Receive a weekly summary and discussion of the top papers of the week by leading researchers in the field.
Development and validation of a nomogram to predict mortality risk in patients with ischemic heart disease.
In Frontiers in cardiovascular medicine
BACKGROUND :
METHODS :
RESULTS :
CONCLUSION :
Yang Long, Dong Xia, Abuduaini Baiheremujiang, Jiamali Nueraihemaiti, Seyiti Zulihuma, Shan Xue-Feng, Gao Xiao-Ming
2023
LASSO, ischemic heart disease, machine learning, mortality, nomogram
Artificial intelligence in coronary computed tomography angiography: Demands and solutions from a clinical perspective.
In Frontiers in cardiovascular medicine
Baeßler Bettina, Götz Michael, Antoniades Charalambos, Heidenreich Julius F, Leiner Tim, Beer Meinrad
2023
artificial intelligence, cardiac computed tomography, clinical workflow, coronary computed tomography angiography, deep learning, machine learning, radiomics
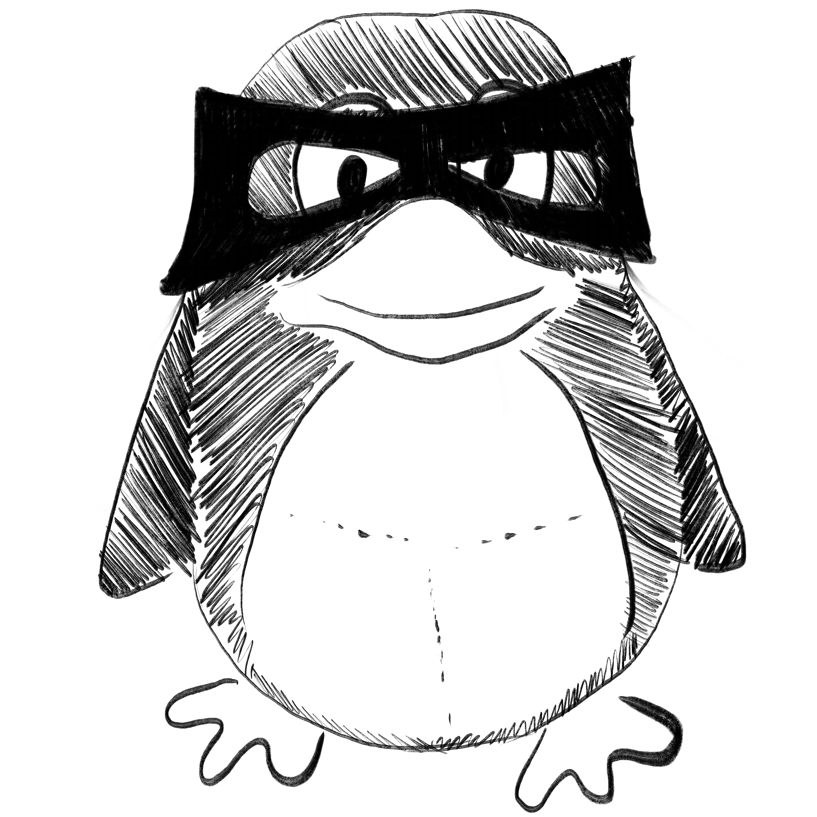
Design and Proofreading of the English-Chinese Computer-Aided Translation System by the Neural Network.
In Computational intelligence and neuroscience
Liu Yutong, Zhang Shile
2023
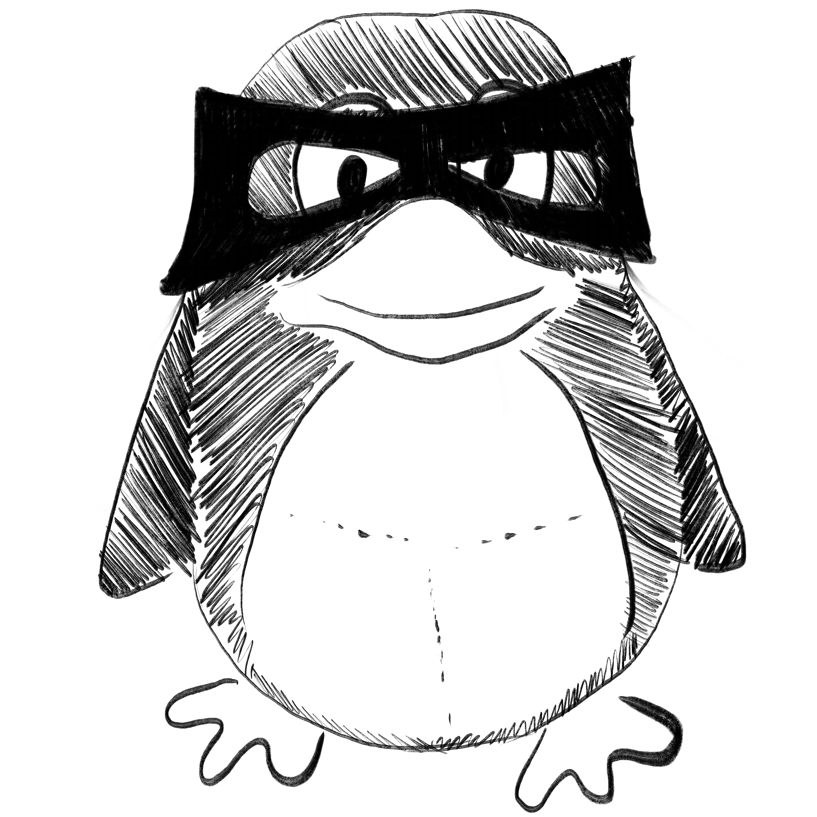
A Greedy Optimized Intelligent Framework for Early Detection of Alzheimer's Disease Using EEG Signal.
In Computational intelligence and neuroscience
Swarnalatha R
2023
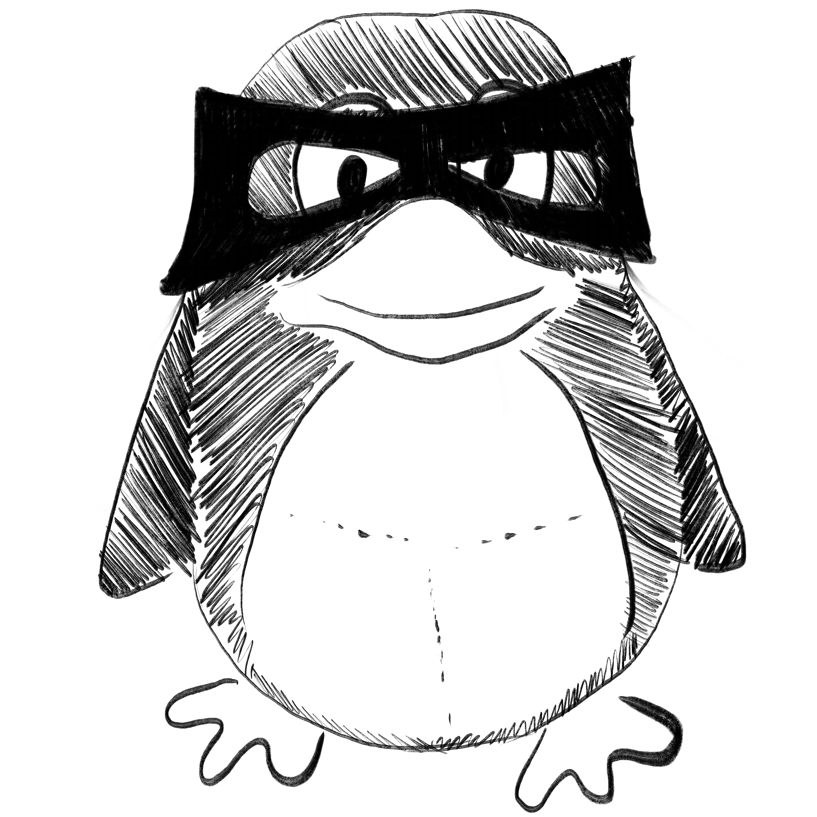
Computational Thinking Training and Deep Learning Evaluation Model Construction Based on Scratch Modular Programming Course.
In Computational intelligence and neuroscience
Chen Xiaoli, Wang XiaoMing
2023
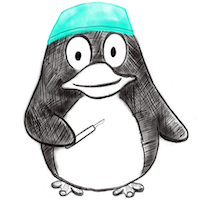
Effects of age, body height, body weight, body mass index and handgrip strength on the trajectory of the plantar pressure stance-phase curve of the gait cycle.
In Frontiers in bioengineering and biotechnology
Wolff Christian, Steinheimer Patrick, Warmerdam Elke, Dahmen Tim, Slusallek Philipp, Schlinkmann Christian, Chen Fei, Orth Marcel, Pohlemann Tim, Ganse Bergita
2023
ageing, gait, ground reaction (forces), handgrip strengh, insoles, motion analysis, obesity, smart healthcare
Weekly Summary
Receive a weekly summary and discussion of the top papers of the week by leading researchers in the field.