Receive a weekly summary and discussion of the top papers of the week by leading researchers in the field.
Interpretable Meta-learning of Multi-omics Data for Survival Analysis and Pathway Enrichment.
In Bioinformatics (Oxford, England)
MOTIVATION :
RESULTS :
AVAILABILITY :
SUPPLEMENTARY INFORMATION :
Cho Hyun Jae, Shu Mia, Bekiranov Stefan, Zang Chongzhi, Zhang Aidong
2023-Mar-02
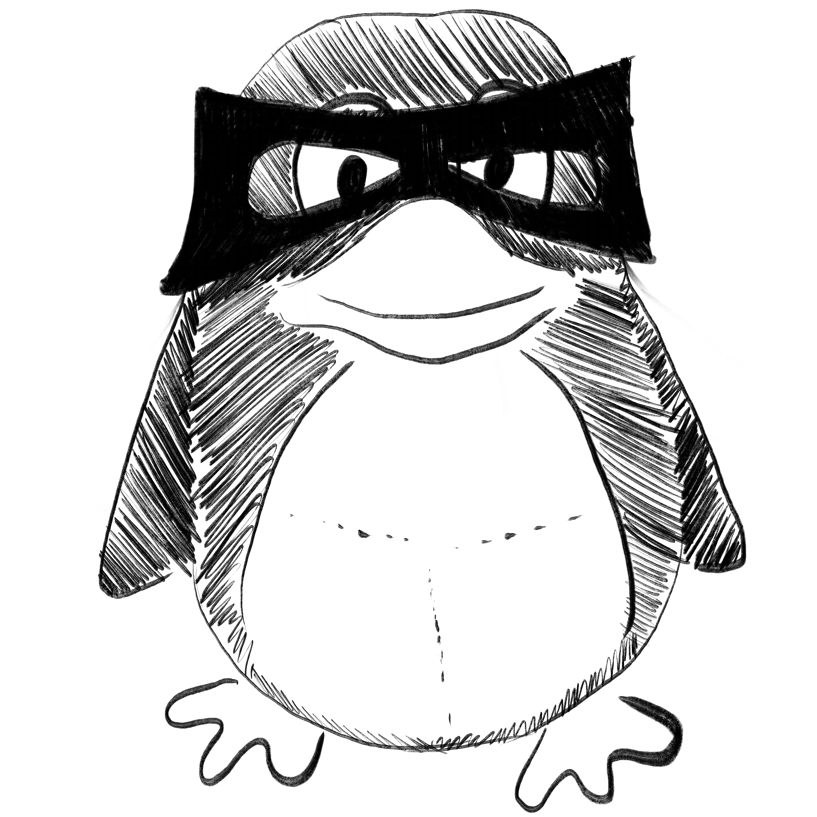
The future of automated infection detection: Innovation to transform practice (Part III/III).
In Antimicrobial stewardship & healthcare epidemiology : ASHE
Branch-Elliman Westyn, Sundermann Alexander J, Wiens Jenna, Shenoy Erica S
2023
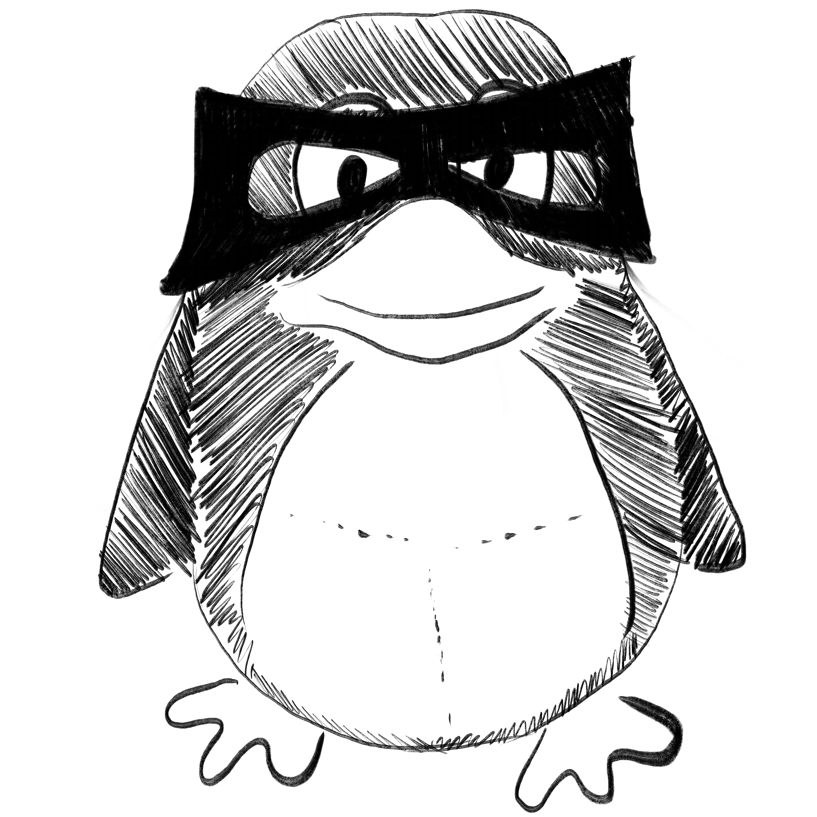
Assessing the Value of ChatGPT for Clinical Decision Support Optimization.
In medRxiv : the preprint server for health sciences
OBJECTIVE :
METHODS :
RESULTS :
CONCLUSION :
Liu Siru, Wright Aileen P, Patterson Barron L, Wanderer Jonathan P, Turer Robert W, Nelson Scott D, McCoy Allison B, Sittig Dean F, Wright Adam
2023-Feb-23
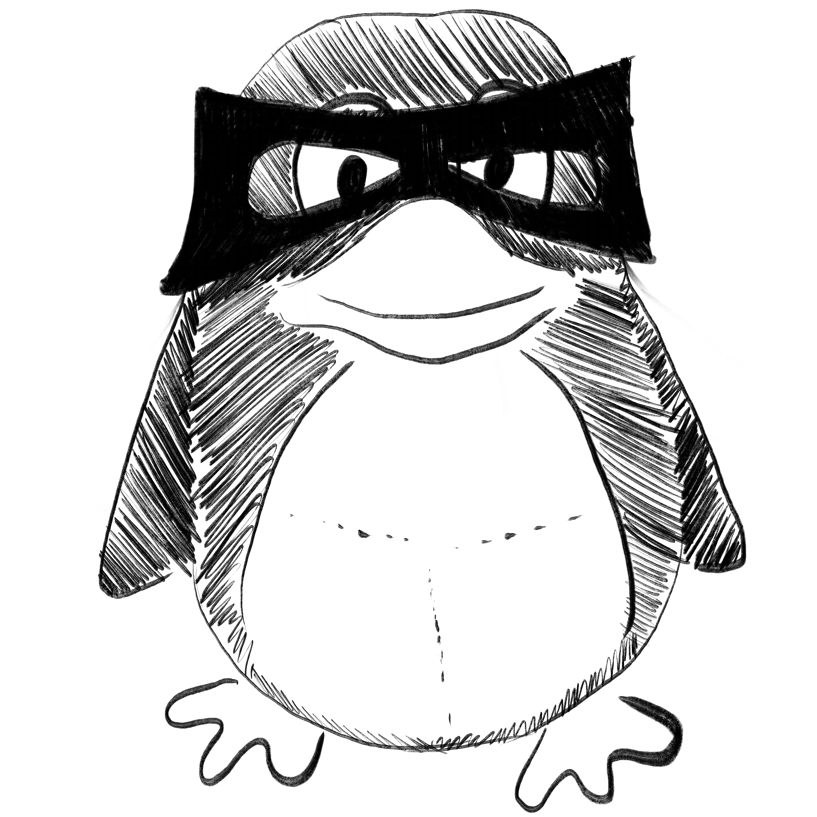
IntroUNET: identifying introgressed alleles via semantic segmentation.
In bioRxiv : the preprint server for biology
Ray Dylan D, Flagel Lex, Schrider Daniel R
2023-Feb-07
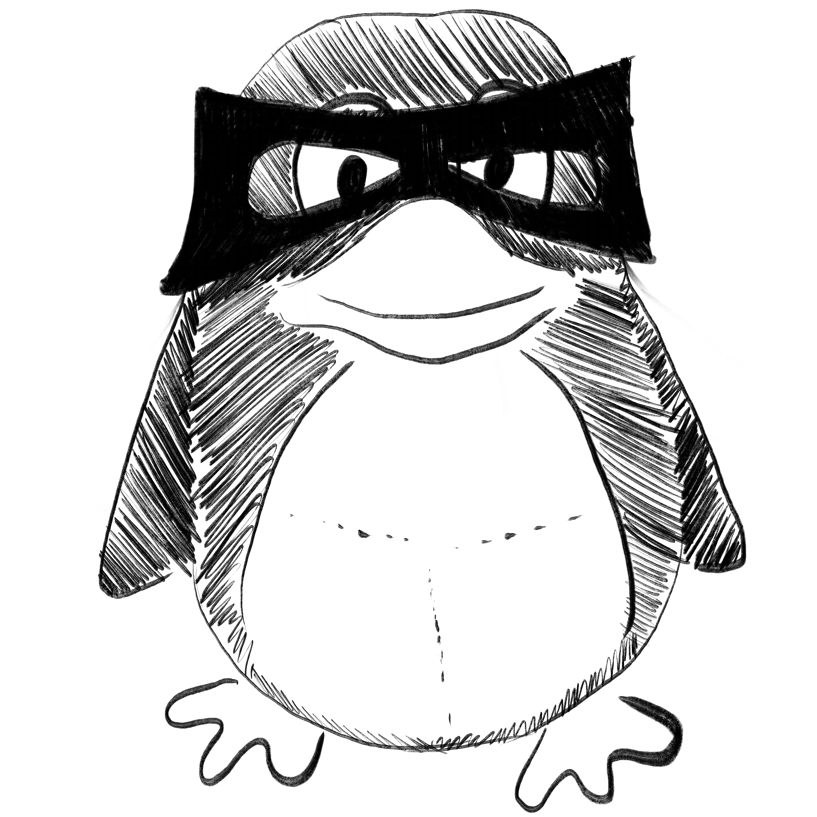
Patients with fibrosis from non-alcoholic steatohepatitis have heterogeneous intrahepatic macrophages and therapeutic targets.
In medRxiv : the preprint server for health sciences
BACKGROUND AND AIMS :
APPROACH & RESULTS :
CONCLUSIONS :
Saldarriaga Omar A, Krishnan Santhoshi, Wanninger Timothy G, Oneka Morgan, Rao Arvind, Bao Daniel, Arroyave Esteban, Gosnell Joseph, Kueht Michael, Moghe Akshata, Millian Daniel, Jiao Jingjing, Sanchez Jessica I, Spratt Heidi, Beretta Laura, Stevenson Heather L
2023-Feb-23
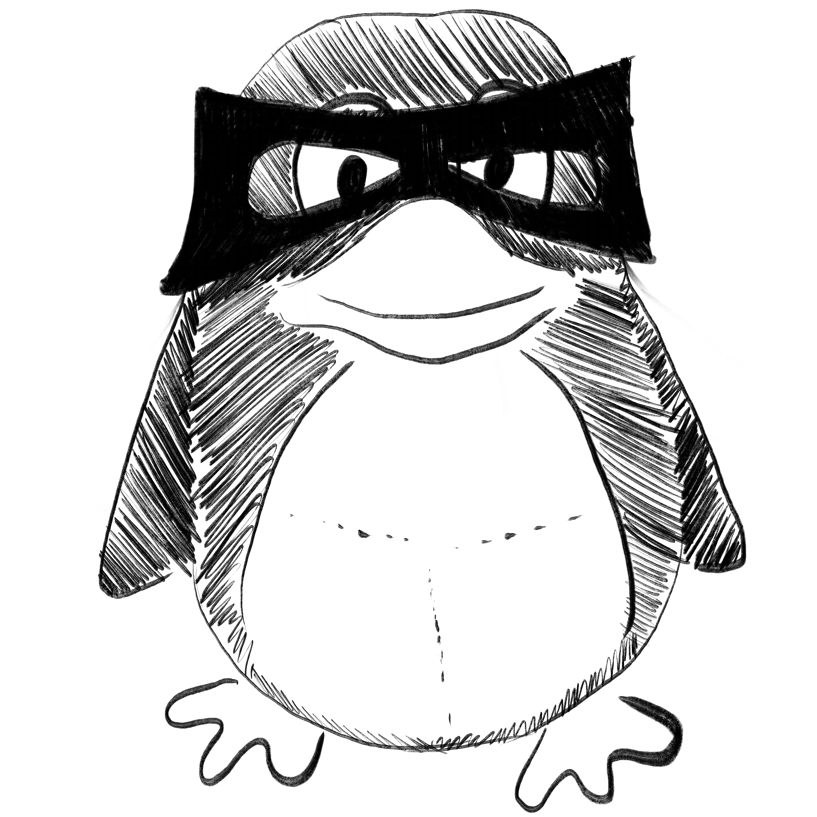
Retracted: Effectiveness of Artificial Intelligence Multimedia Courseware in Classroom Teaching Application.
In Applied bionics and biomechanics
And Biomechanics Applied Bionics
2023
Weekly Summary
Receive a weekly summary and discussion of the top papers of the week by leading researchers in the field.