Receive a weekly summary and discussion of the top papers of the week by leading researchers in the field.
Sex-Related Differences in Patients Characteristics, Risk Factors, and Symptomatology in Older Adults with Pulmonary Embolism: Findings from the SERIOUS-PE Study.
In Seminars in thrombosis and hemostasis ; h5-index 38.0
Bikdeli Behnood, Muriel Alfonso, Wang Yun, Piazza Gregory, Khairani Candrika D, Rosovsky Rachel P, Mehdipoor Ghazaleh, O’Donoghue Michelle L, Madridano Olga, Lopez-Saez Juan Bosco, Mellado Meritxell, Brasero Ana Maria Diaz, Grandone Elvira, Spagnolo Primavera A, Lu Yuan, Bertoletti Laurent, López-Jiménez Luciano, Núñez Manuel Jesús, Blanco-Molina Ángeles, Gerhard-Herman Marie, Goldhaber Samuel Z, Bates Shannon M, Jimenez David, Krumholz Harlan M, Monreal Manuel
2023-Mar-03
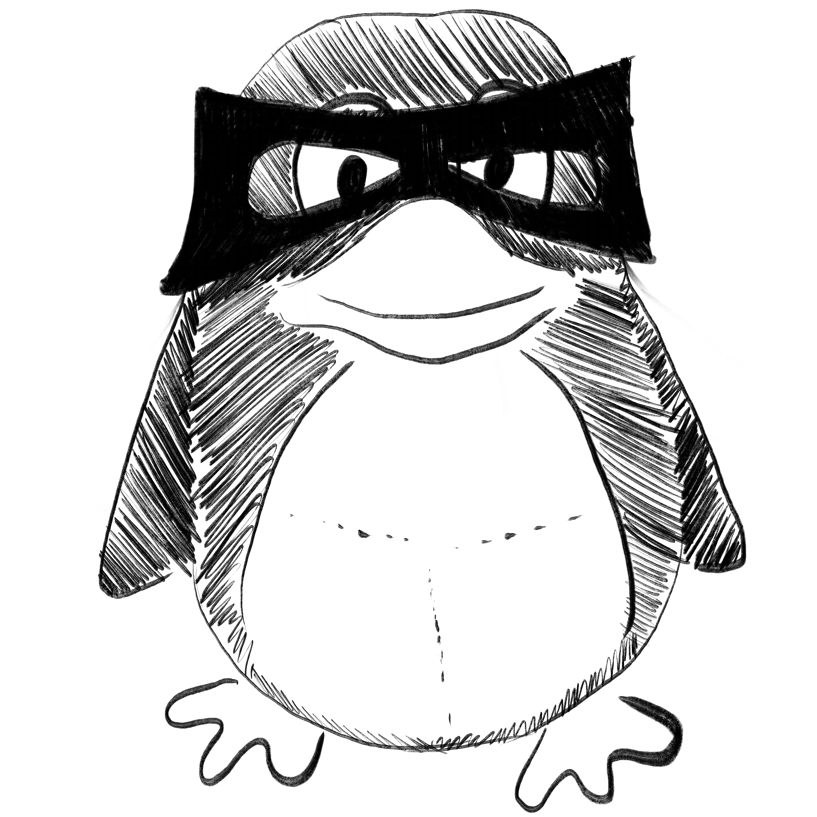
Progressive alterations in electrophysiological and epileptic network properties during the development of temporal lobe epilepsy in rats.
In Epilepsy & behavior : E&B
OBJECTIVE :
METHODS :
RESULTS :
SIGNIFICANCE :
Yang Yufang, Zhang Fang, Gao Xiang, Feng Linqing, Xu Kedi
2023-Mar-01
Electrophysiological properties, Granger causality, Functional connectivity, Neuromodulation, Temporal lobe epilepsy
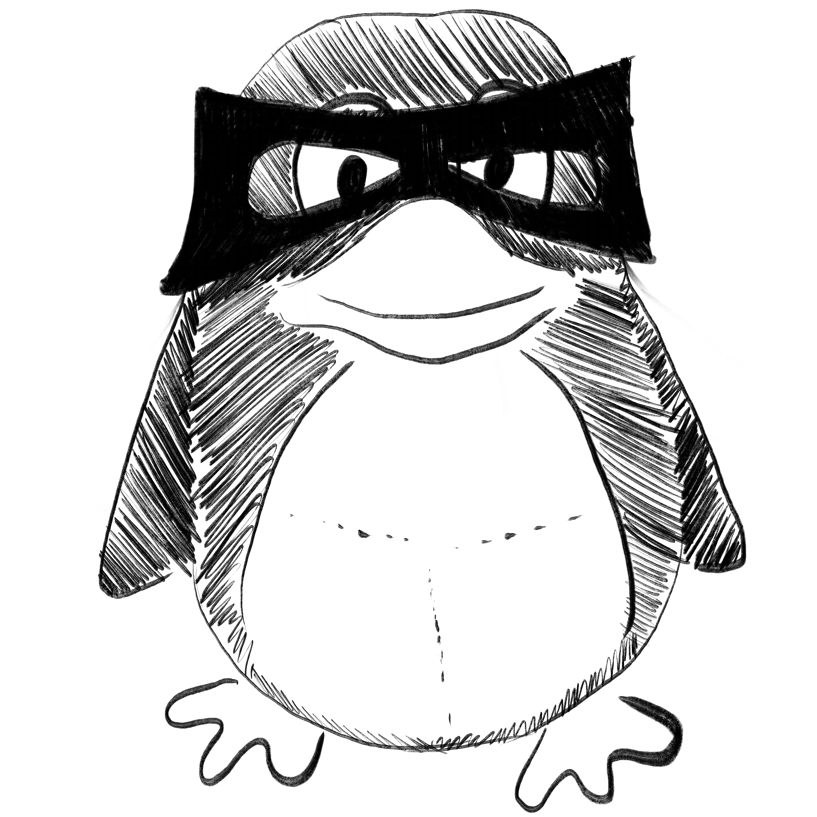
Multi-agent medical image segmentation: A survey.
In Computer methods and programs in biomedicine
Bennai Mohamed T, Guessoum Zahia, Mazouzi Smaine, Cormier Stéphane, Mezghiche Mohamed
2023-Feb-24
Image segmentation, Medical images, Multi-agent systems, Review, Survey
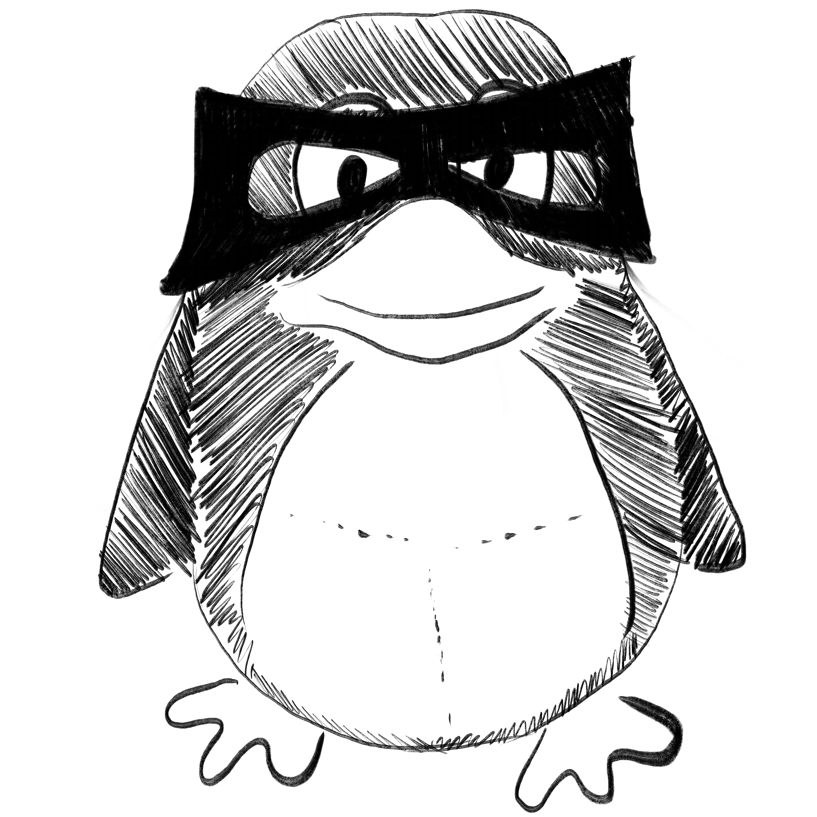
Relationship between physical activity and central sensitization in chronic low back pain: Insights from machine learning.
In Computer methods and programs in biomedicine
BACKGROUND AND OBJECTIVES :
METHODS :
RESULTS :
CONCLUSIONS :
Zheng Xiaoping, Reneman Michiel F, Preuper Rita Hr Schiphorst, Otten Egbert, Lamoth Claudine Jc
2023-Feb-20
Accelerometer, Avoidance-endurance model, Central sensitization, Chronic pain, Daily life, Hidden semi-Markov model, Low back pain, Physical activity
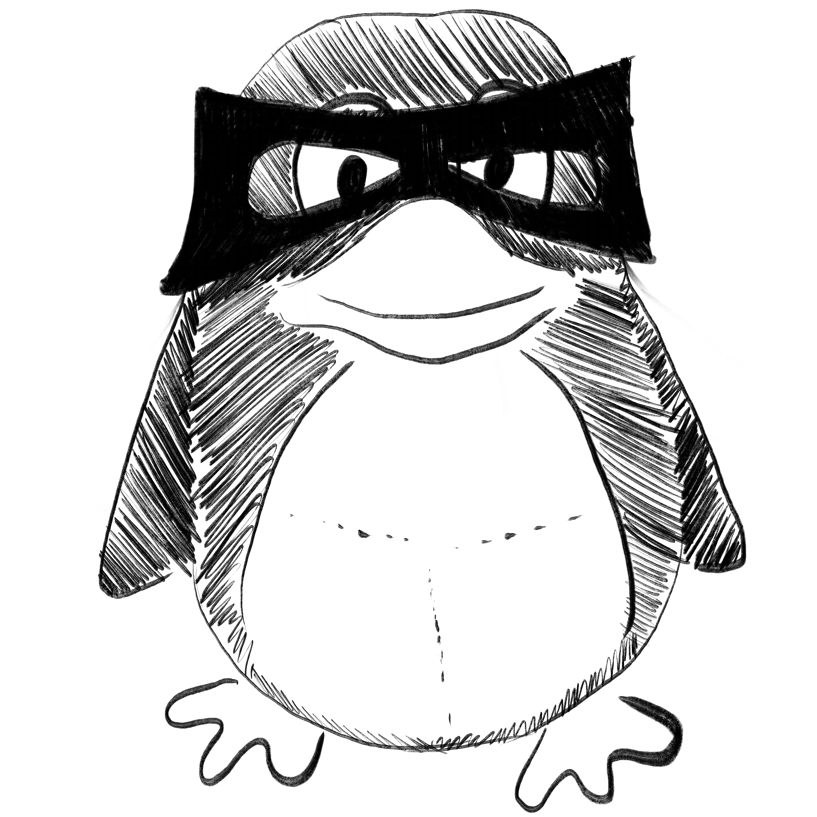
CARWatch - A smartphone application for improving the accuracy of cortisol awakening response sampling.
In Psychoneuroendocrinology ; h5-index 69.0
BACKGROUND :
METHODS :
RESULTS :
CONCLUSION :
Richer Robert, Abel Luca, Küderle Arne, Eskofier Bjoern M, Rohleder Nicolas
2023-Feb-24
Adherence, App, CAR, Saliva, Sampling accuracy, Smartphone
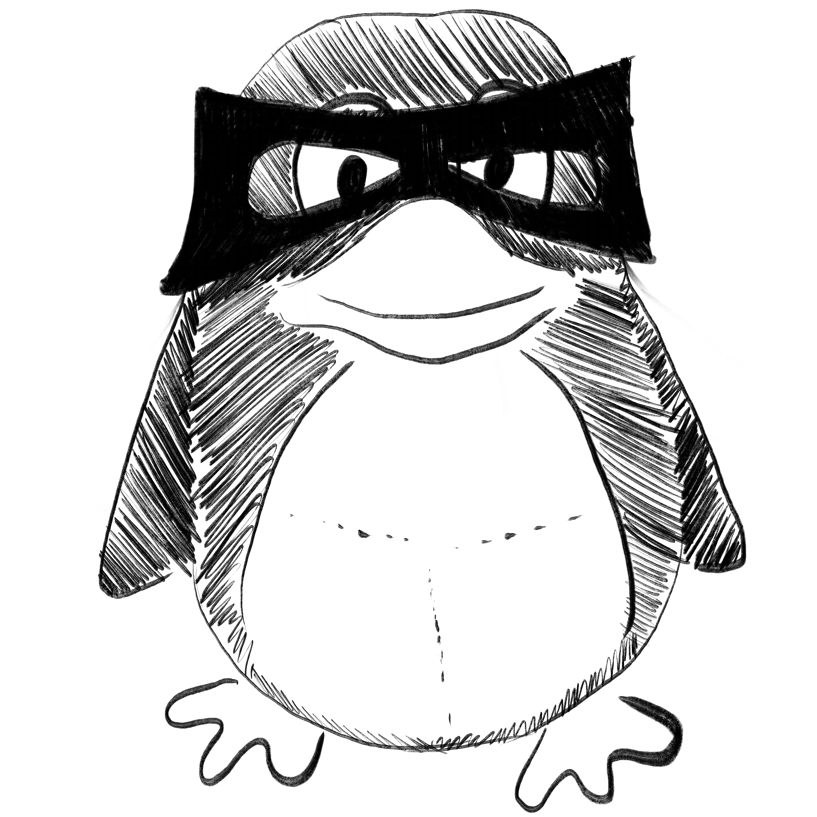
Revealing the importance of prenatal gut microbiome in offspring neurodevelopment in humans.
In EBioMedicine
BACKGROUND :
METHODS :
FINDINGS :
INTERPRETATION :
FUNDING :
Sun Zheng, Lee-Sarwar Kathleen, Kelly Rachel S, Lasky-Su Jessica A, Litonjua Augusto A, Weiss Scott T, Liu Yang-Yu
2023-Mar-01
Ages and stages questionnaire, Childhood neurodevelopment, Early-life gut microbiome, Maternal gut microbiome
Weekly Summary
Receive a weekly summary and discussion of the top papers of the week by leading researchers in the field.