Receive a weekly summary and discussion of the top papers of the week by leading researchers in the field.
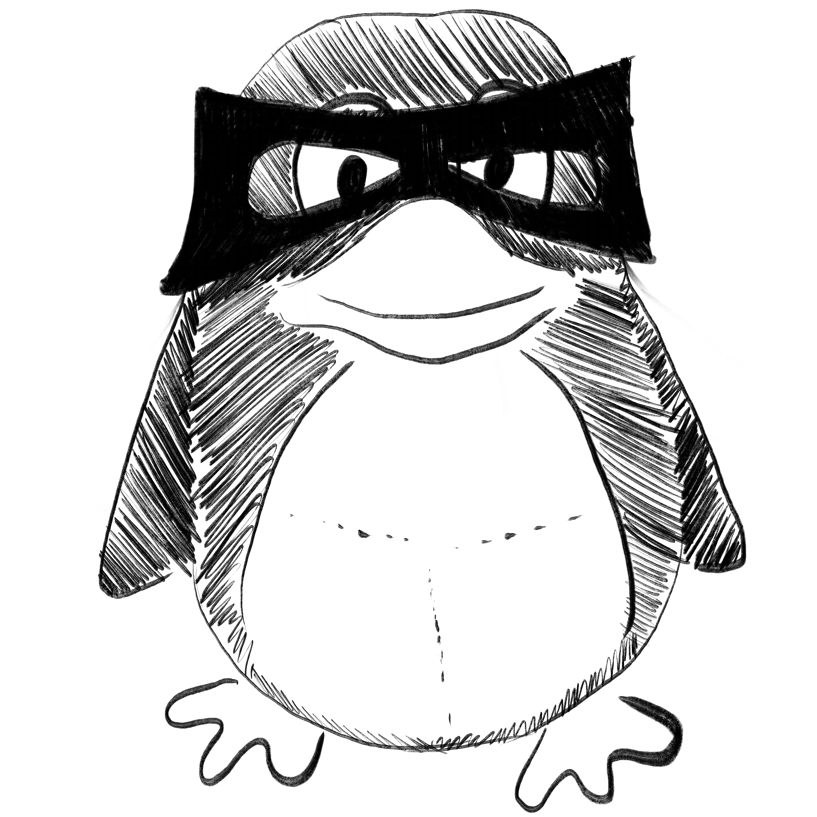
Diagnosis of childhood and adolescent growth hormone deficiency using transcriptomic data.
In Frontiers in endocrinology ; h5-index 55.0
BACKGROUND :
METHODS :
RESULTS :
CONCLUSION :
Garner Terence, Wangsaputra Ivan, Whatmore Andrew, Clayton Peter Ellis, Stevens Adam, Murray Philip George
2023
growth hormone, growth hormone deficiency, machine learning, random forest - ensemble classifier, transcriptome (RNA-seq)
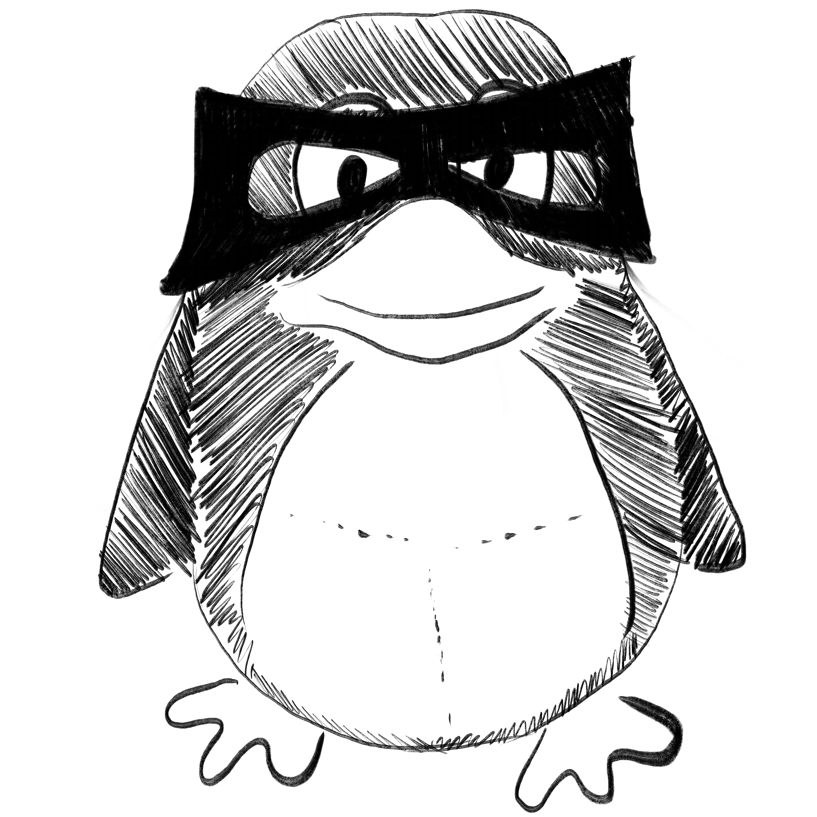
The effect of COVID-19 on self-reported safety incidents in aviation: An examination of the heterogeneous effects using causal machine learning.
In Journal of safety research
INTRODUCTION :
METHOD :
RESULTS :
PRACTICAL APPLICATIONS :
Choi Youngran, Gibson James R
2023-Feb
Aviation incursions/excursions, COVID-19, Heterogeneous treatment effects, Machine learning
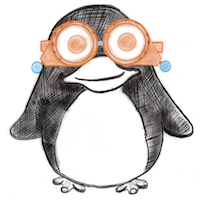
Integrating oculomics with genomics reveals imaging biomarkers for preventive and personalized prediction of arterial aneurysms.
In The EPMA journal
OBJECTIVE :
METHODS :
RESULTS :
CONCLUSION :
SUPPLEMENTARY INFORMATION :
Huang Yu, Li Cong, Shi Danli, Wang Huan, Shang Xianwen, Wang Wei, Zhang Xueli, Zhang Xiayin, Hu Yijun, Tang Shulin, Liu Shunming, Luo Songyuan, Zhao Ke, Mordi Ify R, Doney Alex S F, Yang Xiaohong, Yu Honghua, Li Xin, He Mingguang
2023-Mar
Aneurysm, Genetic risk scores, Imaging biomarker, Oculomics, Phenome-wide association analysis, Predictive preventive and personalized medicine (PPPM / 3PM), Retinal vascular features, Risk assessment
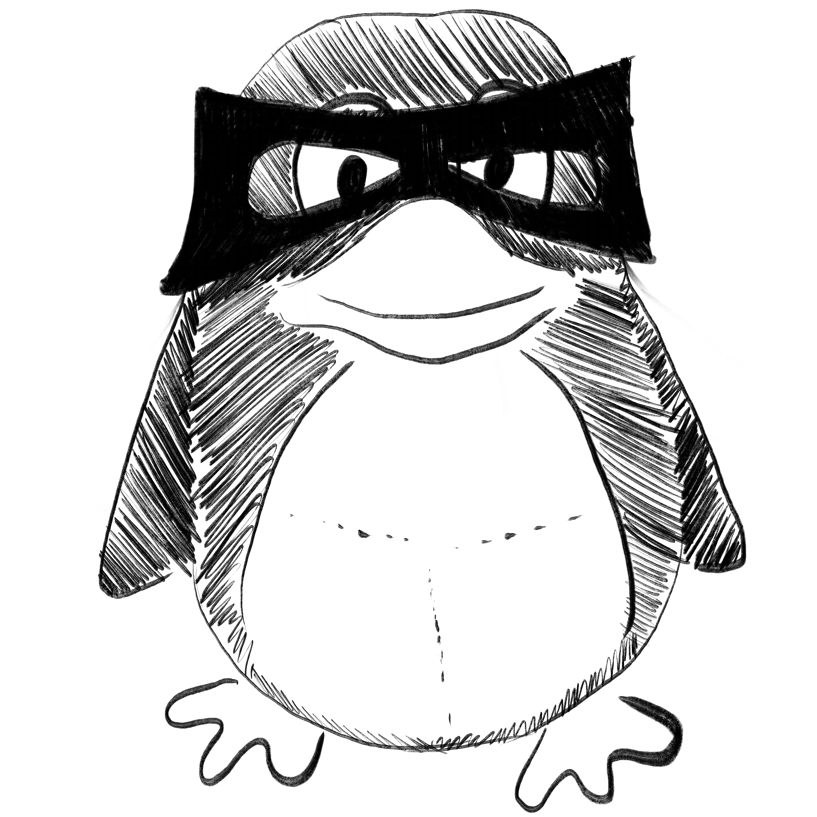
Supervised Classes, Unsupervised Mixing Proportions: Detection of Bots in a Likert-Type Questionnaire.
In Educational and psychological measurement
Ilagan Michael John, Falk Carl F
2023-Apr
Mahalanobis distance, aberrant responding, bots, machine learning, person-total correlation
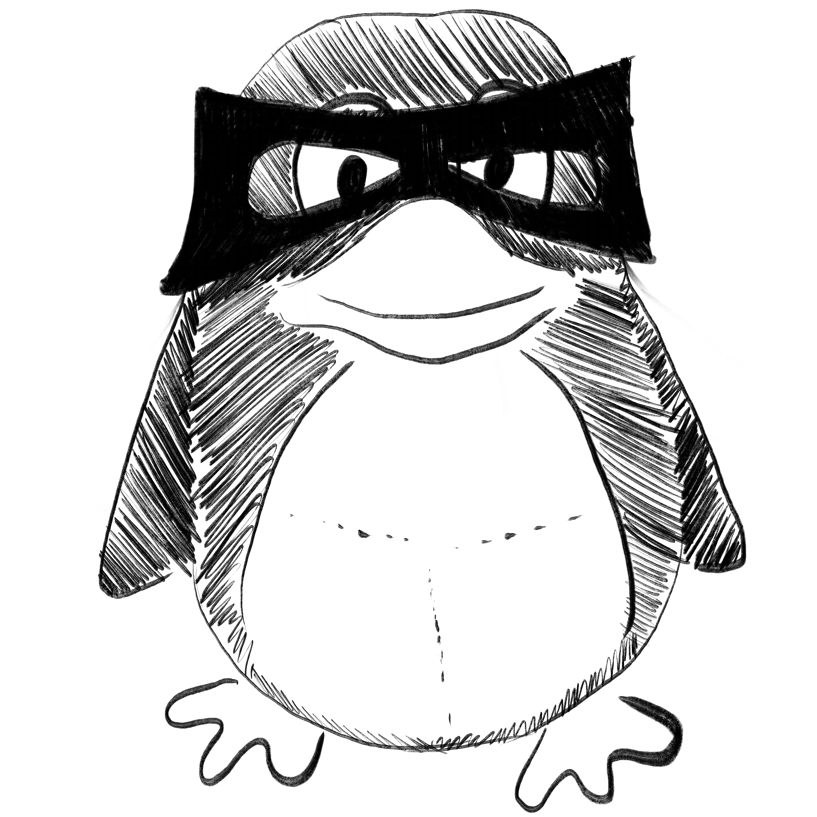
Is early or late biological maturation trigger obesity? A machine learning modeling research in Turkey boys and girls.
In Frontiers in nutrition
Gülü Mehmet, Yagin Fatma Hilal, Yapici Hakan, Irandoust Khadijeh, Dogan Ali Ahmet, Taheri Morteza, Szura Ewa, Barasinska Magdalena, Gabrys Tomasz
2023
adolescent, body mass index, childhood, noncommunicable diseases, overweight, puberty
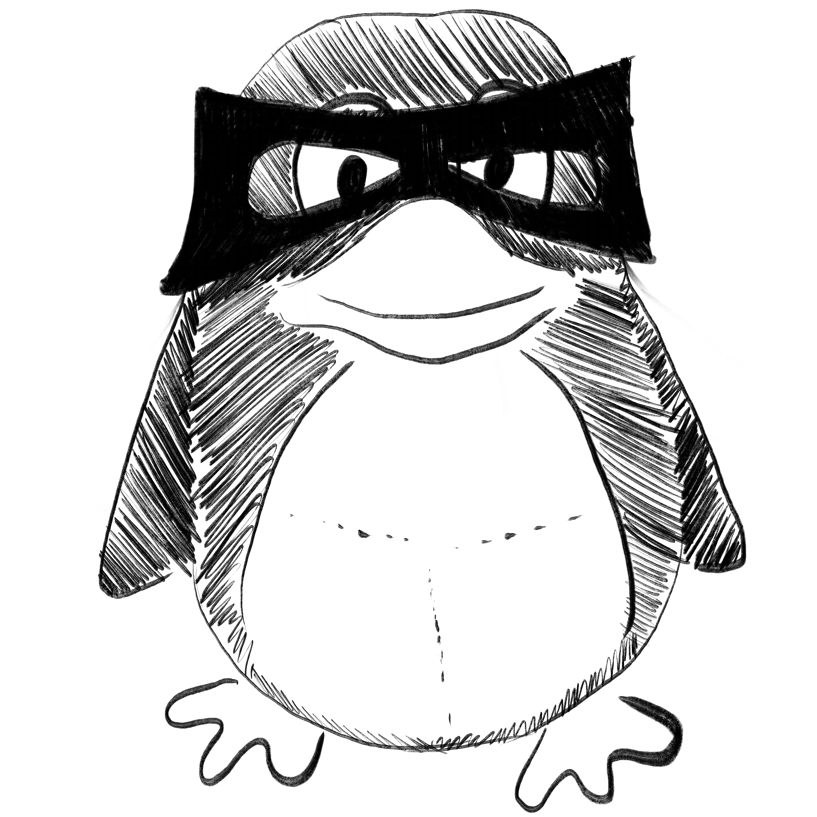
DeepBend: An interpretable model of DNA bendability.
In iScience
Khan Samin Rahman, Sakib Sadman, Rahman M Sohel, Samee Md Abul Hassan
2023-Feb-17
Biochemistry, Biological sciences, Genetics
Weekly Summary
Receive a weekly summary and discussion of the top papers of the week by leading researchers in the field.