Receive a weekly summary and discussion of the top papers of the week by leading researchers in the field.
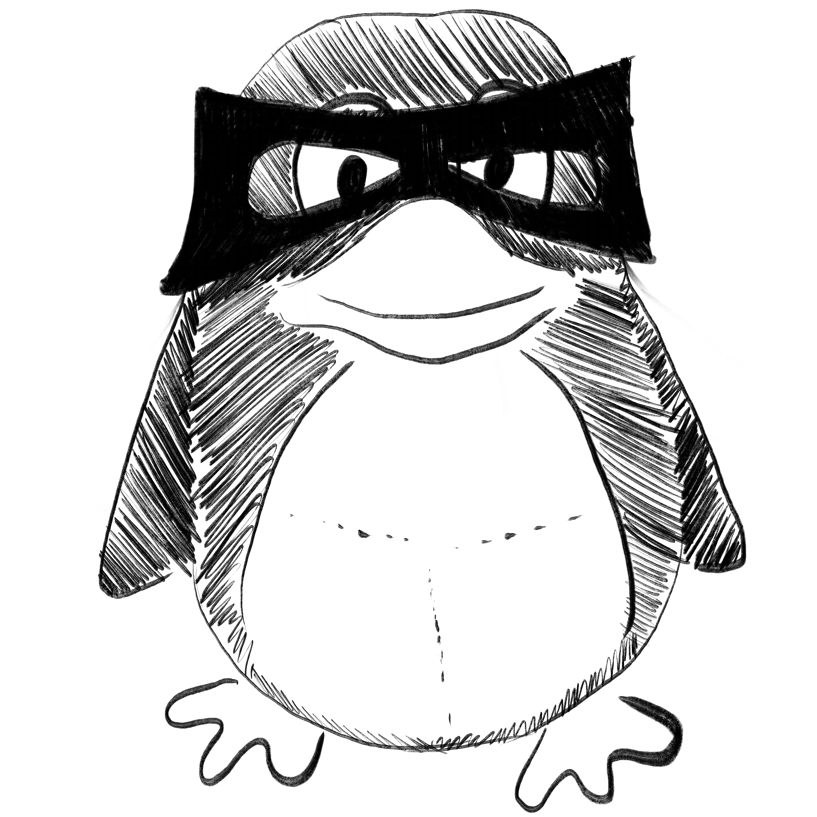
Combining pairwise structural similarity and deep learning interface contact prediction to estimate protein complex model accuracy in CASP15.
In bioRxiv : the preprint server for biology
Cheng Jianlin, Roy Raj Shekhor, Liu Jian, Giri Nabin, Guo Zhiye
2023-Mar-12
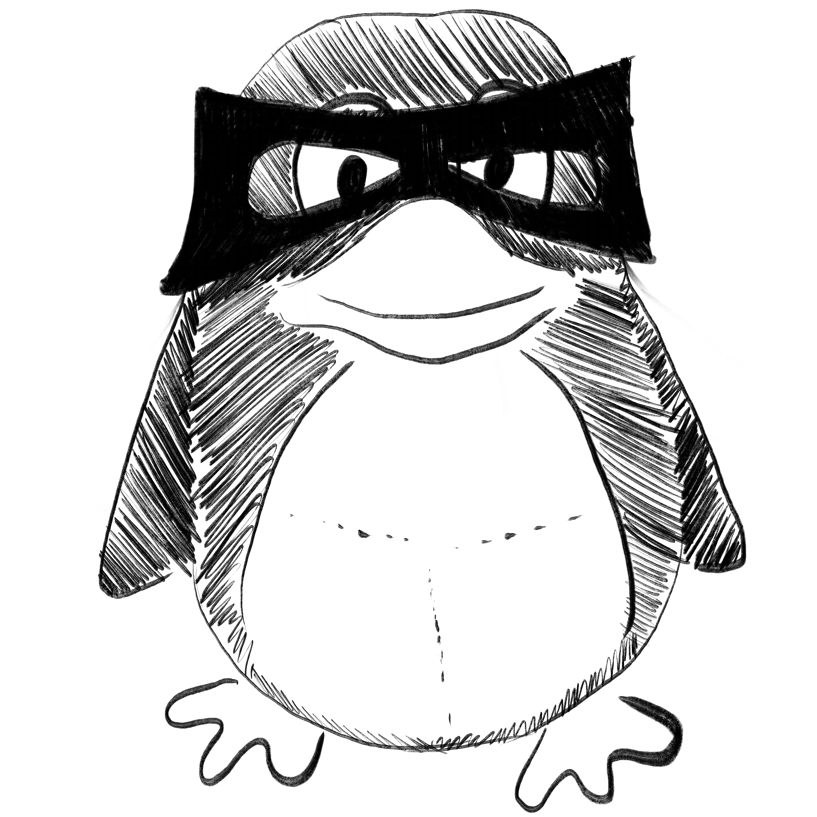
A multiscale functional map of somatic mutations in cancer integrating protein structure and network topology.
In bioRxiv : the preprint server for biology
Zhang Yingying, Leung Alden K, Qiu Tian, Li Le, Zhang Junke, Wierbowski Shayne, Booth James, Yu Haiyuan
2023-Mar-07
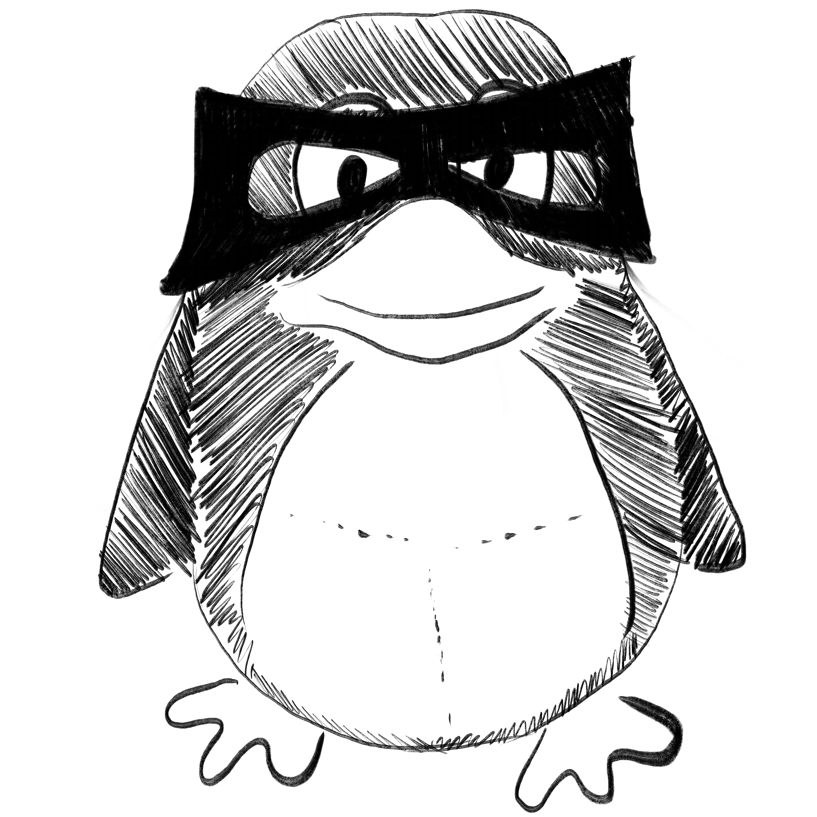
Fully-automated sarcopenia assessment in head and neck cancer: development and external validation of a deep learning pipeline.
In medRxiv : the preprint server for health sciences
PURPOSE :
MATERIALS AND METHODS :
RESULTS :
CONCLUSION :
SUMMARY STATEMENT :
Ye Zezhong, Saraf Anurag, Ravipati Yashwanth, Hoebers Frank, Zha Yining, Zapaishchykova Anna, Likitlersuang Jirapat, Tishler Roy B, Schoenfeld Jonathan D, Margalit Danielle N, Haddad Robert I, Mak Raymond H, Naser Mohamed, Wahid Kareem A, Sahlsten Jaakko, Jaskari Joel, Kaski Kimmo, Mäkitie Antti A, Fuller Clifton D, Aerts Hugo J W L, Kann Benjamin H
2023-Mar-06
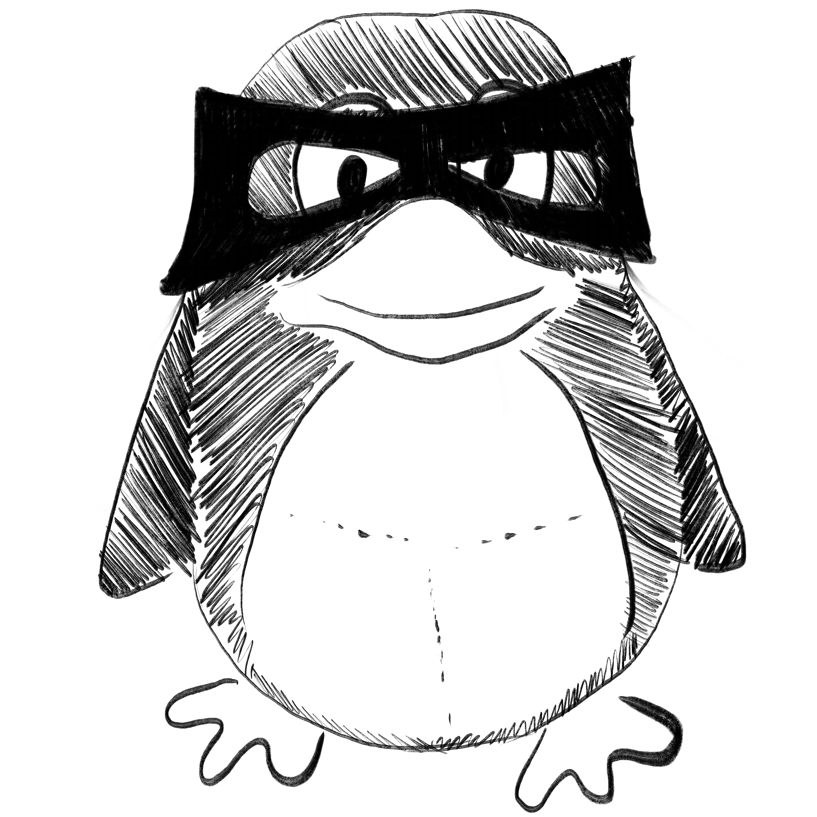
Microbiome Preterm Birth DREAM Challenge: Crowdsourcing Machine Learning Approaches to Advance Preterm Birth Research.
In medRxiv : the preprint server for health sciences
Golob Jonathan L, Oskotsky Tomiko T, Tang Alice S, Roldan Alennie, Chung Verena, Ha Connie W Y, Wong Ronald J, Flynn Kaitlin J, Parraga-Leo Antonio, Wibrand Camilla, Minot Samuel S, Andreoletti Gaia, Kosti Idit, Bletz Julie, Nelson Amber, Gao Jifan, Wei Zhoujingpeng, Chen Guanhua, Tang Zheng-Zheng, Novielli Pierfrancesco, Romano Donato, Pantaleo Ester, Amoroso Nicola, Monaco Alfonso, Vacca Mirco, Angelis Maria De, Bellotti Roberto, Tangaro Sabina, Kuntzleman Abigail, Bigcraft Isaac, Techtmann Stephen, Bae Daehun, Kim Eunyoung, Jeon Jongbum, Joe Soobok, Theis Kevin R, Ng Sherrianne, Lee Li Yun S, Bennett Phillip R, MacIntyre David A, Stolovitzky Gustavo, Lynch Susan V, Albrecht Jake, Gomez-Lopez Nardhy, Romero Roberto, Stevenson David K, Aghaeepour Nima, Tarca Adi L, Costello James C, Sirota Marina
2023-Mar-09
A Computed Tomography-Based Radiomics Analysis of Low-Energy Proximal Femur Fractures in the Elderly Patients.
In Current radiopharmaceuticals
INTRODUCTION :
METHODS :
RESULTS :
CONCLUSION :
Mohammadi Seyed Mohammad, Moniri Samir, Mohammadhoseini Payam, Hanafi Mohammad Ghasem, Farasat Maryam, Cheki Mohsen
2023-Mar-21
Radiomics, computed tomography, low-energy fracture, machine learning, osteoporosis, proximal femur
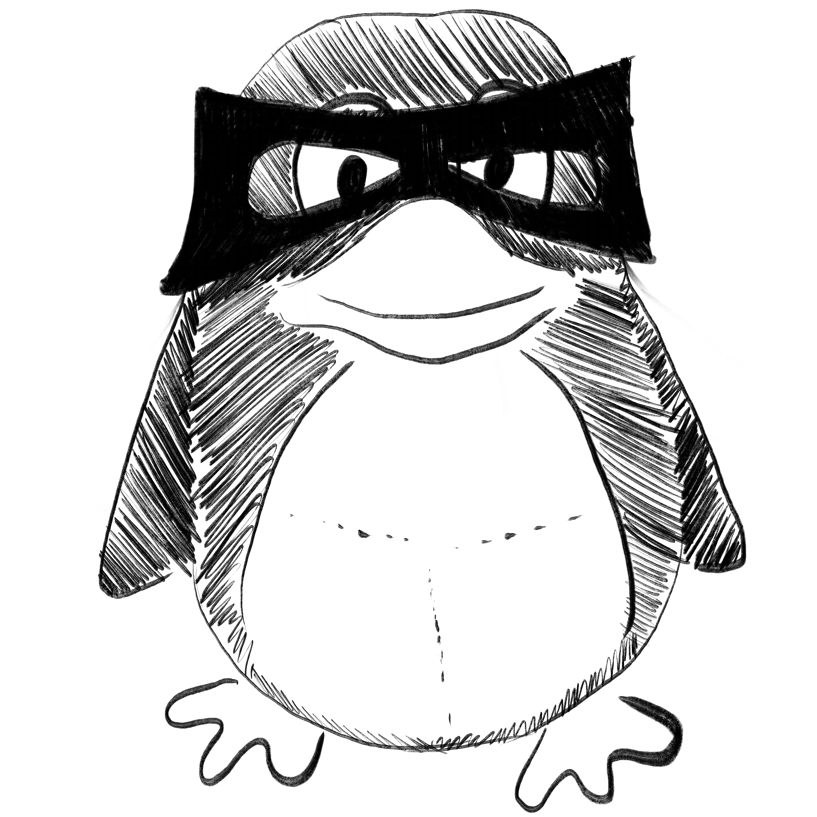
COVID-19 diagnosis: A comprehensive review of pre-trained deep learning models based on feature extraction algorithm.
In Results in engineering
Poola Rahul Gowtham, Pl Lahari, Y Siva Sankar
2023-Jun
Boundary tracing, Covid diagnosis, Deep transfer-learning, Medical imaging, Neural network models and classifiers
Weekly Summary
Receive a weekly summary and discussion of the top papers of the week by leading researchers in the field.