Receive a weekly summary and discussion of the top papers of the week by leading researchers in the field.
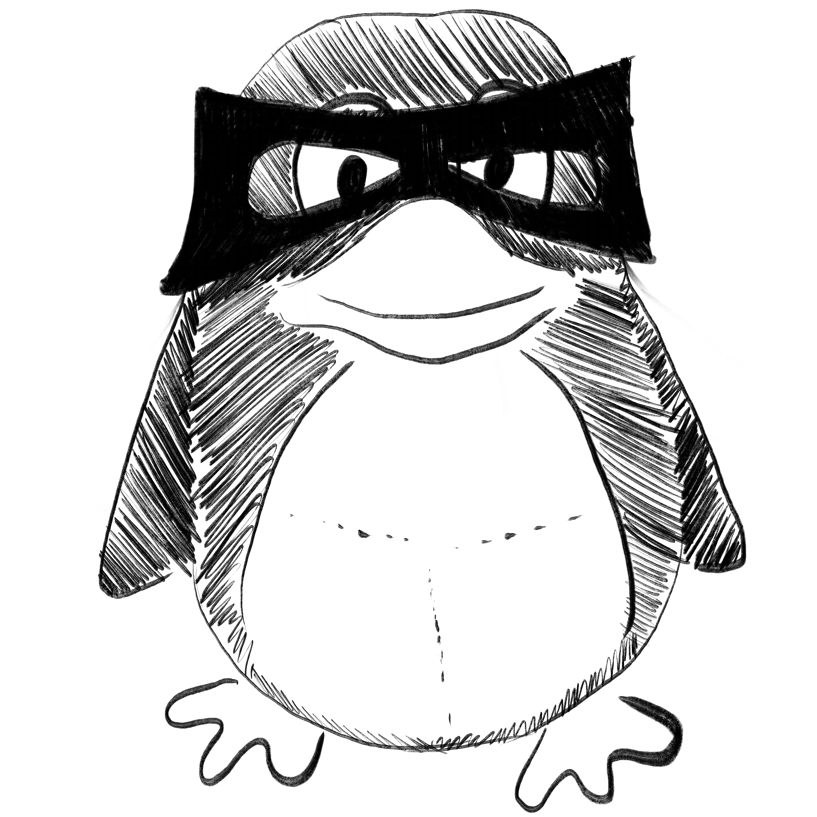
Increased Effective Connectivity of the Left Parietal Lobe During Walking Tasks in Parkinson's Disease.
In Journal of Parkinson's disease
BACKGROUND :
OBJECTIVE :
METHODS :
RESULTS :
CONCLUSION :
Wang Yue, Yu Ningbo, Lu Jiewei, Zhang Xinyuan, Wang Jin, Shu Zhilin, Cheng Yuanyuan, Zhu Zhizhong, Yu Yang, Liu Peipei, Han Jianda, Wu Jialing
2023-Mar-03
Effective connectivity, Parkinson’s disease, cerebral cortex, functional near-infrared spectroscopy, gait automaticity
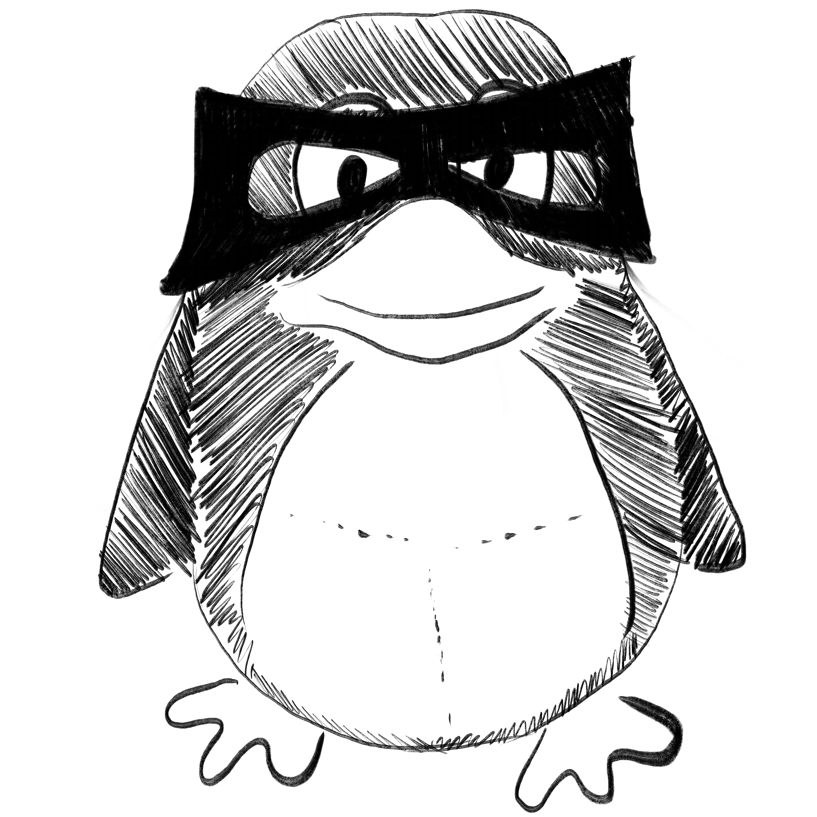
Present and future perspectives in early diagnosis and monitoring for progressive fibrosing interstitial lung diseases.
In Frontiers in medicine
Stanel Stefan Cristian, Rivera-Ortega Pilar
2023
PF-ILD, PPF, idiopathic pulmonary fibrosis, interstitial lung disease, progressive fibrosing interstitial lung disease, progressive pulmonary fibrosis
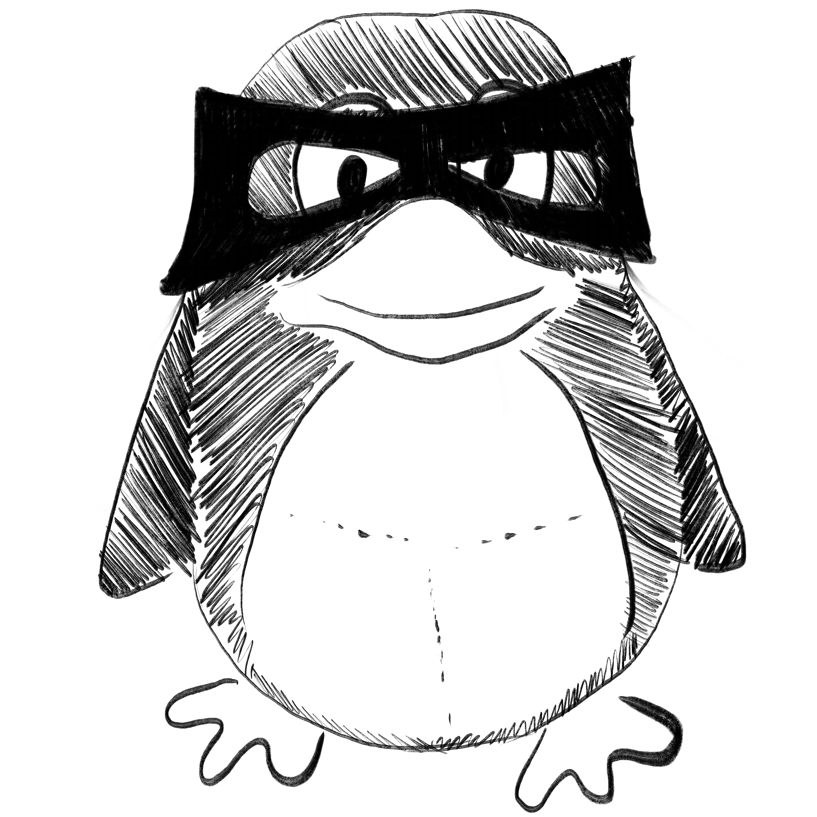
Clinical Trial Protocol: Developing an Image Classification Algorithm for Prostate Cancer Diagnosis on Three-dimensional Multiparametric Transrectal Ultrasound.
In European urology open science
INTRODUCTION AND HYPOTHESIS :
DESIGN :
PROTOCOL OVERVIEW :
STATISTICAL ANALYSIS :
DISCUSSION AND SUMMARY :
Jager Auke, Postema Arnoud W, Mischi Massimo, Wijkstra Hessel, Beerlage Harrie P, Oddens Jorg R
2023-Mar
Contrast-enhanced ultrasound, Elastography, Machine learning, Multiparametric ultrasound, Prostate cancer
Predicting continuous amyloid PET values with CSF and plasma Aβ42/Aβ40.
In Alzheimer's & dementia (Amsterdam, Netherlands)
INTRODUCTION :
METHODS :
RESULTS :
DISCUSSION :
HIGHLIGHTS :
Wisch Julie K, Gordon Brian A, Boerwinkle Anna H, Luckett Patrick H, Bollinger James G, Ovod Vitaliy, Li Yan, Henson Rachel L, West Tim, Meyer Mathew R, Kirmess Kristopher M, Benzinger Tammie L S, Fagan Anne M, Morris John C, Bateman Randall J, Ances Beau M, Schindler Suzanne E
2023
CSF Aβ42/Aβ40, PET, biomarker concordance, machine learning, plasma Aβ42/Aβ40
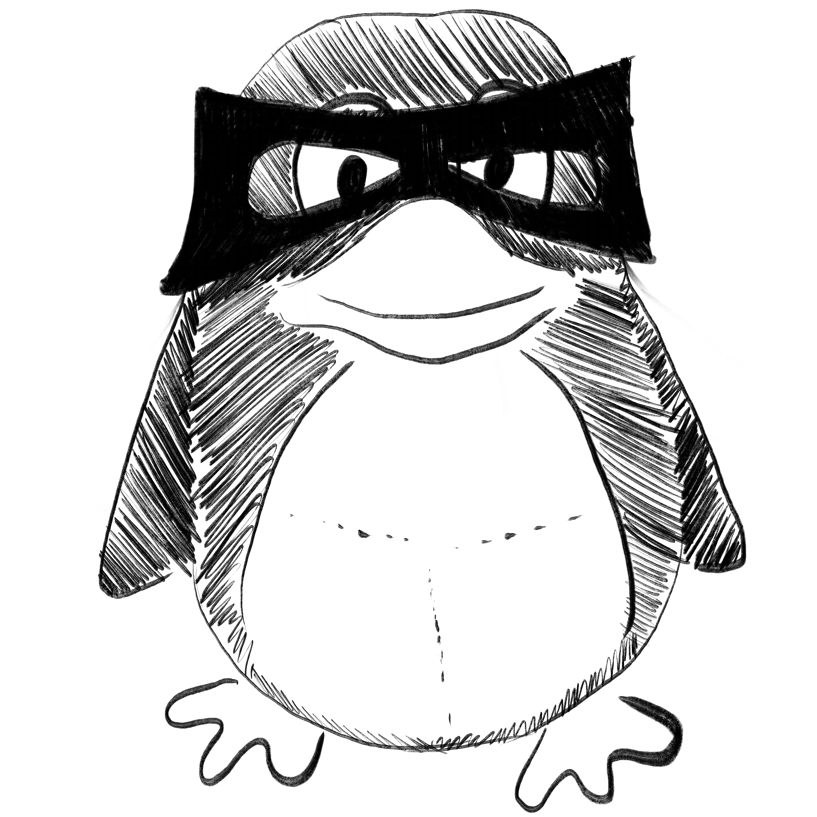
High-fidelity diabetic retina fundus image synthesis from freestyle lesion maps.
In Biomedical optics express
Hou Benjamin
2023-Feb-01
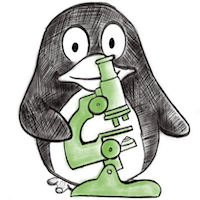
Computationally efficient adaptive decompression for whole slide image processing.
In Biomedical optics express
Li Zheyu, Li Bin, Eliceiri Kevin W, Narayanan Vijaykrishnan
2023-Feb-01
Weekly Summary
Receive a weekly summary and discussion of the top papers of the week by leading researchers in the field.