Receive a weekly summary and discussion of the top papers of the week by leading researchers in the field.
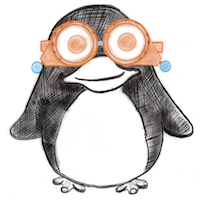
Role of artificial intelligence in determining factors impacting patients' refractive surgery decisions.
In Indian journal of ophthalmology
PURPOSE :
METHODS :
RESULTS :
CONCLUSION :
Kundu Gairik, Virani Imranali, Shetty Rohit, Khamar Pooja, Nuijts Rudy M M A
2023-Mar
Artificial intelligence, machine learning, ophthalmologic surgical procedures, predictive analysis
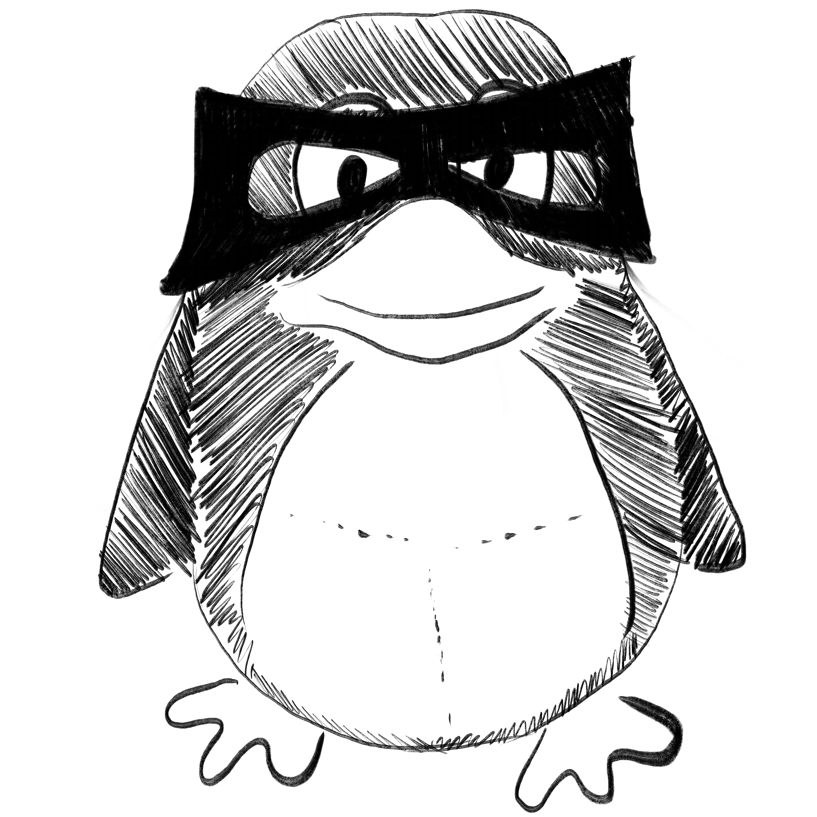
Rough-set based learning: Assessing patterns and predictability of anxiety, depression, and sleep scores associated with the use of cannabinoid-based medicine during COVID-19.
In Frontiers in artificial intelligence
Ramanna Sheela, Ashrafi Negin, Loster Evan, Debroni Karen, Turner Shelley
2023
cannabinoid medicine, electronic health records, machine learning, mental health, rough sets, rough-fuzzy sets
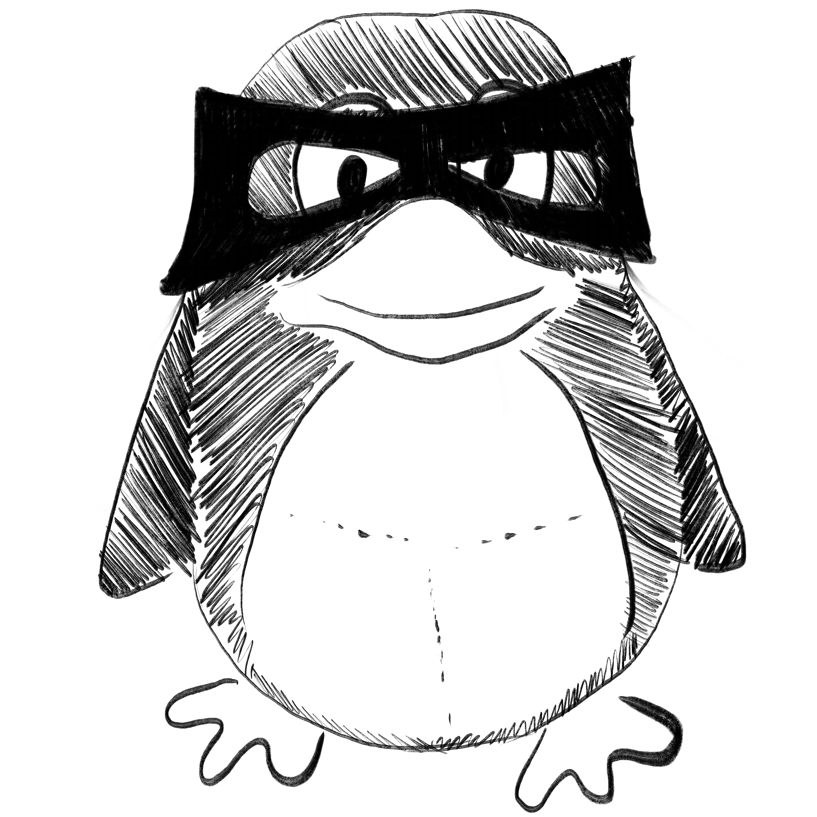
Recent deep learning models for dementia as point-of-care testing: Potential for early detection.
In Intractable & rare diseases research
Karako Kenji, Song Peipei, Chen Yu
2023-Feb
deep learning, dementia, point-of-cate testing, prediction
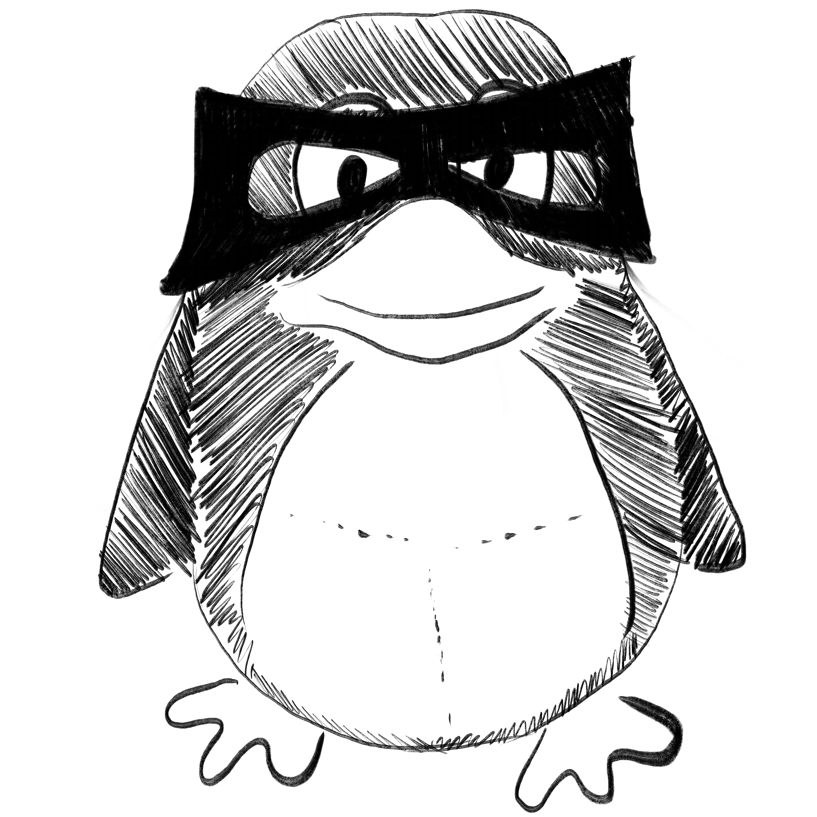
Polymer Informatics at Scale with Multitask Graph Neural Networks.
In Chemistry of materials : a publication of the American Chemical Society
Gurnani Rishi, Kuenneth Christopher, Toland Aubrey, Ramprasad Rampi
2023-Feb-28
A multi-use deep learning method for CITE-seq and single-cell RNA-seq data integration with cell surface protein prediction and imputation.
In Nature machine intelligence
Lakkis Justin, Schroeder Amelia, Su Kenong, Lee Michelle Y Y, Bashore Alexander C, Reilly Muredach P, Li Mingyao
2022-Nov
CITE-seq, deep learning, protein prediction, single-cell RNA-seq, single-cell multi-omics
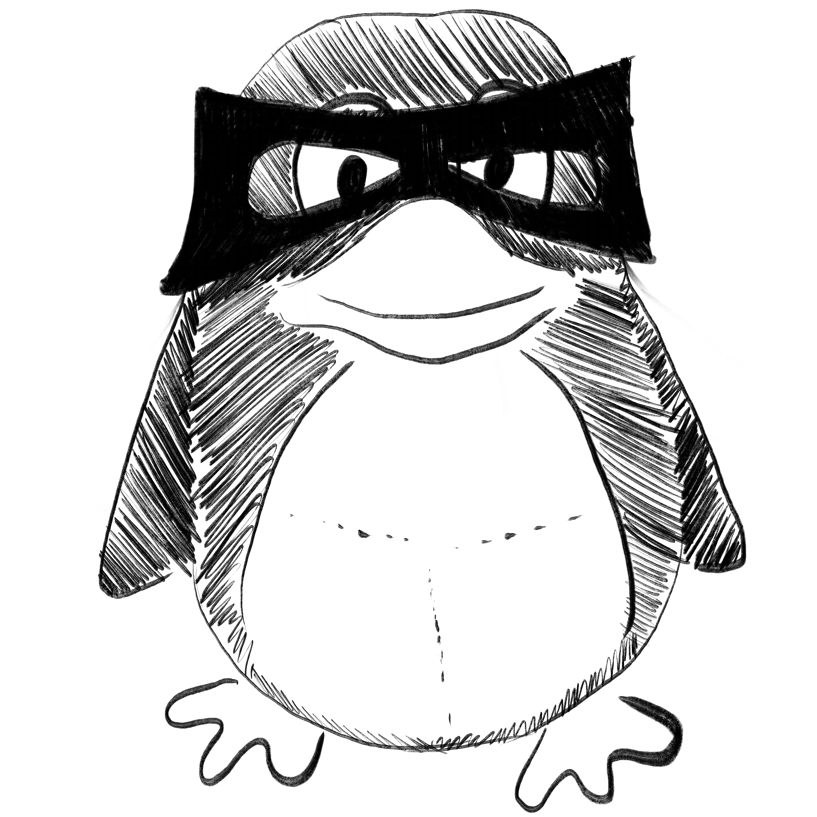
Identifying resilience strategies for disruption management in the healthcare supply chain during COVID-19 by digital innovations: A systematic literature review.
In Informatics in medicine unlocked
Arji Goli, Ahmadi Hossein, Avazpoor Pejman, Hemmat Morteza
2023
COVID-19, Healthcare supply chain, Literature review, Pandemics, Supply chain management
Weekly Summary
Receive a weekly summary and discussion of the top papers of the week by leading researchers in the field.