Receive a weekly summary and discussion of the top papers of the week by leading researchers in the field.
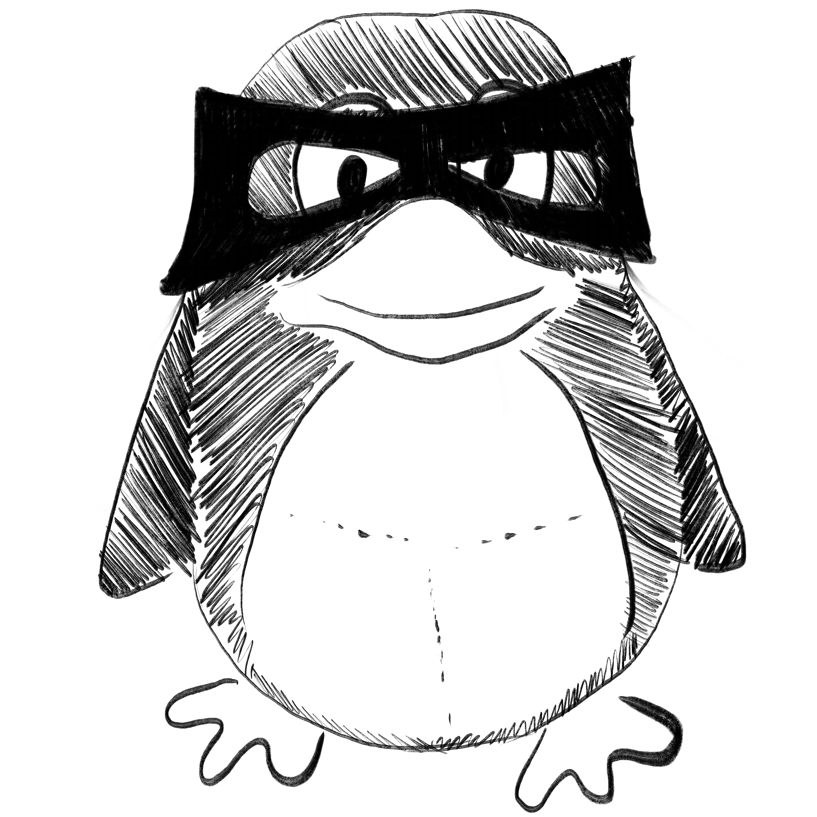
Decreased Resting-State Alpha Self-Synchronization in Depressive Disorder.
In Clinical EEG and neuroscience
Mohammadi Yousef, Kafraj Mohadeseh Shafiei, Graversen Carina, Moradi Mohammad Hassan
2023-Mar-21
Beck Depression Inventory-II, EEG, alpha self-synchronization, depression severity, neural oscillations
Attention fusion network with self-supervised learning for staging of osteonecrosis of the femoral head (ONFH) using multiple MR protocols.
In Medical physics ; h5-index 59.0
BACKGROUND :
PURPOSE :
METHODS :
RESULTS :
CONCLUSIONS :
Kim Bomin, Lee Geun Young, Park Sung-Hong
2023-Mar-21
attention fusion, mr-only staging, multiple mr protocols, osteonecrosis of femoral head, self-supervised learning
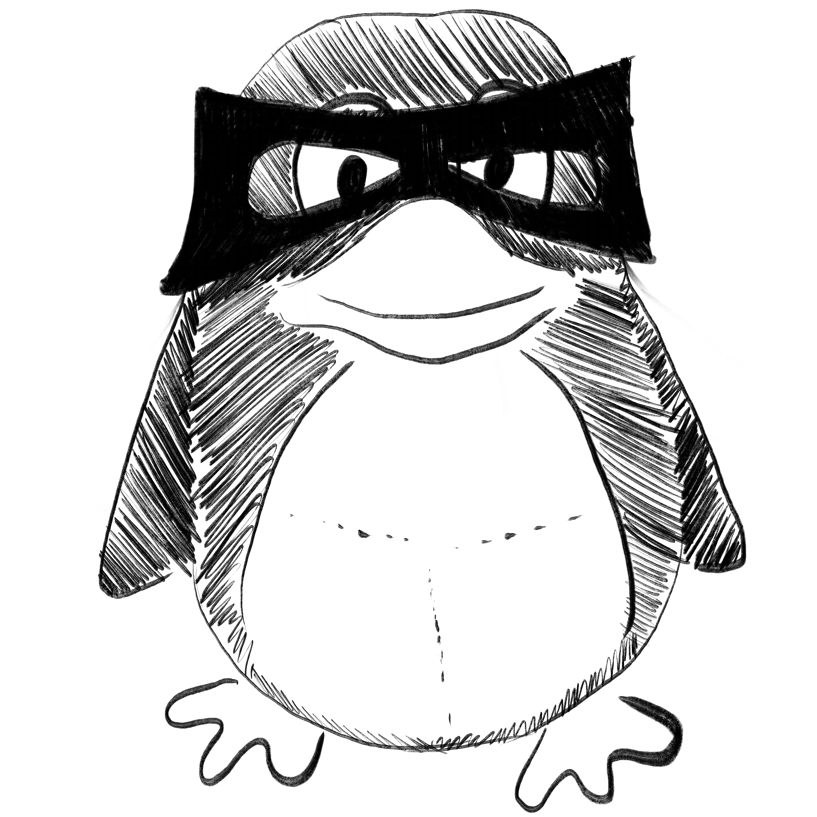
Need for Objective Task-based Evaluation of Deep Learning-Based Denoising Methods: A Study in the Context of Myocardial Perfusion SPECT.
In ArXiv
Yu Zitong, Rahman Md Ashequr, Laforest Richard, Schindler Thomas H, Gropler Robert J, Wahl Richard L, Siegel Barry A, Jha Abhinav K
2023-Mar-16
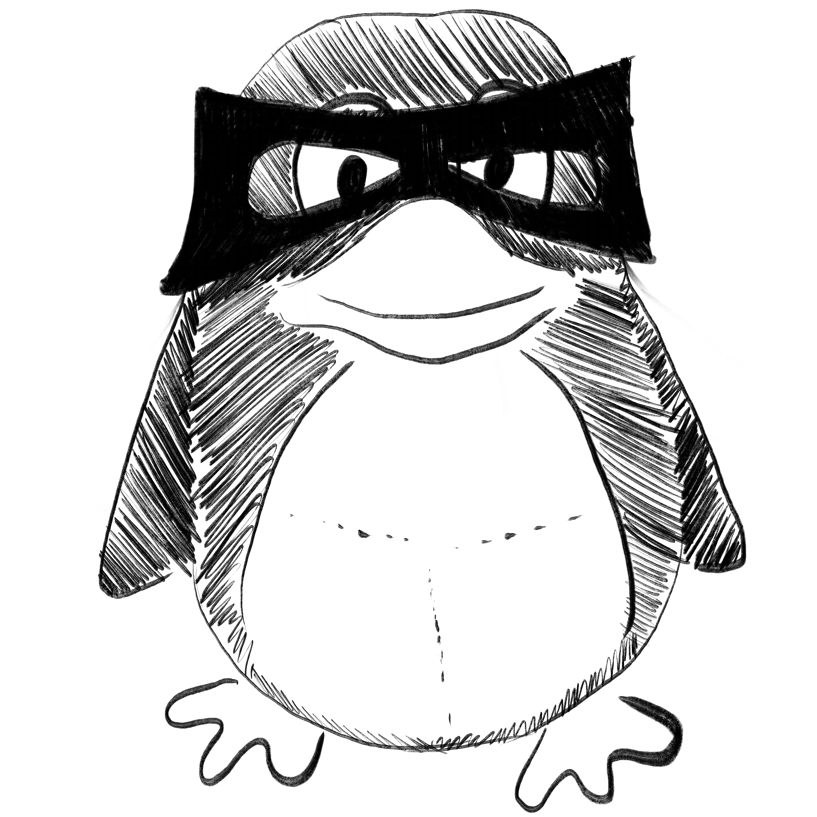
Understanding metric-related pitfalls in image analysis validation.
In ArXiv
Reinke Annika, Tizabi Minu D, Baumgartner Michael, Eisenmann Matthias, Heckmann-Nötzel Doreen, Kavur A Emre, Rädsch Tim, Sudre Carole H, Acion Laura, Antonelli Michela, Arbel Tal, Bakas Spyridon, Benis Arriel, Blaschko Matthew, Büttner Florian, Cardoso M Jorge, Cheplygina Veronika, Chen Jianxu, Christodoulou Evangelia, Cimini Beth A, Collins Gary S, Farahani Keyvan, Ferrer Luciana, Galdran Adrian, van Ginneken Bram, Glocker Ben, Godau Patrick, Haase Robert, Hashimoto Daniel A, Hoffman Michael M, Huisman Merel, Isensee Fabian, Jannin Pierre, Kahn Charles E, Kainmueller Dagmar, Kainz Bernhard, Karargyris Alexandros, Karthikesalingam Alan, Kenngott Hannes, Kleesiek Jens, Kofler Florian, Kooi Thijs, Kopp-Schneider Annette, Kozubek Michal, Kreshuk Anna, Kurc Tahsin, Landman Bennett A, Litjens Geert, Madani Amin, Maier-Hein Klaus, Martel Anne L, Mattson Peter, Meijering Erik, Menze Bjoern, Moons Karel G M, Müller Henning, Nichyporuk Brennan, Nickel Felix, Petersen Jens, Rafelski Susanne M, Rajpoot Nasir, Reyes Mauricio, Riegler Michael A, Rieke Nicola, Saez-Rodriguez Julio, Sánchez Clara I, Shetty Shravya, van Smeden Maarten, Summers Ronald M, Taha Abdel A, Tiulpin Aleksei, Tsaftaris Sotirios A, Calster Ben Van, Varoquaux Gaël, Wiesenfarth Manuel, Yaniv Ziv R, Jäger Paul F, Maier-Hein Lena
2023-Feb-09
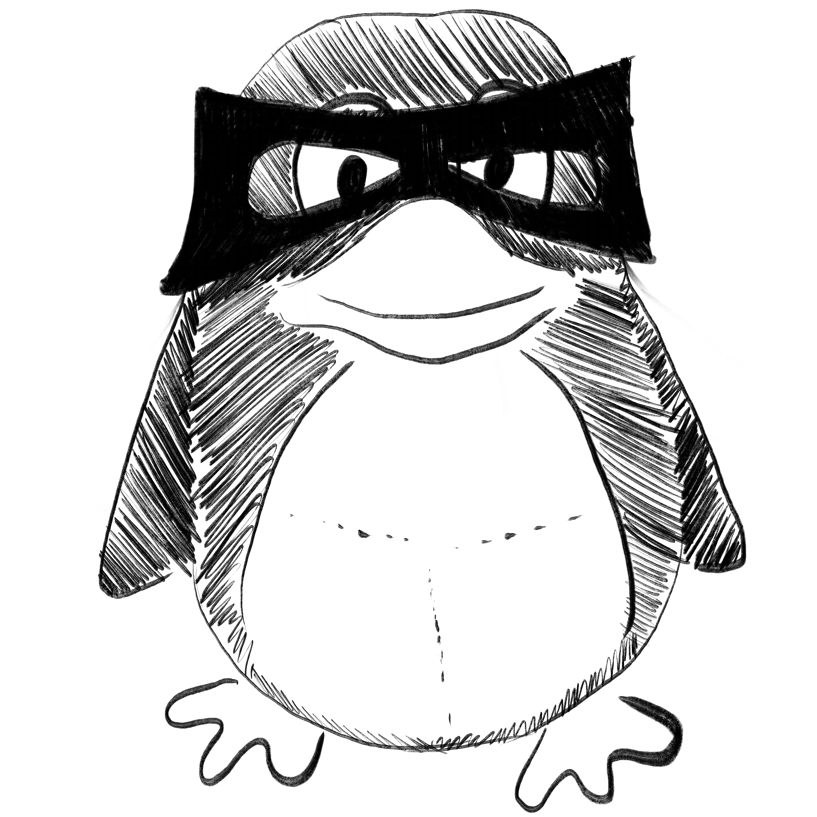
Roadmap on Deep Learning for Microscopy.
In ArXiv
Volpe Giovanni, Wählby Carolina, Tian Lei, Hecht Michael, Yakimovich Artur, Monakhova Kristina, Waller Laura, Sbalzarini Ivo F, Metzler Christopher A, Xie Mingyang, Zhang Kevin, Lenton Isaac C D, Rubinsztein-Dunlop Halina, Brunner Daniel, Bai Bijie, Ozcan Aydogan, Midtvedt Daniel, Wang Hao, Sladoje Nataša, Lindblad Joakim, Smith Jason T, Ochoa Marien, Barroso Margarida, Intes Xavier, Qiu Tong, Yu Li-Yu, You Sixian, Liu Yongtao, Ziatdinov Maxim A, Kalinin Sergei V, Sheridan Arlo, Manor Uri, Nehme Elias, Goldenberg Ofri, Shechtman Yoav, Moberg Henrik K, Langhammer Christoph, Špačková Barbora, Helgadottir Saga, Midtvedt Benjamin, Argun Aykut, Thalheim Tobias, Cichos Frank, Bo Stefano, Hubatsch Lars, Pineda Jesus, Manzo Carlo, Bachimanchi Harshith, Selander Erik, Homs-Corbera Antoni, Fränzl Martin, de Haan Kevin, Rivenson Yair, Korczak Zofia, Adiels Caroline Beck, Mijalkov Mite, Veréb Dániel, Chang Yu-Wei, Pereira Joana B, Matuszewski Damian, Kylberg Gustaf, Sintorn Ida-Maria, Caicedo Juan C, Cimini Beth A, Bell Muyinatu A Lediju, Saraiva Bruno M, Jacquemet Guillaume, Henriques Ricardo, Ouyang Wei, Le Trang, Gómez-de-Mariscal Estibaliz, Sage Daniel, Muñoz-Barrutia Arrate, Lindqvist Ebba Josefson, Bergman Johanna
2023-Mar-07
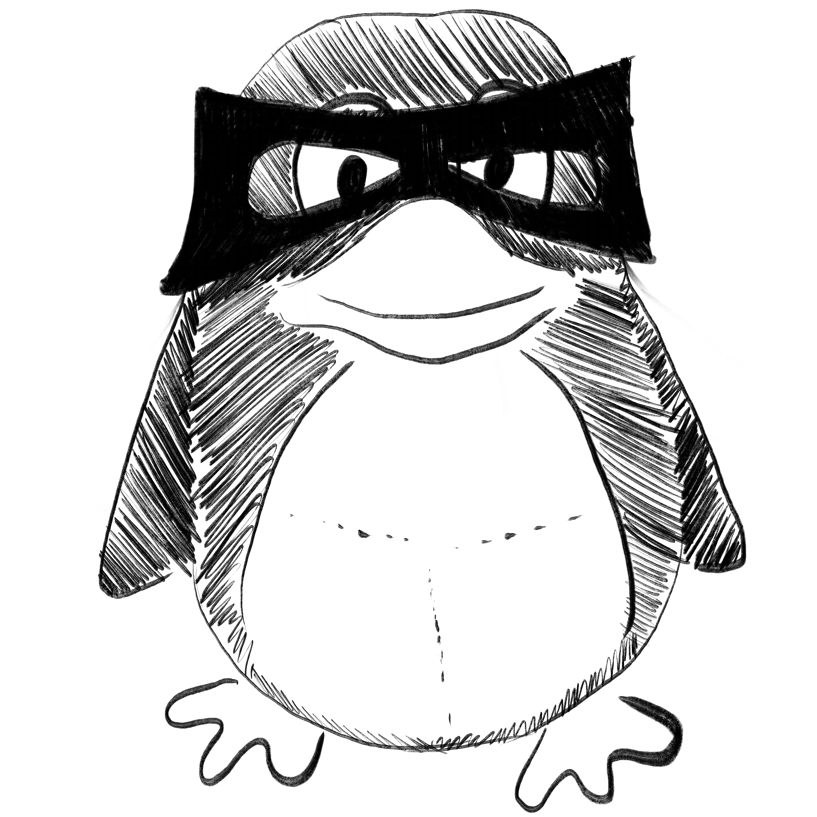
Risk Factors and Predictive Modeling for Post-Acute Sequelae of SARS-CoV-2 Infection: Findings from EHR Cohorts of the RECOVER Initiative.
In Research square
Zang Chengxi, Hou Yu, Schenck Edward, Xu Zhenxing, Zhang Yongkang, Xu Jie, Bian Jiang, Morozyuk Dmitry, Khullar Dhruv, Nordvig Anna, Shenkman Elizabeth, Rothman Russel, Block Jason, Lyman Kristin, Zhang Yiye, Varma Jay, Weiner Mark, Carton Thomas, Wang Fei, Kaushal Rainu, Consortium The Recover
2023-Mar-08
Weekly Summary
Receive a weekly summary and discussion of the top papers of the week by leading researchers in the field.