Receive a weekly summary and discussion of the top papers of the week by leading researchers in the field.
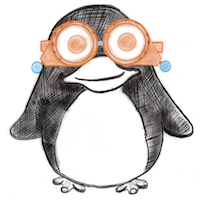
Association between vitamin D and myopia in adolescents and young adults: Evidence of national cross-sectional study.
In European journal of ophthalmology
PURPOSE :
METHODS :
RESULTS :
CONCLUSIONS :
Zhang Rui-Heng, Yang Qiong, Dong Li, Li Yi-Fan, Zhou Wen-Da, Wu Hao-Tian, Li He-Yan, Shao Lei, Zhang Chuan, Wang Ya-Xing, Wei Wen Bin
2023-Mar-03
National Health and Nutrition Examination Survey, myopia, vitamin D
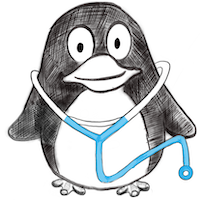
A Computational Approach in the Diagnostic Process of COVID-19: The Missing Link between the Laboratory and Emergency Department.
In Frontiers in bioscience (Landmark edition)
BACKGROUND :
METHODS :
RESULTS :
CONCLUSIONS :
Lanzilao Luisa, Mariniello Antonella, Polenzani Bianca, Aldinucci Alessandra, Nazerian Peiman, Prota Alessio, Grifoni Stefano, Tonietti Barbara, Neri Chiara, Turco Livia, Fanelli Alessandra, Amedei Amedeo, Stanghellini Elena
2023-Feb-22
COVID-19, automated classifiers, diagnosis, laboratory medicine, machine learning, “physicians gestalt”
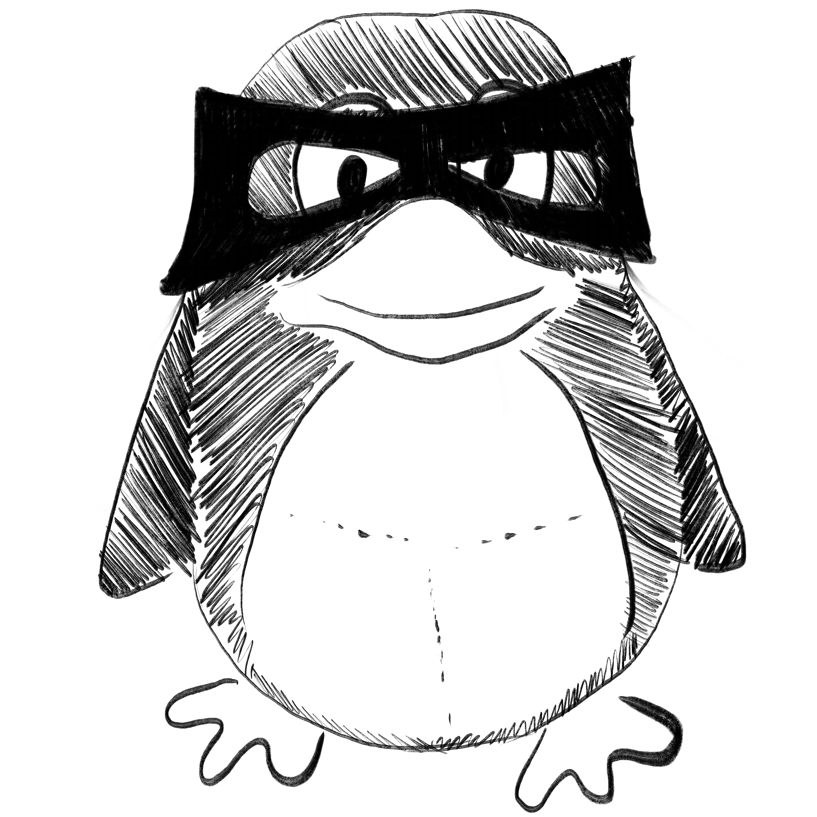
Research of intelligent reasoning system of Arabidopsis thaliana phenotype based on automated multi-task machine learning.
In Frontiers in plant science
Yuan Peisen, Xu Shuning, Zhai Zhaoyu, Xu Huanliang
2023
Arabidopsis thaliana, automated machine learning, cloud deployment, multi-task learning, plant phenotype reasoning
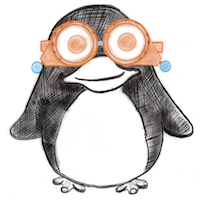
Reverse translation of artificial intelligence in glaucoma: Connecting basic science with clinical applications.
In Frontiers in ophthalmology
Ma Da, Pasquale Louis R, Girard Michaël J A, Leung Christopher K S, Jia Yali, Sarunic Marinko V, Sappington Rebecca M, Chan Kevin C
2023
artificial intelligence, deep learning, glaucoma, optical coherence tomography, reverse translation, transfer learning, visual field
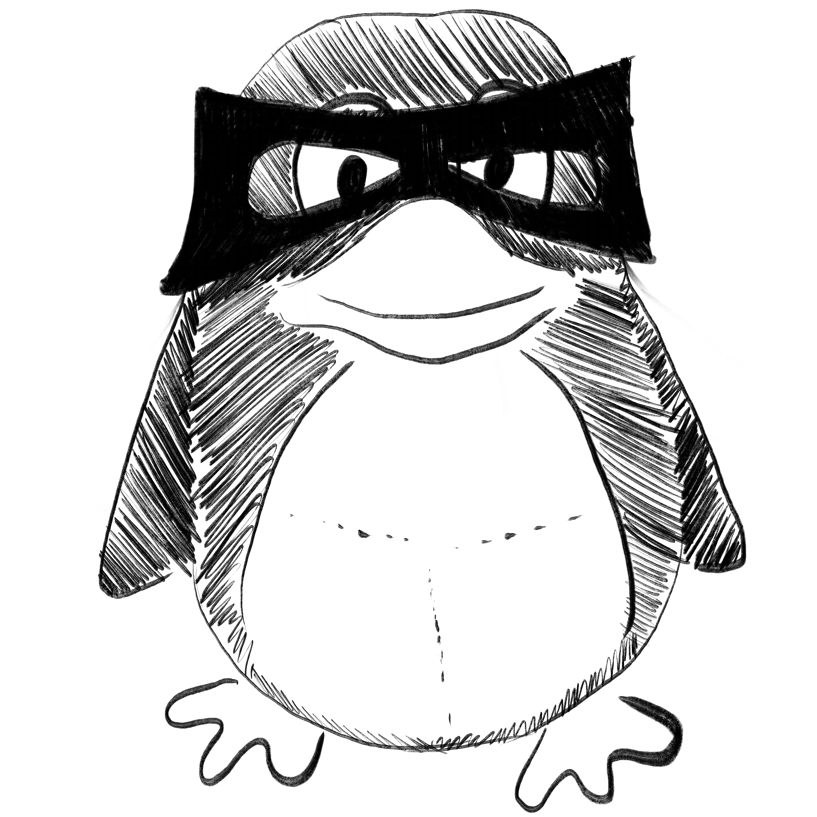
In-vivo processing of nanoassemblies: a neglected framework for recycling to bypass nanotoxicological therapeutics.
In Toxicology research
Kantak Maithili, Shende Pravin
2023-Feb
biotransformation, graphene, iron oxide nanoparticles, nanomaterials, polymeric nanoparticles, recycling
Bone age recognition based on mask R-CNN using xception regression model.
In Frontiers in physiology
Liu Zhi-Qiang, Hu Zi-Jian, Wu Tian-Qiong, Ye Geng-Xin, Tang Yu-Liang, Zeng Zi-Hua, Ouyang Zhong-Min, Li Yuan-Zhe
2023
Xception, bone age assessment, deep learning, hand bone X-ray images, mask R-CNN
Weekly Summary
Receive a weekly summary and discussion of the top papers of the week by leading researchers in the field.