Receive a weekly summary and discussion of the top papers of the week by leading researchers in the field.
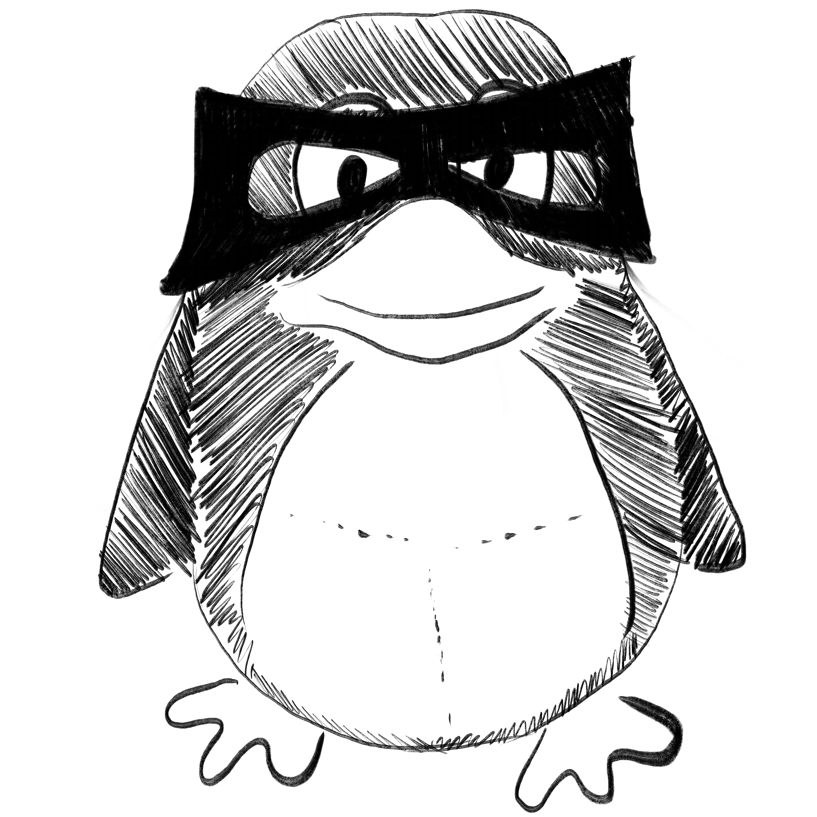
Dielectric Properties of Polymer Nanocomposite Interphases Using Electrostatic Force Microscopy and Machine Learning.
In ACS applied electronic materials
Gupta Praveen, Ruzicka Eric, Benicewicz Brian C, Sundararaman Ravishankar, Schadler Linda S
2023-Feb-28
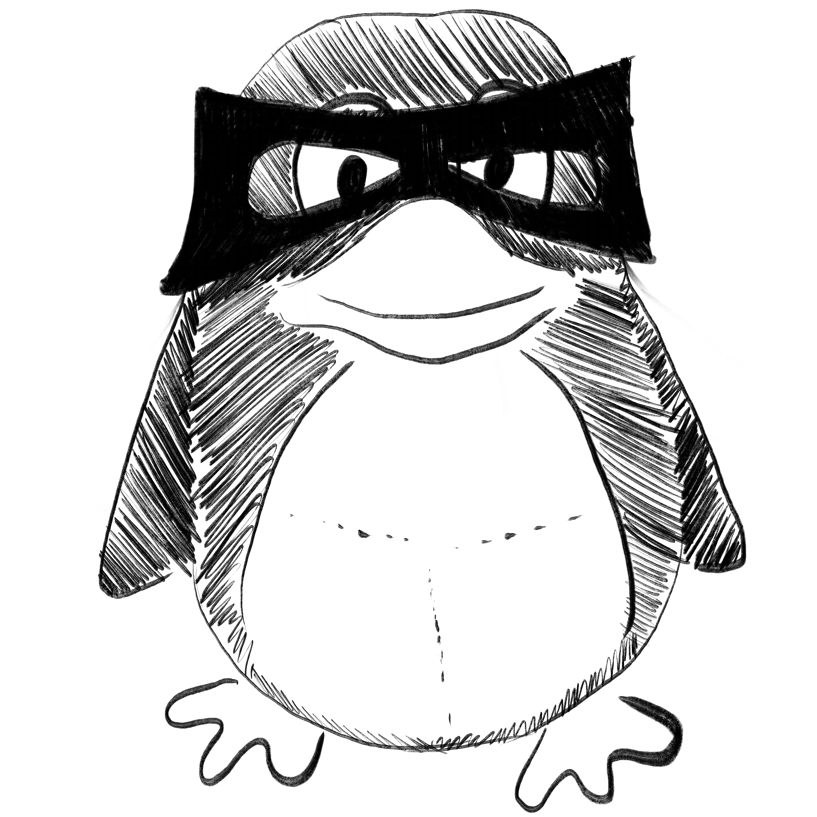
Normative model detects abnormal functional connectivity in psychiatric disorders.
In Frontiers in psychiatry
INTRODUCTION :
METHODS :
RESULTS AND DISCUSSION :
Oliveira-Saraiva Duarte, Ferreira Hugo Alexandre
2023
deep learning, functional brain network, functional connectivity, normative model, psychiatric disorders
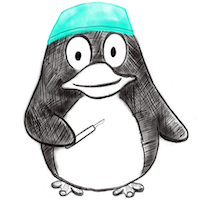
Endometriosis leads to central nervous system-wide glial activation in a mouse model of endometriosis.
In Journal of neuroinflammation
BACKGROUND :
METHODS :
RESULTS :
CONCLUSION :
Bashir Shah Tauseef, Redden Catherine R, Raj Kishori, Arcanjo Rachel B, Stasiak Sandra, Li Quanxi, Steelman Andrew J, Nowak Romana A
2023-Mar-06
Chronic pelvic pain (CPP), Endometriosis, Glial activation, Hyperalgesia
Science fiction or clinical reality: a review of the applications of artificial intelligence along the continuum of trauma care.
In World journal of emergency surgery : WJES
Hunter Olivia F, Perry Frances, Salehi Mina, Bandurski Hubert, Hubbard Alan, Ball Chad G, Morad Hameed S
2023-Mar-06
Artificial intelligence, Machine learning, Trauma
Differentiation of acute and chronic vertebral compression fractures using conventional CT based on deep transfer learning features and hand-crafted radiomics features.
In BMC musculoskeletal disorders ; h5-index 46.0
BACKGROUND :
METHODS :
RESULTS :
CONCLUSION :
Zhang Jun, Liu Jiayi, Liang Zhipeng, Xia Liang, Zhang Weixiao, Xing Yanfen, Zhang Xueli, Tang Guangyu
2023-Mar-06
Deep learning, Differential diagnosis, Radiomics, Tomography, X-ray computed, Vertebral compression fracture (VCF)
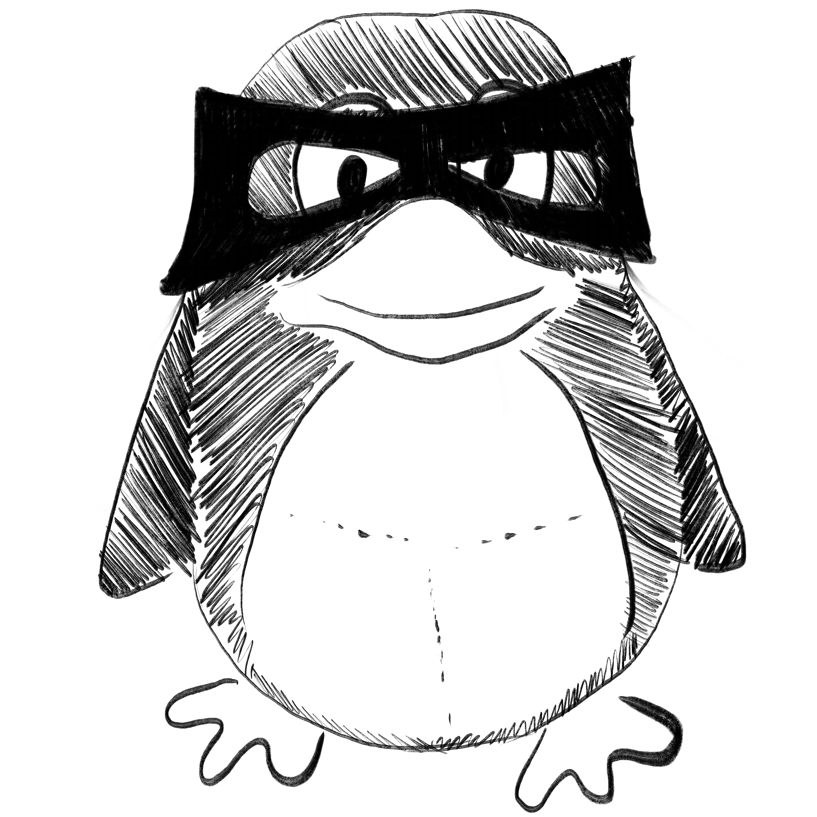
Decision tree learning in Neo4j on homogeneous and unconnected graph nodes from biological and clinical datasets.
In BMC medical informatics and decision making ; h5-index 38.0
BACKGROUND :
METHODS :
RESULTS :
CONCLUSION :
Mondal Rahul, Do Minh Dung, Ahmed Nasim Uddin, Walke Daniel, Micheel Daniel, Broneske David, Saake Gunter, Heyer Robert
2023-Mar-06
Cypher, Decision tree, Graph database, Java, Neo4j, Python, R
Weekly Summary
Receive a weekly summary and discussion of the top papers of the week by leading researchers in the field.