Receive a weekly summary and discussion of the top papers of the week by leading researchers in the field.
Phonocardiogram transfer learning-based CatBoost model for diastolic dysfunction identification using multiple domain-specific deep feature fusion.
In Computers in biology and medicine
Zheng Yineng, Guo Xingming, Yang Yang, Wang Hui, Liao Kangla, Qin Jian
2023-Feb-20
Computer-aided diagnosis, Diastolic dysfunction detection, Heart sounds, Phonocardiogram, Transfer learning
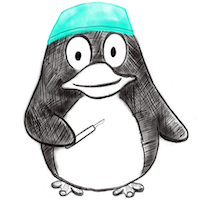
Automated Peripancreatic Vessel Segmentation and Labeling Based on Iterative Trunk Growth and Weakly Supervised Mechanism
ArXiv Preprint
Liwen Zou, Zhenghua Cai, Liang Mao, Ziwei Nie, Yudong Qiu, Xiaoping Yang
2023-03-06
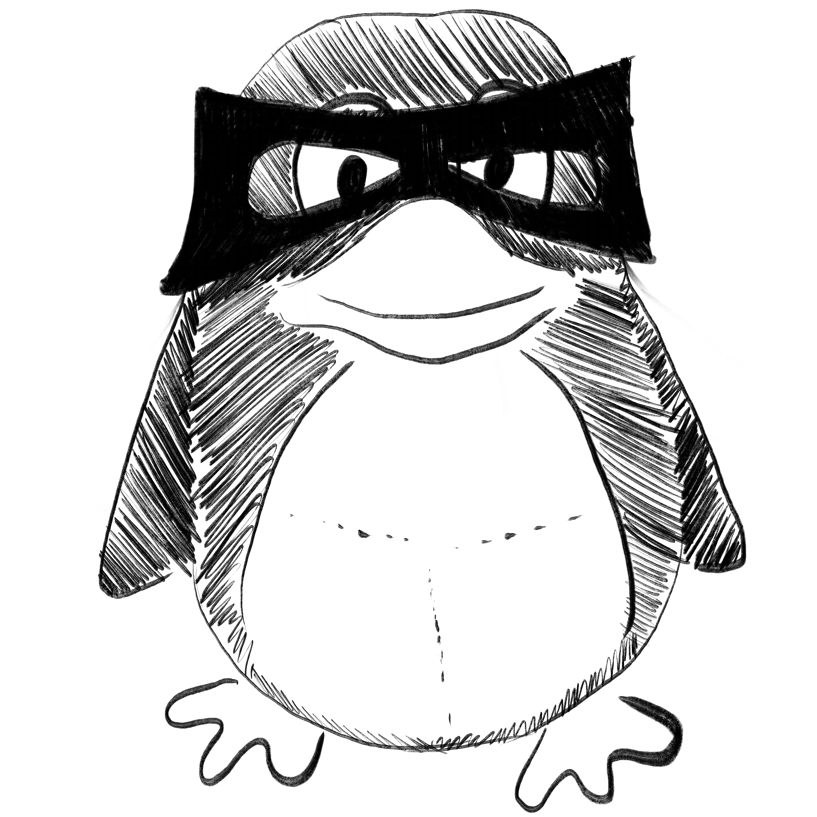
Epilepsy diagnosis using a clinical decision tool and artificially intelligent electroencephalography.
In Epilepsy & behavior : E&B
OBJECTIVE :
MATERIALS AND METHODS :
RESULTS :
SIGNIFICANCE :
McInnis Robert P, Ayub Muhammad Abubakar, Jing Jin, Halford Jonathan J, Mateen Farrah J, Brandon Westover M
2023-Mar-03
Decision tool, Diagnosis, EEG, Epilepsy
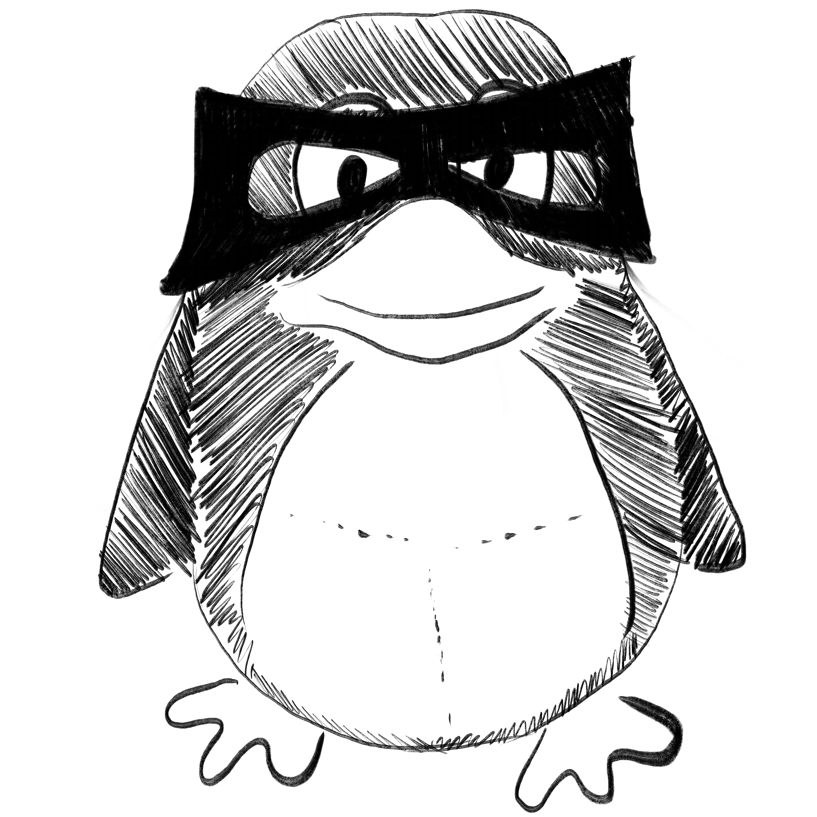
Cybersecurity of AI medical devices: risks, legislation, and challenges
ArXiv Preprint
Elisabetta Biasin, Erik Kamenjasevic, Kaspar Rosager Ludvigsen
2023-03-06
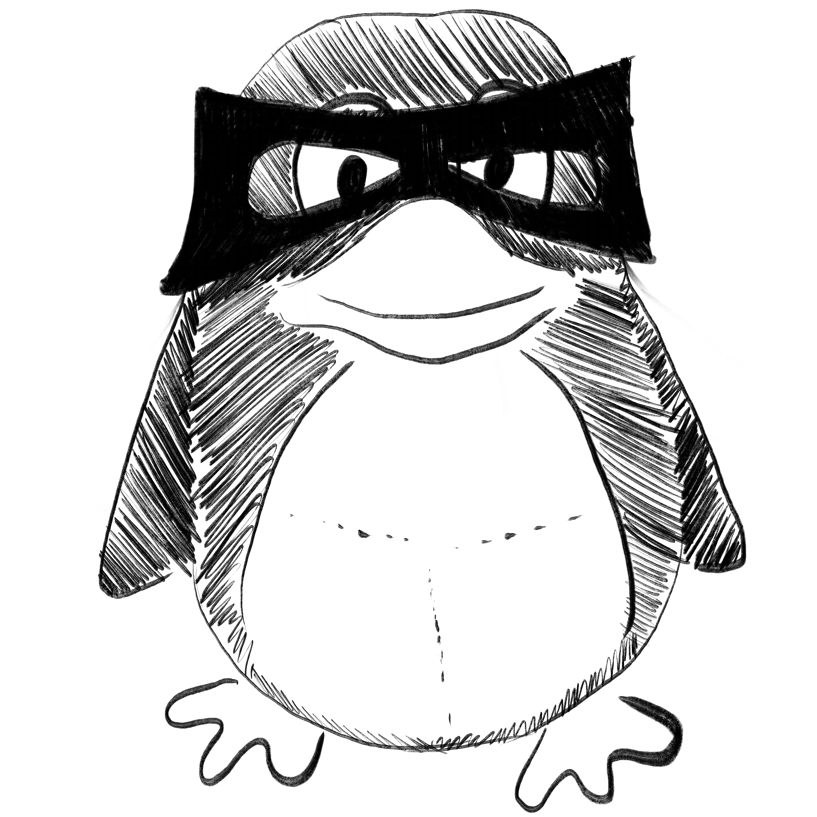
A Real-World Exploration into Clinical Outcomes of Direct Oral Anticoagulant Dosing Regimens in Morbidly Obese Patients Using Data-Driven Approaches.
In American journal of cardiovascular drugs : drugs, devices, and other interventions
INTRODUCTION :
METHOD :
RESULTS :
CONCLUSION :
Nwanosike Ezekwesiri Michael, Sunter Wendy, Ansari Muhammad Ayub, Merchant Hamid A, Conway Barbara, Hasan Syed Shahzad
2023-Mar-06
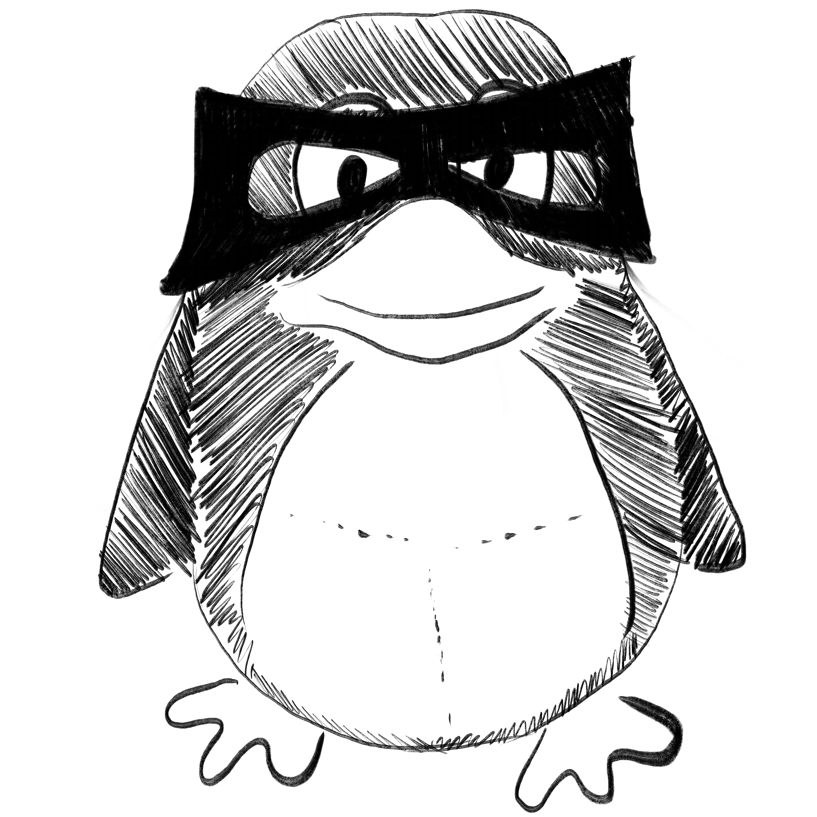
Applications and prospects of cryo-EM in drug discovery.
In Military Medical Research
Zhu Kong-Fu, Yuan Chuang, Du Yong-Ming, Sun Kai-Lei, Zhang Xiao-Kang, Vogel Horst, Jia Xu-Dong, Gao Yuan-Zhu, Zhang Qin-Fen, Wang Da-Ping, Zhang Hua-Wei
2023-Mar-06
Artificial intelligence (AI), Cryo-electron microscopy (cryo-EM), Drug discovery, Drug repurposing, Fragment-based drug discovery, Proteolysis targeting chimeras, Structure-based drug design
Weekly Summary
Receive a weekly summary and discussion of the top papers of the week by leading researchers in the field.