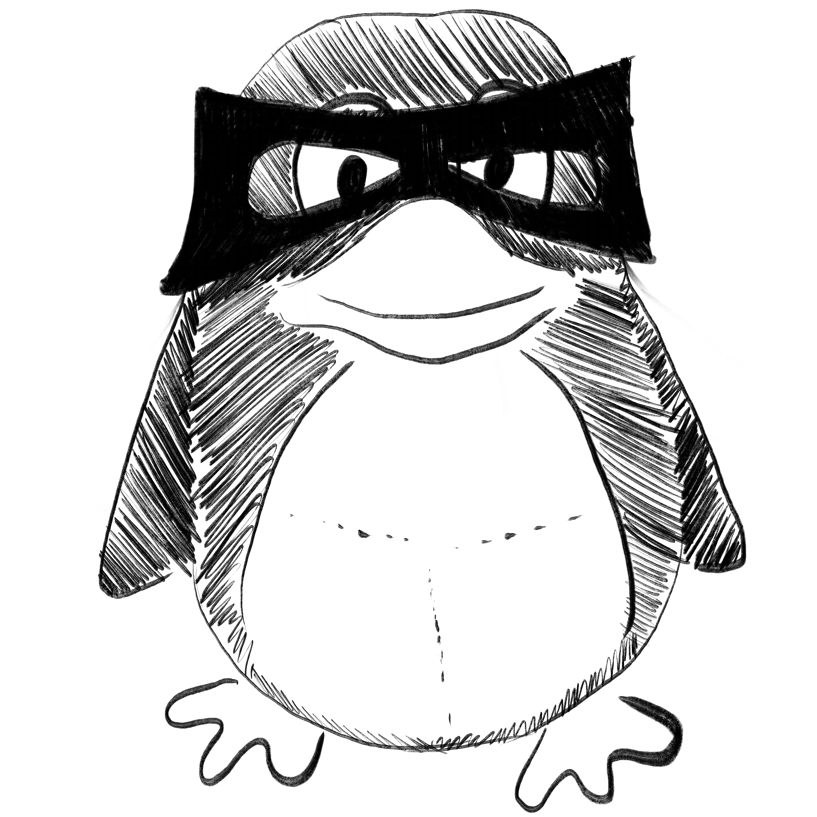
Machine learning classifiers for screening nonalcoholic fatty liver disease in general adults.
In Scientific reports ; h5-index 158.0
Qin Shenghua, Hou Xiaomin, Wen Yuan, Wang Chunqing, Tan Xiaxian, Tian Hao, Ao Qingqing, Li Jingze, Chu Shuyuan
2023-Mar-03
Iodine maps derived from sparse-view kV-switching dual-energy CT equipped with a deep learning reconstruction for diagnosis of hepatocellular carcinoma.
In Scientific reports ; h5-index 158.0
Narita Keigo, Nakamura Yuko, Higaki Toru, Kondo Shota, Honda Yukiko, Kawashita Ikuo, Mitani Hidenori, Fukumoto Wataru, Tani Chihiro, Chosa Keigo, Tatsugami Fuminari, Awai Kazuo
2023-Mar-03
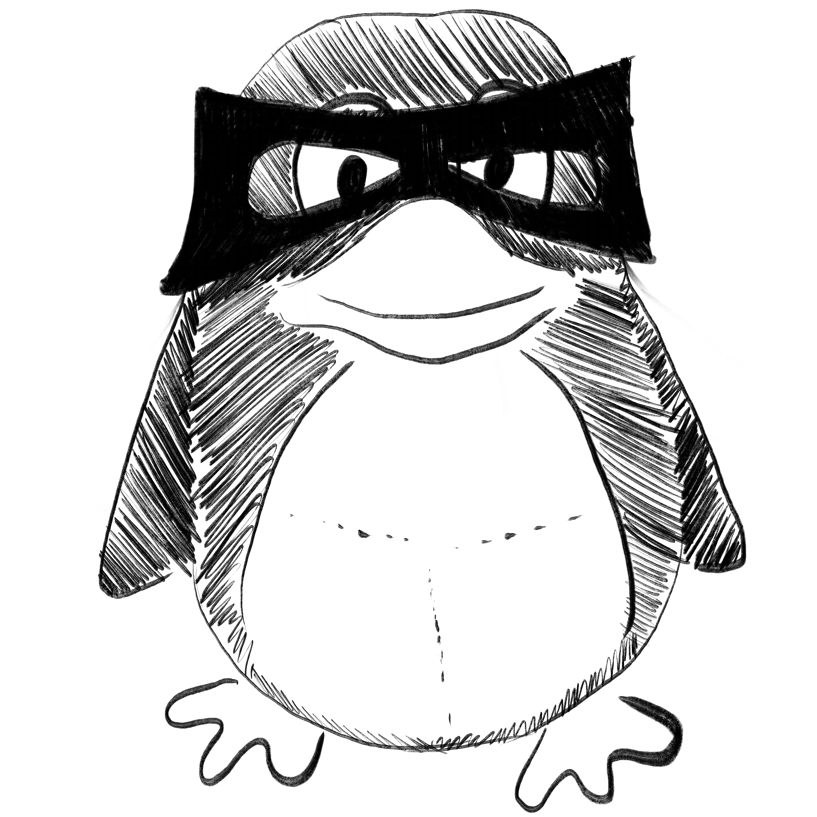
Current and future implications of artificial intelligence in colonoscopy.
In Annals of gastroenterology
Antonelli Giulio, Rizkala Tommy, Iacopini Federico, Hassan Cesare
2023
Artificial intelligence, adenoma detection rate, colonoscopy, machine learning, polyp detection
Weekly Summary
Receive a weekly summary and discussion of the top papers of the week by leading researchers in the field.