Receive a weekly summary and discussion of the top papers of the week by leading researchers in the field.
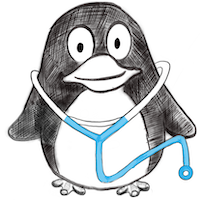
Effect of multimodal comprehensive communication skills training with video analysis by artificial intelligence for physicians on acute geriatric care: a mixed-methods study.
In BMJ open
OBJECTIVES :
DESIGN :
SETTING :
PARTICIPANTS :
INTERVENTIONS :
MAIN OUTCOME MEASURES :
RESULTS :
CONCLUSIONS :
TRIAL REGISTRATION NUMBER :
Kobayashi Masaki, Katayama Mitsuya, Hayashi Tomofumi, Hashiyama Takuhiro, Iyanagi Toshinori, Une Saki, Honda Miwako
2023-Mar-03
Dementia, GERIATRIC MEDICINE, INTERNAL MEDICINE, MEDICAL EDUCATION & TRAINING, QUALITATIVE RESEARCH
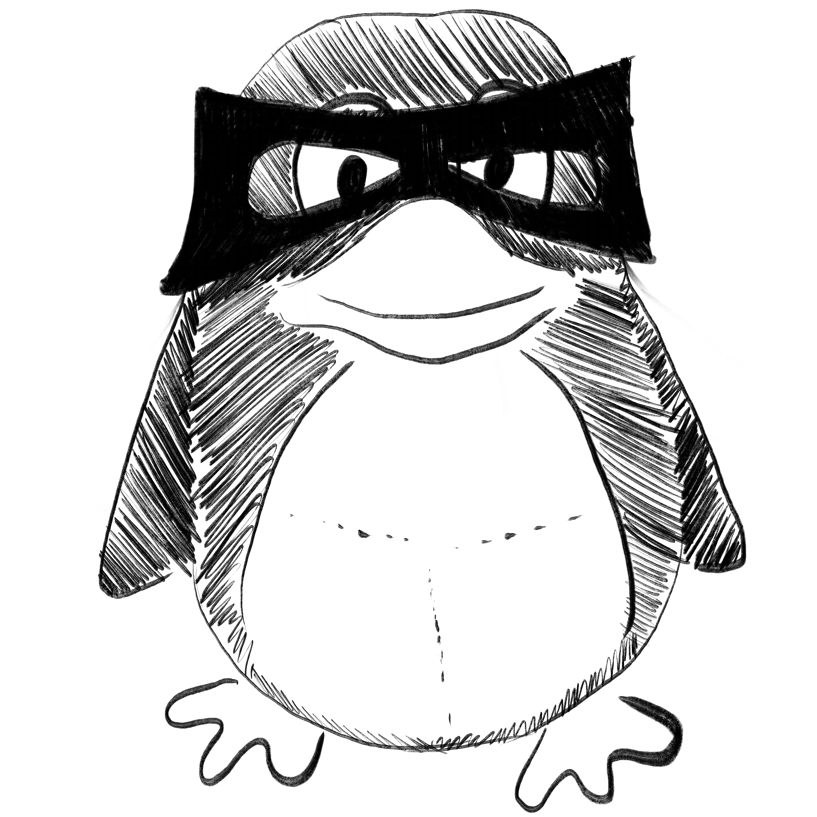
Automatic Intelligent System Using Medical of Things for Multiple Sclerosis Detection.
In Computational intelligence and neuroscience
Krishnamoorthy Sujatha, Zhang Yaxi, Kadry Seifedine, Khan Muhammad Attique, Alhaisoni Majed, Mustafa Nasser, Yu Weifeng, Alqahtani Abdullah
2023
Liquid biopsy-based protein biomarkers for risk prediction, early diagnosis and prognostication of cholangiocarcinoma.
In Journal of hepatology ; h5-index 119.0
BACKGROUND & AIMS :
METHODS :
RESULTS :
CONCLUSIONS :
IMPACT AND IMPLICATIONS :
Lapitz Ainhoa, Azkargorta Mikel, Milkiewicz Piotr, Olaizola Paula, Zhuravleva Ekaterina, Grimsrud Marit M, Schramm Christoph, Arbelaiz Ander, O’Rourke Colm J, La Casta Adelaida, Milkiewicz Malgorzata, Pastor Tania, Vesterhus Mette, Jimenez-Agüero Raul, Dill Michael T, Lamarca Angela, Valle Juan W, Macias Rocio I R, Izquierdo-Sanchez Laura, Castaño Ylenia Pérez, Caballero-Camino Francisco Javier, Riaño Ioana, Krawczyk Marcin, Ibarra Cesar, Bustamante Javier, Nova-Camacho Luiz M, Falcon-Perez Juan M, Elortza Felix, Perugorria Maria J, Andersen Jesper B, Bujanda Luis, Karlsen Tom H, Folseraas Trine, Rodrigues Pedro M, Banales Jesus M
2023-Mar-01
Cholangiocarcinoma, extracellular vesicles, liquid biopsy, mass spectrometry, primary sclerosing cholangitis, protein biomarkers, single-cell RNA-sequencing
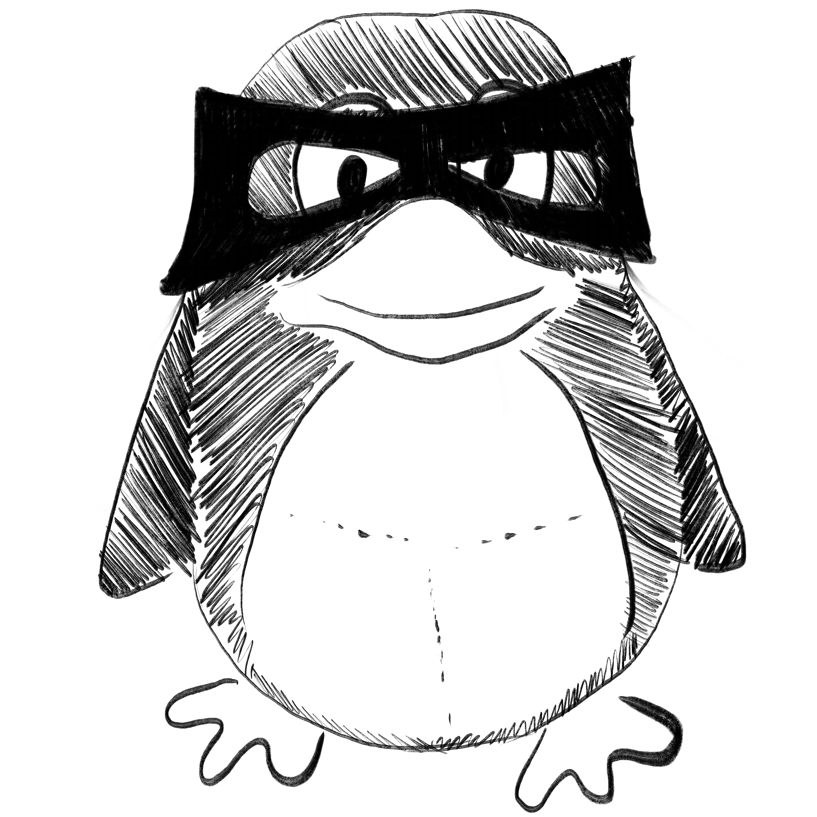
In silico approaches for prediction of anti-CRISPR proteins.
In Journal of molecular biology ; h5-index 65.0
Makarova Kira S, I Wolf Yuri, V Koonin Eugene
2023-Mar-01
anti-CRISPR proteins, comparative genomics, guilt-by-association, machine learning, self-targeting
Towards Artificial Intelligence to Multi-omics Characterization of Tumor Heterogeneity in Esophageal Cancer.
In Seminars in cancer biology
Li Junyu, Li Lin, You Peimeng, Wei Yiping, Xu Bin
2023-Mar-01
artificial intelligence, esophageal cancer, multi-omics, tumor heterogeneity, tumor microenvironment
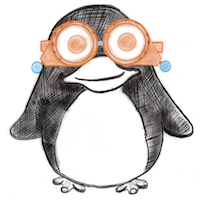
Differentiating Glaucomatous Optic Neuropathy from Non-Glaucomatous Optic Neuropathies Using Deep Learning Algorithms.
In American journal of ophthalmology ; h5-index 67.0
PURPOSE :
DESIGN :
METHOD :
RESULTS :
CONCLUSIONS :
Vali Mahsa, Mohammadi Massoud, Zarei Nasim, Samadi Melika, Atapour-Abarghouei Amir, Supakontanasan Wasu, Suwan Yanin, Subramanian Prem S, Miller Neil R, Kafieh Rahele, Fard Masoud Aghsaei
2023-Mar-01
Weekly Summary
Receive a weekly summary and discussion of the top papers of the week by leading researchers in the field.