Receive a weekly summary and discussion of the top papers of the week by leading researchers in the field.
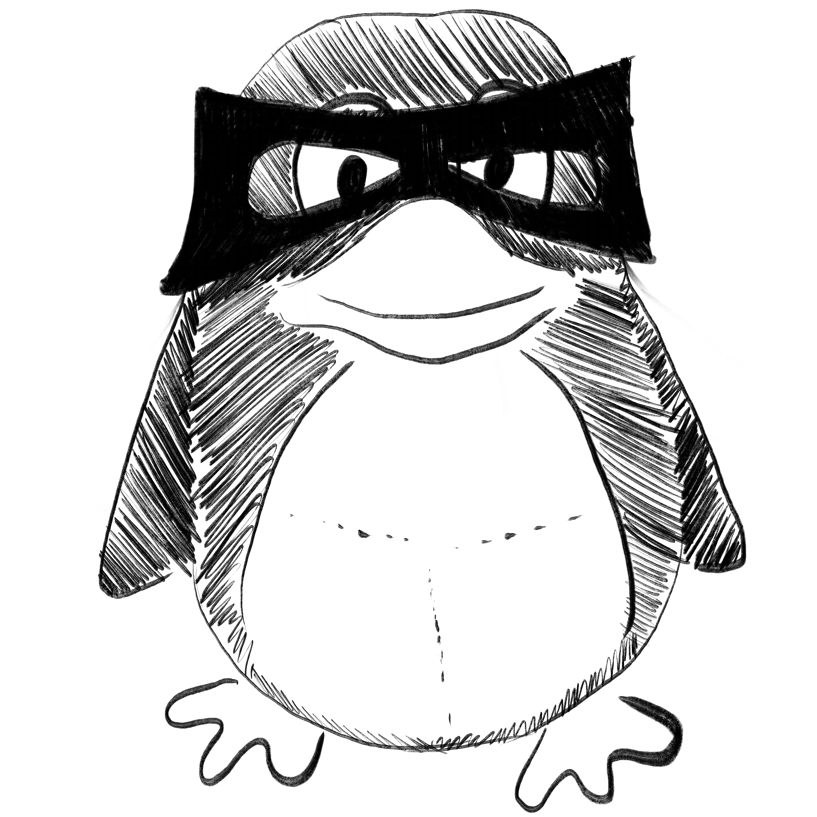
SAN-Net: Learning generalization to unseen sites for stroke lesion segmentation with self-adaptive normalization.
In Computers in biology and medicine
Yu Weiyi, Huang Zhizhong, Zhang Junping, Shan Hongming
2023-Feb-28
Convolutional neural network, Domain generalization, Multi-site learning, Site generalization, Stroke lesion segmentation
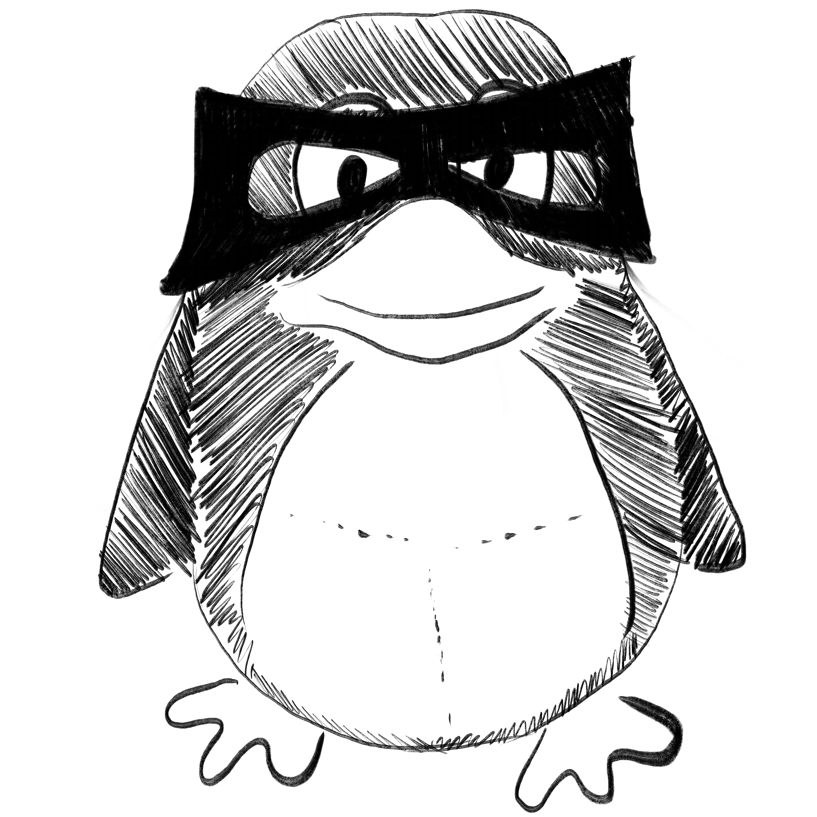
Establishment of extensive artificial intelligence models for kinase inhibitor prediction: Identification of novel PDGFRB inhibitors.
In Computers in biology and medicine
Lien Ssu-Ting, Lin Tony Eight, Hsieh Jui-Hua, Sung Tzu-Ying, Chen Jun-Hong, Hsu Kai-Cheng
2023-Mar-01
Artificial intelligence, Kinase, Machine learning, PDGFRB inhibitor, Small molecule
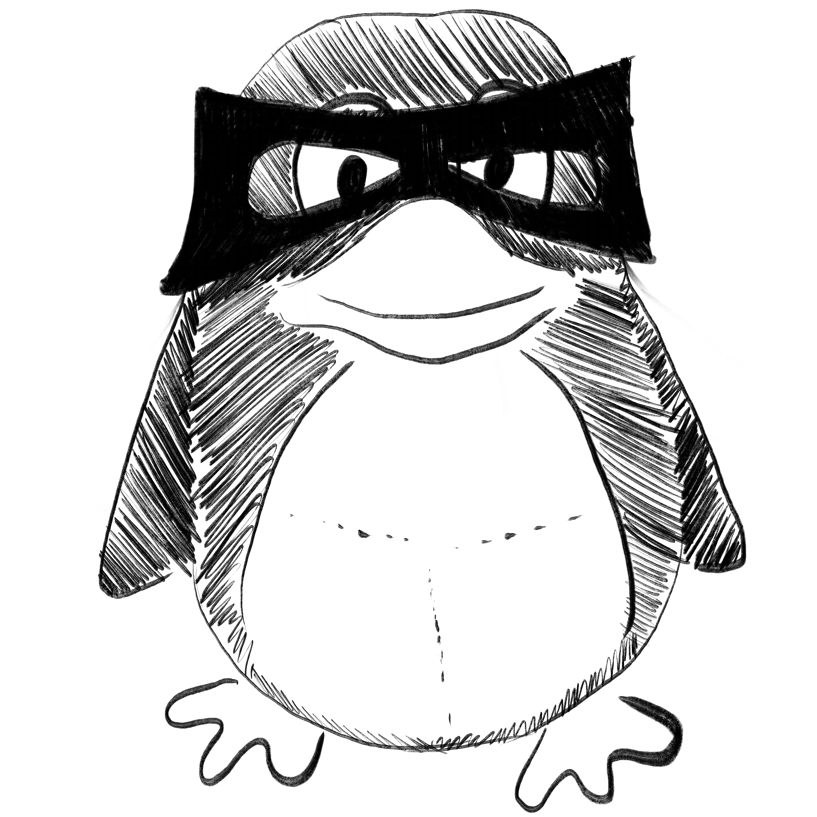
Rough-set based learning: Assessing patterns and predictability of anxiety, depression, and sleep scores associated with the use of cannabinoid-based medicine during COVID-19.
In Frontiers in artificial intelligence
Ramanna Sheela, Ashrafi Negin, Loster Evan, Debroni Karen, Turner Shelley
2023
cannabinoid medicine, electronic health records, machine learning, mental health, rough sets, rough-fuzzy sets
Integrative Scoring System for Survival Prediction in Patients With Locally Advanced Nasopharyngeal Carcinoma: A Retrospective Multicenter Study.
In JCO clinical cancer informatics
PURPOSE :
MATERIALS AND METHODS :
RESULTS :
CONCLUSION :
Zhang Bin, Luo Chun, Zhang Xiao, Hou Jing, Liu Shuyi, Gao Mingyong, Zhang Lu, Jin Zhe, Chen Qiuying, Yu Xiaoping, Zhang Shuixing
2023-Feb
Application of machine learning approach in emergency department to support clinical decision making for SARS-CoV-2 infected patients.
In Journal of integrative bioinformatics
Casano Nicolò, Santini Silvano Junior, Vittorini Pierpaolo, Sinatti Gaia, Carducci Paolo, Mastroianni Claudio Maria, Ciardi Maria Rosa, Pasculli Patrizia, Petrucci Emiliano, Marinangeli Franco, Balsano Clara
2023-Mar-07
COVID-19, ROX index, SARS-CoV-2, emergency medicine, machine learning
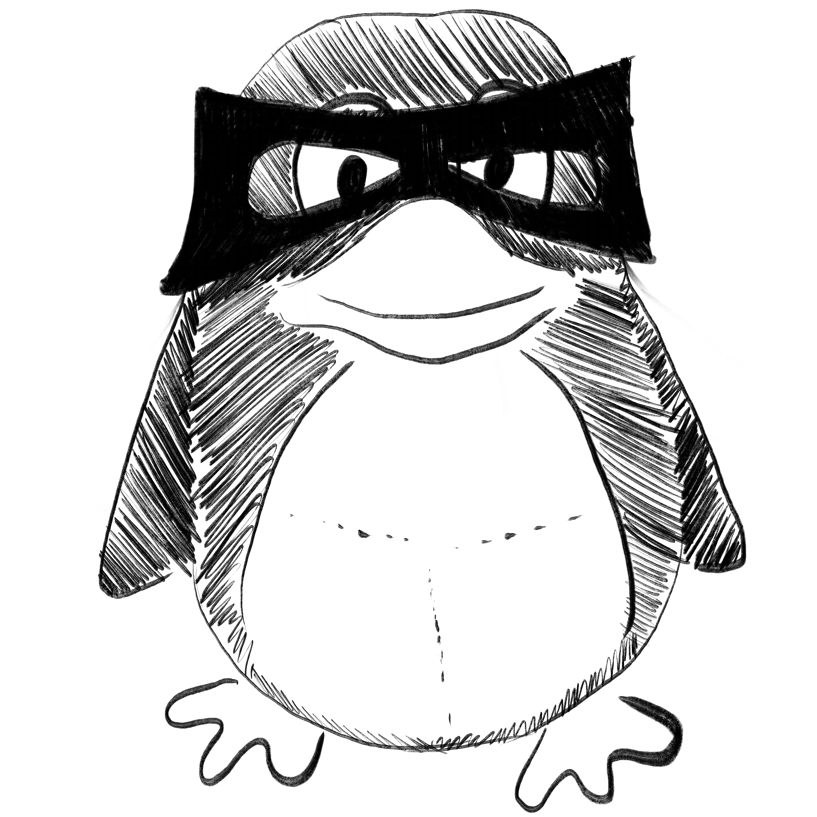
Social copying drives a tipping point for nonlinear population collapse.
In Proceedings of the National Academy of Sciences of the United States of America
Oro Daniel, Alsedà Lluís, Hastings Alan, Genovart Meritxell, Sardanyés Josep
2023-Mar-14
feedback, nonlinear population dynamics, runaway dispersal, social behavior, tipping points
Weekly Summary
Receive a weekly summary and discussion of the top papers of the week by leading researchers in the field.