Receive a weekly summary and discussion of the top papers of the week by leading researchers in the field.
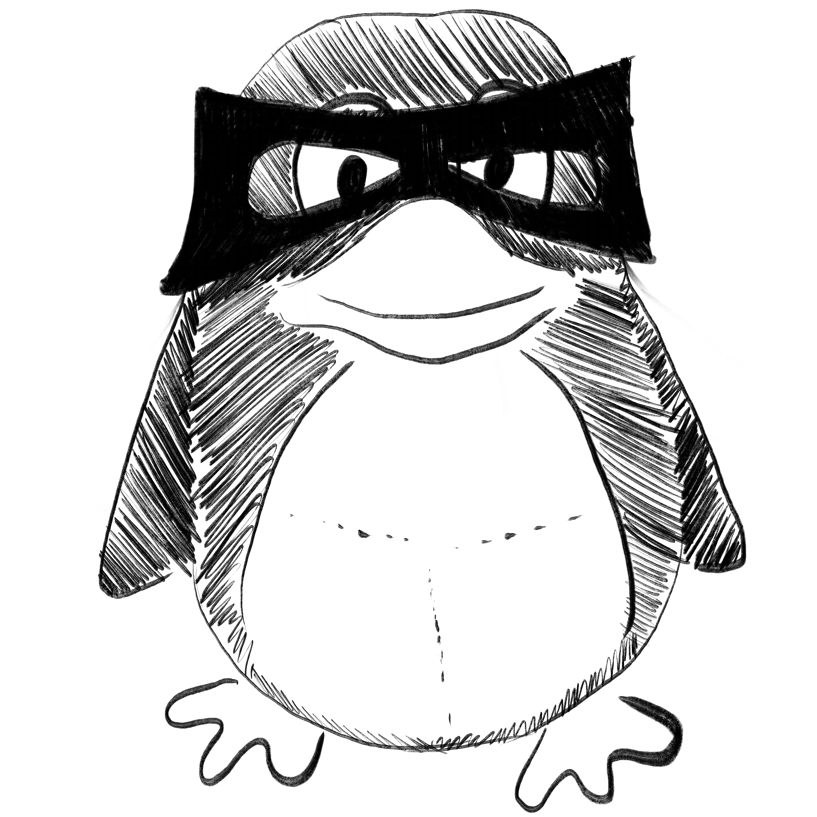
Argument-based human-AI collaboration for supporting behavior change to improve health.
In Frontiers in artificial intelligence
Kilic Kaan, Weck Saskia, Kampik Timotheus, Lindgren Helena
2023
Human-Centered Artificial Intelligence, argumentation schemes, behavior change, digital companion, formal argumentation dialogues, health promotion, user-modeling, value-based argumentation
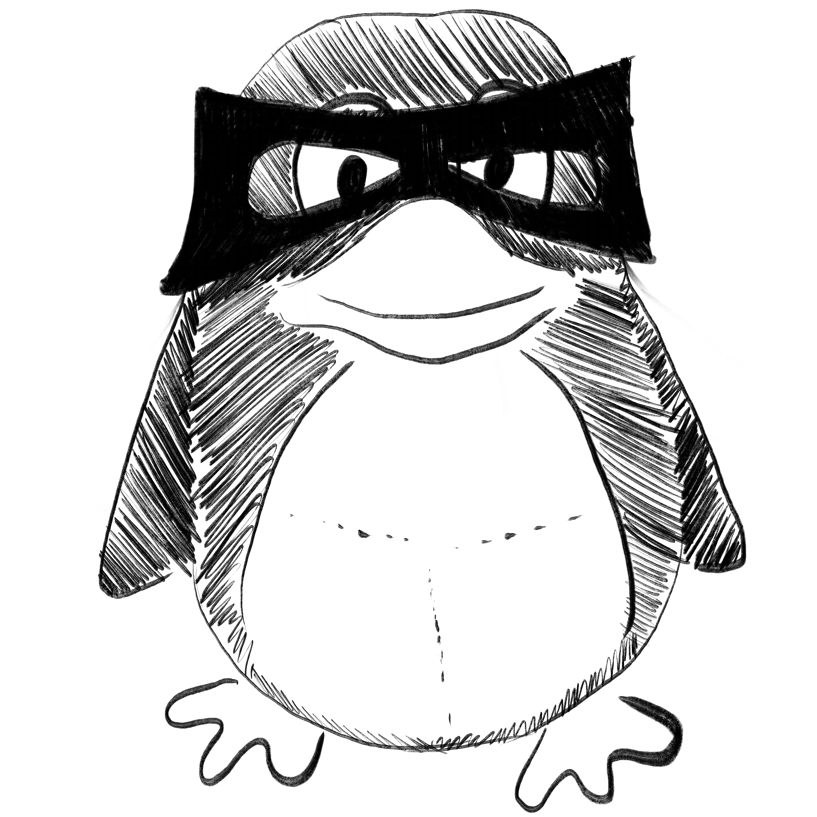
Data driven contagion risk management in low-income countries using machine learning applications with COVID-19 in South Asia.
In Scientific reports ; h5-index 158.0
Shonchoy Abu S, Mahzab Moogdho M, Mahmood Towhid I, Ali Manhal
2023-Mar-06
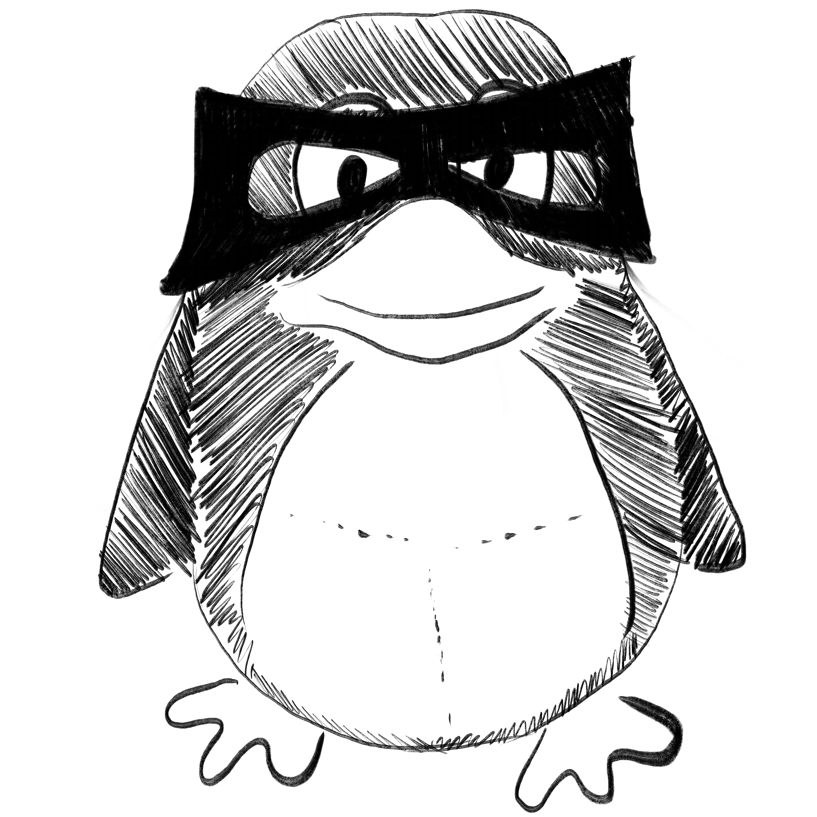
[Individualized treatment in anesthesiology and intensive care medicine].
In Die Anaesthesiologie
BACKGROUND :
OBJECTIVE :
MATERIAL AND METHODS :
RESULTS AND DISCUSSION :
Sadjadi Mahan, Meersch-Dini Melanie
2023-Mar-06
Acute kidney injury, Artificial intelligence, Biomarkers, Respiratory diseases, Sepsis
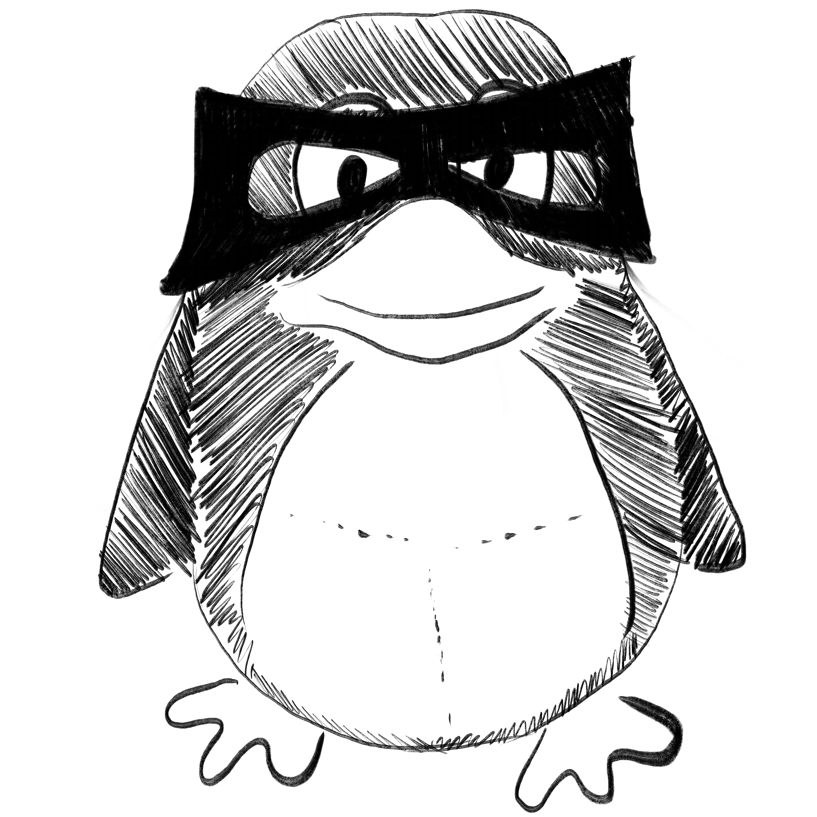
Exploring the use of association rules in random forest for predicting heart disease.
In Computer methods in biomechanics and biomedical engineering
Barry Khalidou Abdoulaye, Manzali Youness, Flouchi Rachid, Balouki Youssef, Chelhi Khadija, Elfar Mohamed
2023-Mar-06
Heart disease, Naïve Bayes, XGBoost, association rules, decision tree, random forest, support vector machine
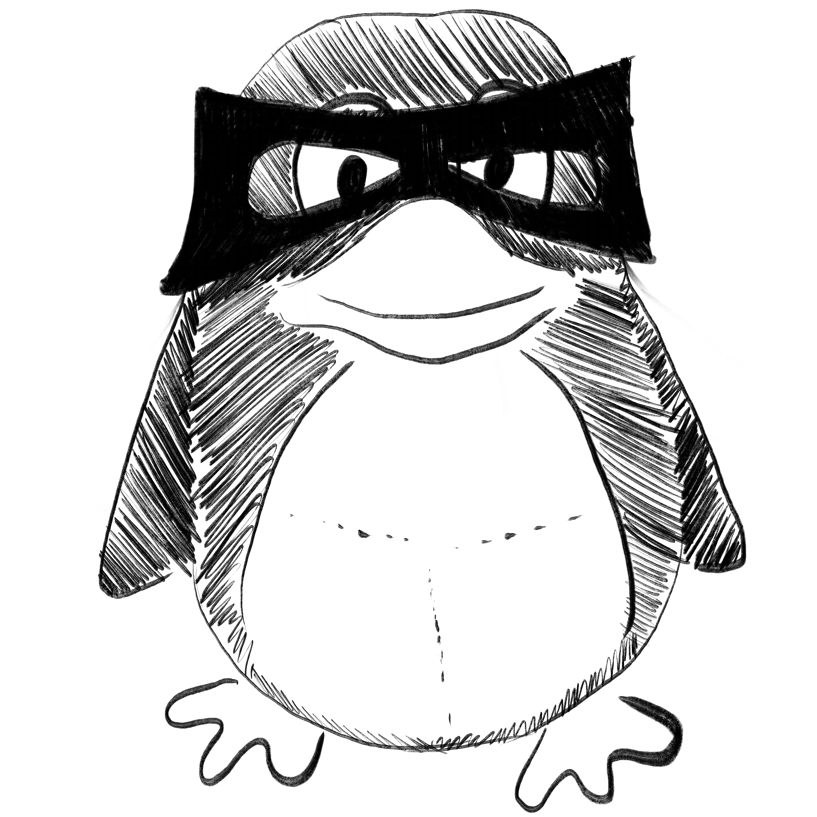
KomaMRI.jl: An open-source framework for general MRI simulations with GPU acceleration.
In Magnetic resonance in medicine ; h5-index 66.0
PURPOSE :
METHODS :
RESULTS :
CONCLUSIONS :
Castillo-Passi Carlos, Coronado Ronal, Varela-Mattatall Gabriel, Alberola-López Carlos, Botnar René, Irarrazaval Pablo
2023-Mar-06
Bloch equations, GPU, GUI, Julia, open source, simulation
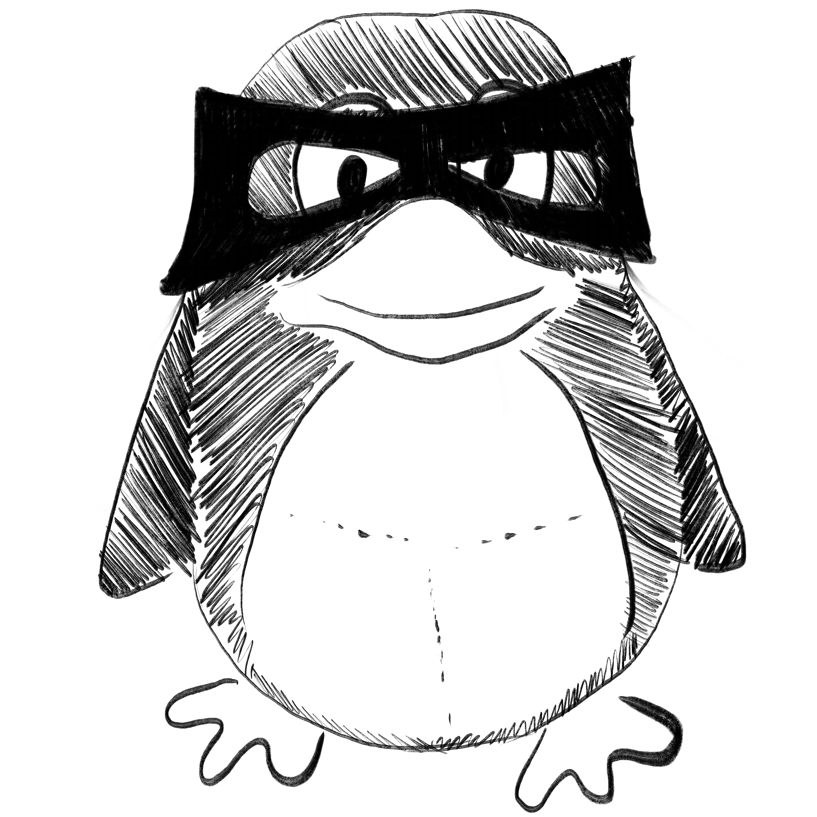
Complex faces and naïve machines A commentary on facial perceptions of age, gender, and leader preferences in the age of AI.
In Politics and the life sciences : the journal of the Association for Politics and the Life Sciences
Spisak Brian R
2023-Mar
age, face perception, facial recognition systems, gender, leadership, voting
Weekly Summary
Receive a weekly summary and discussion of the top papers of the week by leading researchers in the field.