Receive a weekly summary and discussion of the top papers of the week by leading researchers in the field.
A Machine Learning Prediction Model for Immediate Graft Function After Deceased Donor Kidney Transplantation.
In Transplantation ; h5-index 56.0
BACKGROUND :
METHODS :
RESULTS :
CONCLUSIONS :
Quinino Raquel M, Agena Fabiana, Modelli de Andrade Luis Gustavo, Furtado Mariane, Chiavegatto Filho Alexandre D P, David-Neto Elias
2023-Mar-06
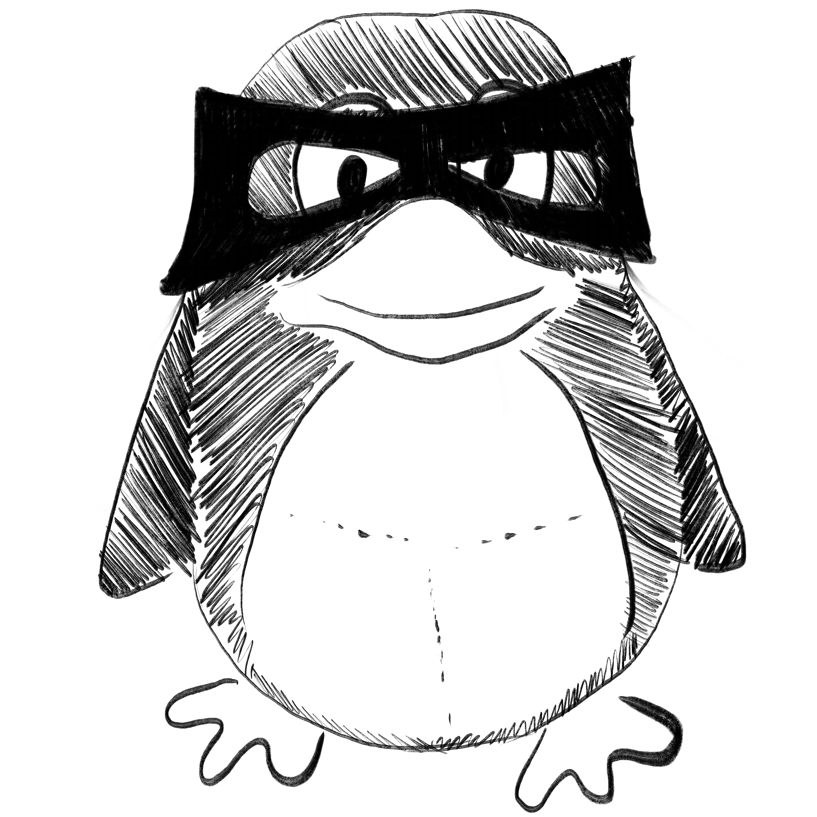
Coronavirus diagnosis using cough sounds: Artificial intelligence approaches.
In Frontiers in artificial intelligence
INTRODUCTION :
METHOD :
RESULT :
CONCLUSION :
Askari Nasab Kazem, Mirzaei Jamal, Zali Alireza, Gholizadeh Sarfenaz, Akhlaghdoust Meisam
2023
artificial intelligence, coronavirus, cough, deep learning, machine learning, respiratory sounds
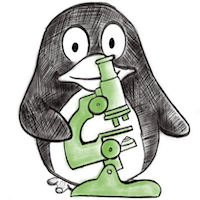
Patched Diffusion Models for Unsupervised Anomaly Detection in Brain MRI
ArXiv Preprint
Finn Behrendt, Debayan Bhattacharya, Julia Krüger, Roland Opfer, Alexander Schlaefer
2023-03-07
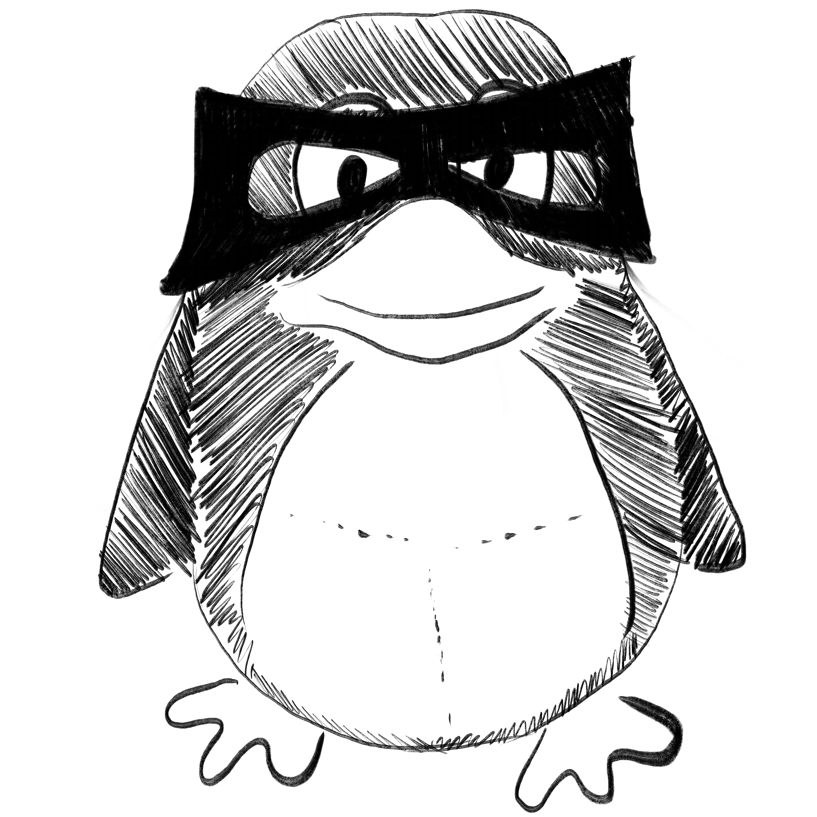
Application of deep learning in recognition of accrued earnings management.
In Heliyon
Li Jia, Sun Zhoutianyang
2023-Mar
Artificial intelligence, Deep belief network, Deep learning, Earnings management, Jones model
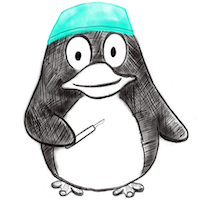
Benchmarking performance of an automatic polysomnography scoring system in a population with suspected sleep disorders.
In Frontiers in neurology
AIM :
METHODOLOGY :
RESULTS :
CONCLUSION :
Choo Bryan Peide, Mok Yingjuan, Oh Hong Choon, Patanaik Amiya, Kishan Kishan, Awasthi Animesh, Biju Siddharth, Bhattacharjee Soumya, Poh Yvonne, Wong Hang Siang
2023
AI sleep scoring, automatic sleep scoring, machine learning, sleep staging, sleep-disordered breathing
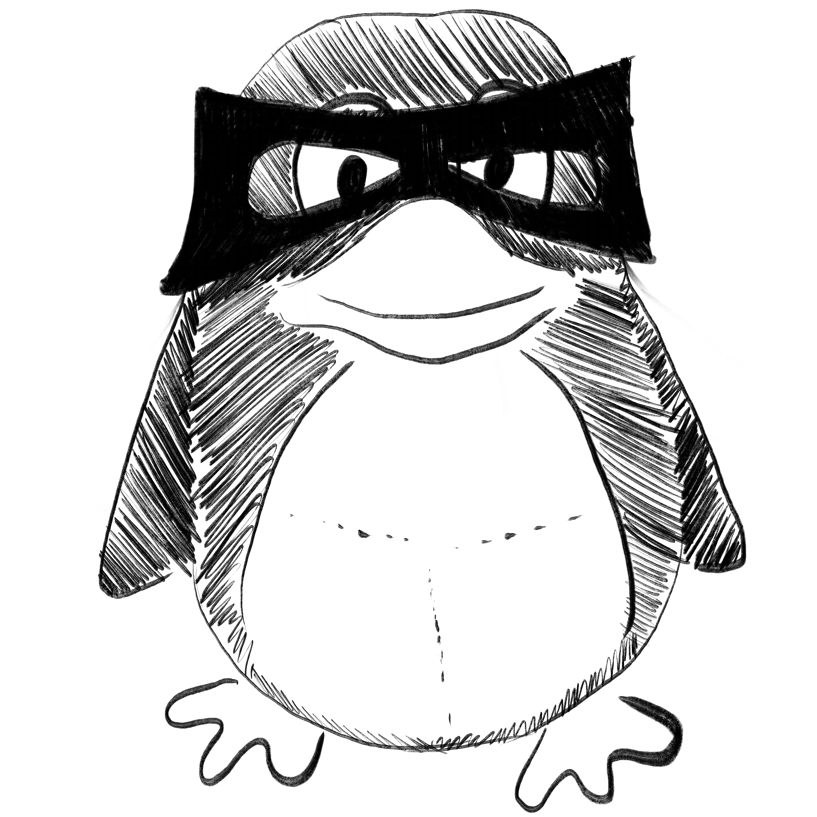
mRisk: Continuous Risk Estimation for Smoking Lapse from Noisy Sensor Data with Incomplete and Positive-Only Labels.
In Proceedings of the ACM on interactive, mobile, wearable and ubiquitous technologies
Ullah Md Azim, Chatterjee Soujanya, Fagundes Christopher P, Lam Cho, Nahum-Shani Inbal, Rehg James M, Wetter David W, Kumar Santosh
2022-Sep
Behavioral Intervention, Human-centered computing, Risk prediction, Smoking Cessation, Ubiquitous and mobile computing design and evaluation methods, Wearable Sensors, mHealth
Weekly Summary
Receive a weekly summary and discussion of the top papers of the week by leading researchers in the field.